Exploring Top AutoML Platforms for Businesses
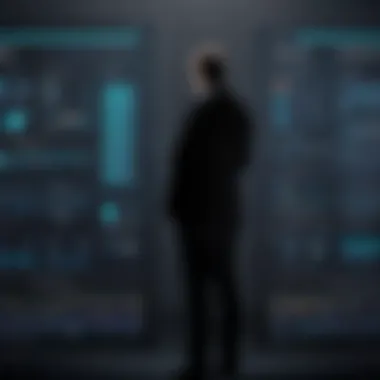
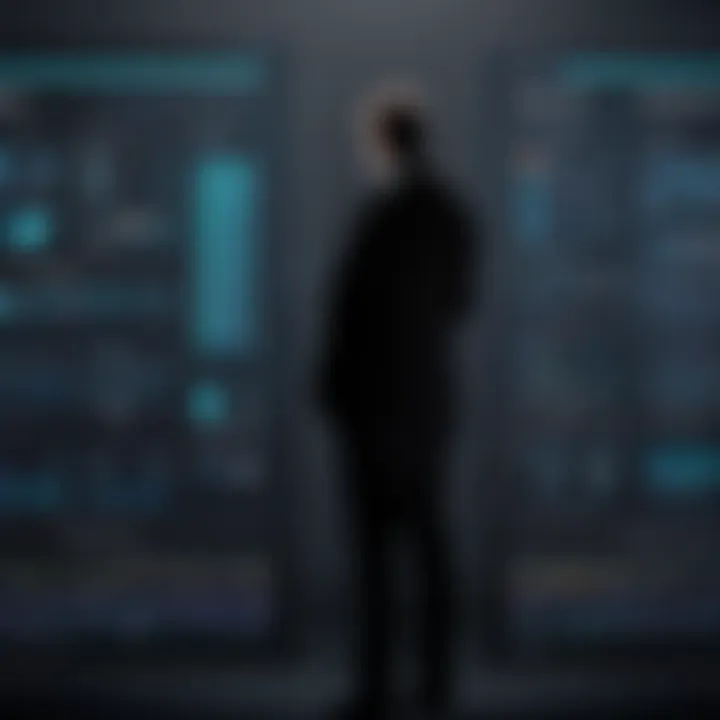
Intro
In recent years, automated machine learning (AutoML) has gained incredible traction among organizations seeking to harness the power of machine learning without needing extensive expertise in the field. The landscape of AutoML platforms has broadened substantially, offering various tools that cater to different business requirements. This article aims to explore these platforms in a comprehensive manner, focusing on their capabilities, unique features, and overall performance.
With companies collecting and analyzing large volumes of data, the ability to process and interpret that data efficiently is more crucial than ever. AutoML bridges the gap between complex algorithms and practical application. Businesses can benefit from these platforms, whether they are large corporations or small startups. The analysis here not only highlights what each platform offers but also delves into the implications of adopting such technology.
By understanding the key features and benefits of leading AutoML solutions, decision-makers will be equipped with the knowledge to make informed choices tailored to their organizational needs. As the industry evolves, staying ahead with capable tools can be a significant competitive advantage. Thus, the upcoming sections will dig into the core aspects of these platforms, paving the way for a strategic selection process.
Prologue to AutoML
AutoML, or automated machine learning, represents a significant advancement in the field of data science and artificial intelligence. As organizations increasingly leverage data for decision-making, the need for efficient and effective machine learning solutions has become paramount. This section serves as a crucial entry point into the exploration of AutoML by establishing a clear understanding of its definition and its importance in contemporary data science practices.
Definition of AutoML
Automated Machine Learning (AutoML) refers to the process of automating the end-to-end process of applying machine learning to real-world problems. This includes tasks such as data preprocessing, model selection, hyperparameter tuning, and model evaluation. The aim of AutoML is to make machine learning accessible to non-experts, enabling users to generate predictive models without requiring extensive background knowledge in the underlying algorithms or programming.
In essence, AutoML tools streamline the complex workflows associated with traditional machine learning practices. This not only saves time but also minimizes human error, enhancing the probability of successful implementation. By offering an interface that simplifies the technicalities, these platforms provide a bridge for businesses to harness machine learning effectively.
Importance of AutoML in Modern Data Science
The importance of AutoML in today's data-driven environment cannot be overstated. Companies across various industries are accumulating vast amounts of data, yet many struggle to derive actionable insights from this information. AutoML addresses several critical challenges in modern data science:
- Increased Efficiency: AutoML platforms significantly reduce the time spent on model development. Data scientists can shift their focus from mundane tasks to strategic analysis and interpretation of results.
- Democratization of Technology: By simplifying access to machine learning, AutoML enables teams without deep technical expertise to participate in data analysis. This growth in accessibility broadens the talent pool and fosters innovation.
- Cost Reduction: Implementing machine learning solutions can be costly. AutoML dramatically lowers the resource requirements for developing models, making advanced analytics available to smaller organizations or those with limited budgets.
- Rapid Prototyping: Businesses can quickly develop and test multiple models to identify the best solutions for their specific needs. This iterative approach enhances decision-making and agility in response to market changes.
- Improved Model Performance: Many AutoML systems incorporate advanced techniques to optimize performance automatically. This helps in achieving better accuracy and reliability in predictive modeling.
"AutoML is not merely a convenience but a transformational approach that empowers organizations to tap into the power of machine learning through automation."
As a result, understanding AutoML is essential not only for data scientists but also for IT professionals and business leaders who aim to integrate data-driven decision-making into their operations. The subsequent sections will delve deeper into the criteria for evaluating AutoML platforms, leading to a comprehensive comparison of the leading solutions available in the market.
Criteria for Evaluating AutoML Platforms
The landscape of AutoML platforms can be confusing due to the vast number of available tools. Evaluating these platforms requires a clear understanding of specific criteria that impact their effectiveness in meeting business needs. This section outlines critical metrics that professionals should consider when selecting an AutoML platform. Understanding these criteria enables organizations to make informed decisions and optimize their machine learning initiatives, ultimately enhancing productivity and outcomes.
Usability and User Experience
Usability is paramount in choosing an AutoML platform. A platform must have an intuitive interface that simplifies the machine learning pipeline for users with varying expertise. Good user experience minimizes the learning curve. Clear documentation and resources are also essential. Tools like Google AutoML offer features tailored for non-technical users, enhancing accessibility.
Key points to consider regarding usability include:
- User Interface Design: A visually appealing and well-organized dashboard improves navigation.
- Guided Workflows: Step-by-step assistance can help users complete their tasks effectively.
- Learning Resources: Availability of tutorials and community support reinforces user confidence.
- Feedback Mechanisms: Systems that allow users to submit issues or seek help enhance the user experience further.
Support for Various Data Types
Flexibility in handling various data types is another crucial evaluation criterion. A robust AutoML platform should accommodate structured, unstructured, and semi-structured data. Support for diverse data formats, including text, images, and time-series data, fosters adaptability. Platforms that excel in this area can address different business needs.
Professionals should evaluate the following aspects:
- Data Ingestion Capabilities: The ease with which data can be uploaded and processed is vital.
- Data Transformation Features: Tools that provide data cleaning and preprocessing functionalities are advantageous.
- Multi-Modal Support: The ability to integrate and analyze different data types together enriches results.
- Scalability for Large Datasets: The platform must efficiently handle big data without compromising performance.
Integration Capabilities
Integration with existing workflows and tools is essential for seamless adoption. An effective AutoML platform should connect easily with databases, cloud services, and other analytics tools. This integration allows organizations to leverage their existing infrastructure and data resources without significant overhaul.
Consider evaluating:
- API Availability: Comprehensive Application Programming Interfaces (APIs) enable smoother connections with various systems.
- Compatibility with Other Tools: The ability to work with popular data storage and processing platforms, such as Amazon Web Services or Azure, enhances usability.
- Support for Workflow Automation: Features that automate data retrieval, model training, and deployment save valuable time.
Performance and Scalability
Performance and scalability are critical components for any organization expecting rapid growth. The selected AutoML platform should not only deliver fast and accurate results but also handle increased workloads as the business scales.
Evaluate the following aspects:
- Model Performance Metrics: Look for features that provide insights into model efficiency and accuracy.
- Resource Management: The ability to adjust resource allocation dynamically based on workload is significant for performance.
- Scalability Options: Support for scaling up or down is essential to meet the organization’s needs.
- Latency and Response Times: Fast model predictions improve user satisfaction and operational efficiency.
Leading AutoML Platforms
In the realm of machine learning, the emergence of AutoML platforms is transforming business practices. These platforms simplify the model development process, making it accessible for users ranging from data science novices to seasoned professionals. The importance of understanding leading AutoML platforms cannot be overstated. They provide organizations with tools that significantly reduce the time and skill required to build effective predictive models. In this section, we will delve into an overview of the platforms, highlight their key features and benefits, and explore various use cases and applications.
Platform Overview
Leading AutoML platforms streamline the process of model building. They automate tasks such as data pre-processing, feature selection, model training, and hyperparameter tuning. By utilizing these platforms, businesses can efficiently leverage their data to derive actionable insights without the extensive investment in human resources typically required for machine learning projects. Notable platforms include Google AutoML, Azure Machine Learning, DataRobot, O.ai, and TPOT, each bringing unique strengths and capabilities to the table.
Key Features and Benefits
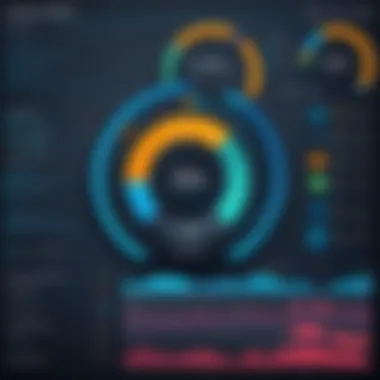
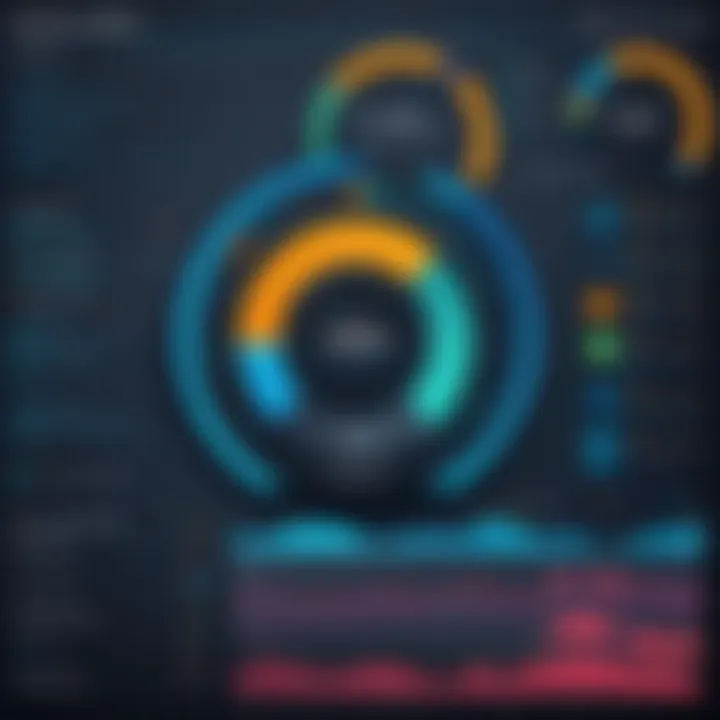
These platforms offer a range of features that make them appealing to users:
- User-friendly Interfaces: Most platforms prioritize ease of use, allowing non-experts to engage with machine learning processes.
- Automation Capabilities: They automate the time-consuming tasks associated with traditional model development, saving significant resources.
- Integration with Existing Systems: Leading AutoML solutions often integrate seamlessly with various data sources, tools, and cloud services.
- Scalability: Many platforms can handle large datasets and complex models, allowing businesses to scale their solutions as their needs grow.
- Reporting and Visualization Tools: These platforms often include dashboards and reporting capabilities to help users interpret model results effectively.
The benefits of using leading AutoML platforms are substantial: they enable faster deployment of machine learning solutions, enhance productivity, and promote innovation. The automation minimizes the margin for human error, allowing organizations to make more informed decisions based on their data.
Use Cases and Applications
The versatility of AutoML platforms allows for a broad array of applications across industries. Some common use cases include:
- Predictive Analytics: Businesses can leverage these platforms to create models for sales forecasting, customer behavior analysis, and risk assessment.
- Healthcare: AutoML is applied to predict patient outcomes and optimize treatment plans based on historical data.
- Finance: Financial institutions use these platforms for fraud detection, credit scoring, and market trading strategies.
- Manufacturing: Companies can employ machine learning models to predict equipment failures and optimize supply chain processes.
- Marketing: AutoML helps in segmenting customer data and personalizing marketing strategies.
"Leading AutoML platforms not only enhance productivity but also empower businesses to harness the true potential of their data."
The insights derived from these platforms contribute to better decision-making and strategic planning, making AutoML a critical resource for today’s organizations. Additionally, as businesses increasingly rely on data-driven approaches, the role of AutoML platforms becomes even more significant in fostering innovation and competitiveness.
Google AutoML
Google AutoML is a prominent player in the automated machine learning landscape. Its significance lies not only in its technological sophistication but also in its accessibility to both seasoned data scientists and those with limited technical skills. This platform enables users to automate the training of high-quality machine learning models. Its integration into the Google Cloud ecosystem is crucial for businesses looking to leverage cloud-based solutions. The importance of Google AutoML in this article is underscored by its comprehensive capabilities that align with varied business needs.
Overview and Capabilities
Google AutoML offers a versatile suite of tools aimed at simplifying machine learning processes. It provides several capabilities, including AutoML Vision for image classification, AutoML Natural Language for text analysis, and AutoML Tables for structured data. Each service is designed to handle specific types of data and tasks, making it adaptable for different use cases. The underlying algorithm uses transfer learning, which allows users to benefit from existing models developed by Google. This feature accelerates the deployment of machine learning solutions while maintaining high accuracy levels.
Benefits to Users
The benefits of using Google AutoML for organizations are multi-faceted:
- Ease of Use: Users without a strong background in machine learning can navigate the platform. Its intuitive interface guides users through the model training process without the need for extensive coding.
- Time Efficiency: Automated processes reduce the time required to develop models. Features like hyperparameter tuning and model selection are performed in the background, allowing teams to focus on higher-level tasks.
- Integration with Google Cloud: Being part of the Google Cloud suite ensures seamless integration with a range of data storage and processing tools available within the cloud infrastructure. This synergy enhances data management and operational efficiency.
"Google AutoML empowers businesses to harness the power of machine learning without requiring deep expertise in the field."
Limitations and Challenges
Despite its strengths, Google AutoML does present several limitations and challenges:
- Cost Implications: While it provides valuable features, costs can accumulate based on usage. Businesses may find expenses challenging to manage, especially at scale.
- Customization Restrictions: Users looking for fine-tuned control over model architecture and tuning might find the platform limiting. This can be an issue for advanced users who need precise adjustments.
- Data Privacy Concerns: For sensitive data, using a cloud service raises inevitable privacy considerations. Organizations must weigh the benefits of cloud solutions against their data security policies.
In summary, Google AutoML represents a powerful tool for organizations eager to implement machine learning. Its focus on usability, efficiency, and integration into the broader Google ecosystem adds to its appeal. However, potential users should carefully consider its limitations to ensure it meets their specific needs.
Azure Machine Learning
Azure Machine Learning is a pivotal player in the autoML landscape. Its extensive features make it a robust choice for organizations aiming to leverage machine learning capabilities without deep expertise. The platform offers various services that help data scientists and developers streamline the workflow of building, training, and deploying machine learning models. Notably, Azure Machine Learning integrates seamlessly with Azure's suite of cloud services, creating a cohesive and powerful ecosystem for both small startups and large corporations.
Functionality and Features
Azure Machine Learning provides a comprehensive set of functionalities designed to cater to diverse user needs. The platform supports both code-first and low-code approaches, allowing users with varying expertise to engage with machine learning. Features like automated machine learning (AutoML) simplify the model selection and tuning process. Additionally, it offers
- Integration with popular programming languages like Python and R
- Support for various data sources, including structured and unstructured data
- Robust deployment options through Azure Kubernetes Service and Azure Functions for model scalability
- MLOps capabilities that facilitate model monitoring and management over time
These features enable teams to efficiently build and deploy models while maximizing performance.
Strengths for Enterprises
Enterprises benefit significantly from Azure Machine Learning's design and integration advantages. One of its key strengths is scalability. Organizations can start with small-scale experiments and easily escalate to production-level applications as required. The platform also offers enhanced security features, crucial for industries handling sensitive data.
Several specific strengths include:
- Enterprise-grade compliance and security, meeting regulatory demands in industries such as finance and healthcare
- Collaboration tools that foster teamwork among data scientists, developers, and business users
- Predictive analytics capabilities that drive insights and data-driven decision-making
- Robust model interpretability options which are essential for businesses to trust their models
These elements combined make Azure Machine Learning an appealing option for businesses looking to integrate machine learning into their operations.
Potential Drawbacks
While Azure Machine Learning has many advantages, it also comes with potential drawbacks. One notable concern is the learning curve for new users who may be overwhelmed by its extensive features and options. Sometimes, its flexibility can lead to complexity, making it challenging for companies with limited technical expertise.
Other drawbacks include:
- Cost considerations that could escalate quickly, especially for heavy usage scenarios
- Dependency on the Azure ecosystem, potentially limiting organizations that use a variety of cloud providers
- Limited community support compared to more open-source options, which can hinder quick troubleshooting and resource sharing
Understanding these drawbacks is crucial for businesses to make informed decisions about adopting Azure Machine Learning.
DataRobot
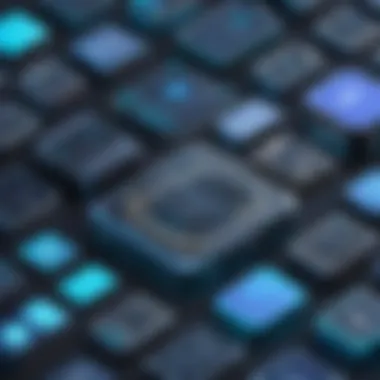
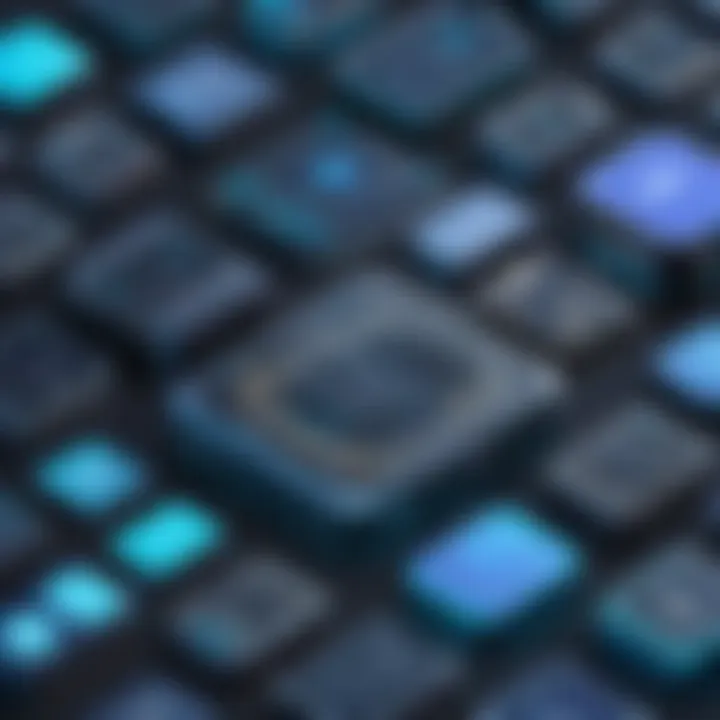
DataRobot stands out as a powerful tool in the AutoML landscape. It provides automated machine learning capabilities that simplify the model building process. For organizations looking to leverage data science without extensive in-house expertise, DataRobot offers essential advantages. This section will explore its features, enterprise benefits, and implementation challenges.
Comprehensive Overview
DataRobot's platform offers end-to-end automation for building and deploying machine learning models. Users can upload their data, specify the target outcome, and let DataRobot perform the heavy lifting. It automatically selects algorithms, engineers features, and performs hyperparameter tuning.
The user interface is designed to be intuitive, allowing non-technical users to engage effectively. DataRobot supports a variety of data formats and integrates well with cloud services and on-premise systems. It offers transparency in model selection, giving insights into why certain models were chosen over others. This clarity is crucial for decision-making and trust in automated results.
Enterprise Benefits and Use Cases
DataRobot is particularly beneficial for enterprises. It enables rapid model development, which is essential in competitive markets. Organizations can gain insights faster, thereby improving decision-making. Here are a few specific benefits:
- Speed: Reduced time from data to deployment can significantly enhance productivity.
- Scalability: Enterprises can scale their machine learning efforts without proportional increases in resources.
- Flexibility: The platform accommodates various use cases, from predictive maintenance in manufacturing to customer churn analysis in retail.
Use cases are diverse. For instance, a large retail chain may utilize DataRobot to analyze customer purchasing patterns and optimize inventory. Similarly, financial institutions can streamline risk assessment processes using historical data.
Challenges in Implementation
Despite its strengths, implementing DataRobot is not without challenges. Some of the factors to consider include:
- Cost: Licensing fees can be a hurdle for smaller organizations.
- Data Quality: The effectiveness of DataRobot depends heavily on the quality of input data. Poor quality or incomplete data may lead to inaccurate models.
- User Resistance: Staff accustomed to traditional methods may resist adopting a new automated approach, hindering integration.
In summary, while DataRobot provides robust capabilities for automated machine learning, careful consideration regarding costs and user buy-in is essential for successful implementation.
O.ai
O.ai stands out in the field of automated machine learning as a powerful tool that caters to a wide range of users, from small startups to large enterprises. Its importance lies in its flexibility and capabilities, allowing users to deploy machine learning models effectively and efficiently. This section will highlight the strengths of O.ai, its key features tailored for data scientists, and some potential shortcomings that users should consider.
Platform Strengths
One of the primary strengths of O.ai is its open-source nature, which provides access to a robust set of features without the constraints of licensing fees. This encourages innovation and community contributions, making it a continually evolving platform.
Furthermore, O.ai is known for its scalability. It can handle large datasets and complex computations, which is crucial for businesses that rely on data-driven decisions. The incorporation of advanced algorithms, including Gradient Boosting Machines and Deep Learning, offers users high-performance modeling capabilities.
- Performance: O.ai shows rapid performance benchmarks in model training and real-time predictions.
- Interoperability: It integrates seamlessly with popular data science languages including R, Python, and Java, enhancing its usability across different user groups.
Key Features for Data Scientists
O.ai includes numerous features that specifically cater to data scientists. One key aspect is its AutoML functionality. This allows users to automate the machine learning process, from data preprocessing to model selection and tuning.
- Model Interpretability: O.ai provides tools for understanding model decisions, which is crucial for transparency in machine learning workflows.
- Visualizations: It has built-in visualizations that help users analyze data and understand model performance intuitively.
- Hyperparameter Tuning: Users can easily adjust and fine-tune parameters, thus optimizing model performance based on specific needs.
Additionally, O.ai supports both supervised and unsupervised learning, making it a versatile tool for a variety of applications, such as fraud detection, customer segmentation, and predictive maintenance.
Potential Shortcomings
Despite its many advantages, O.ai is not without drawbacks. One main issue is the steep learning curve for users who are new to machine learning or data science. While it offers powerful tools, the complexity can be overwhelming for those without a solid background in these areas.
Another limitation is the need for adequate computational resources. While O.ai can scale effectively, deploying it on-demand for large datasets may require significant infrastructure investments,
- Documentation: Although the platform has a wealth of resources, some users find it lacking in more specific examples which could aid in the onboarding process.
- Community Support: While there is an active community, troubleshooting can sometimes be a challenge due to insufficient responses to specific queries.
TPOT
TPOT, an acronym for Tree-based Pipeline Optimization Tool, stands out in the landscape of AutoML platforms for its open-source nature and ability to automate the end-to-end machine learning process. This section explores TPOT's unique features, its advantages as an open-source tool, its engaged user community, and the challenges associated with its accessibility.
Open Source Advantages
The open-source status of TPOT brings several key advantages. First, it offers users the freedom to customize and extend the tool's capabilities. Being open-source enhances transparency; users can examine the underlying code and understand how models are built and altered. This can lead to increased trust in the results generated. Furthermore, it encourages collaboration among developers and data scientists around the world. Users can contribute enhancements, report bugs, or create documentation, benefiting from a culture of co-creation.
In addition, TPOT is built on top of popular libraries such as scikit-learn. This integration allows users to leverage a wide array of pre-existing machine learning models and techniques, thus broadening the functionalities available to them.
User Community and Support
The user community around TPOT is a significant asset. It serves as a space for information exchange and support. Forums like Reddit or specialized chat groups exist where users can share experiences, troubleshoot issues, and discuss best practices. These platforms foster a collaborative learning environment, proving beneficial for both beginners and professionals.
However, compared to proprietary tools, the formal support for TPOT can be limited. Users often rely on community-contributed resources, which can vary in quality and comprehensiveness. The thriving discussions on platforms such as GitHub facilitate a vibrant community where updates and user troubles get addressed, but formal training resources might not be as abundant.
Limitations in Accessibility
While TPOT offers several compelling features, it does come with certain limitations. One of the most significant challenges is its accessibility for non-technical users. Although TPOT has simplified many processes, it still requires familiarity with Python programming and data science principles.
This creates a barrier for business professionals or those without a technical background. Installation can also be complex, as users often need to navigate through various dependencies involved with the software. Additionally, since TPOT primarily runs on local machines, it may not leverage the scalability and processing power of cloud solutions effectively, which is a concern for large-scale datasets.
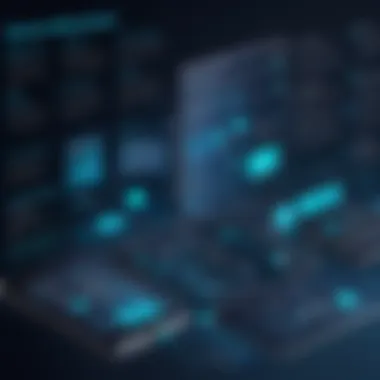
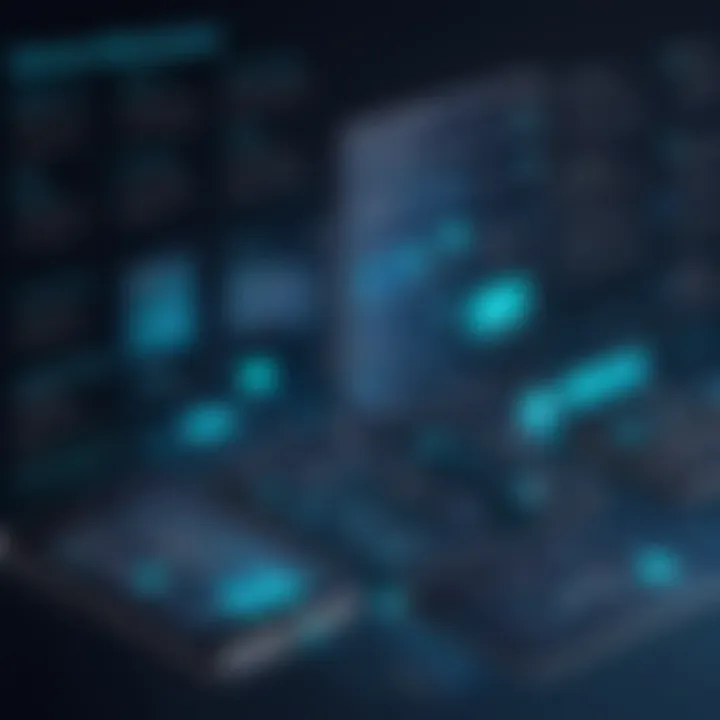
In summary, TPOT is a powerful open-source AutoML tool, but it may not be perfect for every organization due to its accessibility challenges.
Comparative Analysis of Platforms
The comparative analysis of AutoML platforms is a critical aspect of understanding which solutions best meet the needs of various organizations. By examining these platforms side by side, we illuminate their strengths, weaknesses, and overall effectiveness. This analysis is not just academic; it informs business decisions that can significantly affect project outcomes. The key elements to consider during this comparison include performance, ease of use, scalability, and integration capabilities.
A thorough comparative analysis allows businesses to prioritize their requirements and discern which platform aligns with their strategic goals. By evaluating features and operational efficiencies, organizations can avoid potential pitfalls and maximize their investment in automated machine learning.
Strengths and Weaknesses
Each AutoML platform presents unique advantages and disadvantages. Understanding these characteristics is crucial for organizations when making informed decisions.
Strengths:
- User-Friendly Interfaces: Platforms like Google AutoML have intuitive interfaces designed for users regardless of their technical expertise. This accessibility is important for businesses without extensive data science teams.
- Diverse Feature Sets: Platforms often boast a range of features, such as automated data preprocessing, model selection, and hyperparameter tuning, which varies by provider.
Weaknesses:
- Cost Variability: Some platforms, such as DataRobot, can be expensive as they often charge on a subscription basis with costs that scale with usage.
- Limited Customization: For users seeking deep customization, some platforms may feel restrictive, as seen in Azure Machine Learning. Despite its extensive capabilities, it can limit advanced users looking for tailored features.
The balance between a platform’s strengths and weaknesses ultimately determines its fit for specific organizational needs.
Cost Considerations
Cost is a paramount factor in the decision-making process when assessing AutoML platforms. Pricing structures can vary greatly, from subscription-based models to pay-as-you-go.
- Initial Costs vs. Long-Term Value: Some platforms may present low initial costs but become more expensive as usage increases, leading to higher total expenses over time. Solutions like O.ai offer open-source options, potentially reducing upfront investment but may require significant resources for deployment and ongoing maintenance.
- Hidden Fees: Organizations must also be cautious about additional costs associated with data storage, API usage, or other features not initially included in the pricing plans.
Integration Workflows
Effective integration workflows are essential for the successful adoption of any AutoML platform. Organizations should carefully evaluate how each platform interacts with existing data infrastructure and tools.
- Compatibility with Existing Systems: Robust platforms ensure seamless compatibility with popular data sources like SQL databases, Spark, and cloud storage. For instance, Azure Machine Learning excels here by offering integrations that cater to a wide variety of data scenarios.
- APIs and Export Options: The ability to export models and results easily into production environments is vital. Platforms like O.ai and DataRobot provide straightforward APIs that facilitate deployment.
Future Trends in AutoML
The realm of automated machine learning (AutoML) is witnessing rapid advancement, shaping how organizations approach data analytics and modeling. Future trends in AutoML are significant as they represent the evolution of this technology, influencing both the development of new tools and the manner in which data scientists work. Staying abreast of these trends allows businesses to adapt and seize opportunities while remaining competitive in a data-driven environment.
Evolving Capabilities
AutoML platforms are continuously enhancing their capabilities. Recent developments focus on integrating deep learning with meta-learning, enabling platforms to automatically learn from past modeling experiences. A prominent trend is transfer learning, where models trained on one task are effectively used for another, reducing the need for extensive data. This not only saves time but improves accuracy by leveraging existing knowledge.
Moreover, the introduction of explainable AI into AutoML is gaining traction. This approach seeks to provide insights into the decision-making processes of machine learning models. Users can better understand how models arrive at conclusions, which builds trust and aids compliance with regulations like GDPR.
Impact on Data Science Roles
As AutoML systems grow more sophisticated, the roles of data scientists will also shift. Automation will handle routine tasks, allowing data professionals to focus on more strategic and creative functions. Skills in interpreting results and managing model performance will become paramount, while the need for programming may diminish as user-friendly interfaces proliferate.
However, this evolution creates a dual challenge. Data scientists must adapt to new tools while retaining their core analytical skills. Mastery of collaboration with automated systems will become a vital aspect of the role, emphasizing the importance of both technical expertise and interpersonal skills.
Predictions for Market Growth
The future market for AutoML appears promising. Analysts forecast a substantial increase in its adoption across various industries, driven primarily by the need to enhance decision-making processes and operational efficiency. According to recent studies, the AutoML market could potentially reach several billion dollars by 2027.
Factors influencing this growth include:
- Increased demand for data-driven insights among businesses.
- Rising awareness of the benefits of automation in machine learning.
- Expanding capabilities offered by AutoML platforms.
As more businesses recognize the value of AutoML, competition among platforms will likely intensify, leading to improved offerings and better pricing structures. Thus, organizations that remain observant of these trends can leverage this technology to stay ahead in their fields.
As we look ahead, the future of AutoML promises to reshape the landscape of data science.
Overall, the future trends in AutoML are set not only to enhance current functionalities but also to redefine the roles of professionals within the industry, marking a significant shift in data science practices.
Culmination and Recommendations
In the fast-evolving world of data science, selecting an AutoML platform is not just about convenience; it is about choosing a path that aligns with your organization’s strategic goals. With the myriad of platforms available, understanding their unique offerings becomes essential. The concluding section synthesizes key insights from the previous analyses, providing clarity on how to navigate the complexities of AutoML.
An important aspect of this discussion is recognizing that not all AutoML solutions are created alike. Factors such as usability, performance, and integration capabilities significantly influence the effectiveness of these tools in real-world applications. The right AutoML platform can accelerate development cycles, enhance analytical capabilities, and ultimately drive business growth. Conversely, a poor fit might hinder progress and waste resources.
Key Takeaways
- Diverse Options: A range of platforms like Google AutoML, Azure Machine Learning, and DataRobot cater to different needs, from small businesses to large enterprises.
- Evaluate Fit: It is critical to evaluate how a platform aligns with specific project requirements. Considerations like project scale, team expertise, and existing technology stack are key.
- Trial Before Commitment: Utilizing free trials or demos can provide hands-on experience, revealing both strengths and limitations that may not be evident from reviews.
- Future-Proofing Investments: Choose platforms that not only meet current needs but also offer scalability for future projects and evolving data science trends.
"Selecting the right AutoML solution serves to not only enhance efficiency but also to future-proof organizational capabilities in a data-driven marketplace."
Selecting the Right AutoML Solution
When faced with the decision to choose an AutoML platform, various elements come into play. Organizations must first assess their specific needs in terms of data types, project complexities, and output requirements. Here are several key considerations:
- User-Friendliness: Opt for interfaces that promote ease of use. Not all team members will have extensive technical knowledge, so intuitive platforms can play a significant role in adoption.
- Support and Community: Engaging with a vibrant user community and robust support can make a considerable difference. Platforms with active forums or customer service enhance troubleshooting and knowledge sharing.
- Customization and Flexibility: Look for platforms that allow you to customize workflows and model selection, which can adapt to specific project challenges more effectively.
- Cost Efficiency: Evaluate how pricing structures vary among platforms. Some options may charge per usage or offer a subscription model, which can affect long-term viability.