Comprehensive Review of IBM SPSS Statistics Software
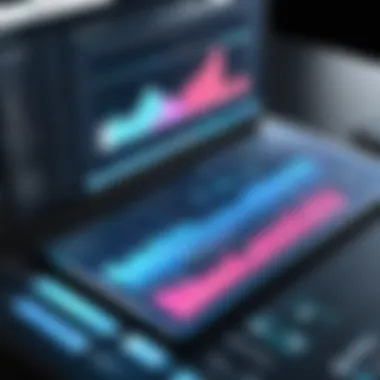
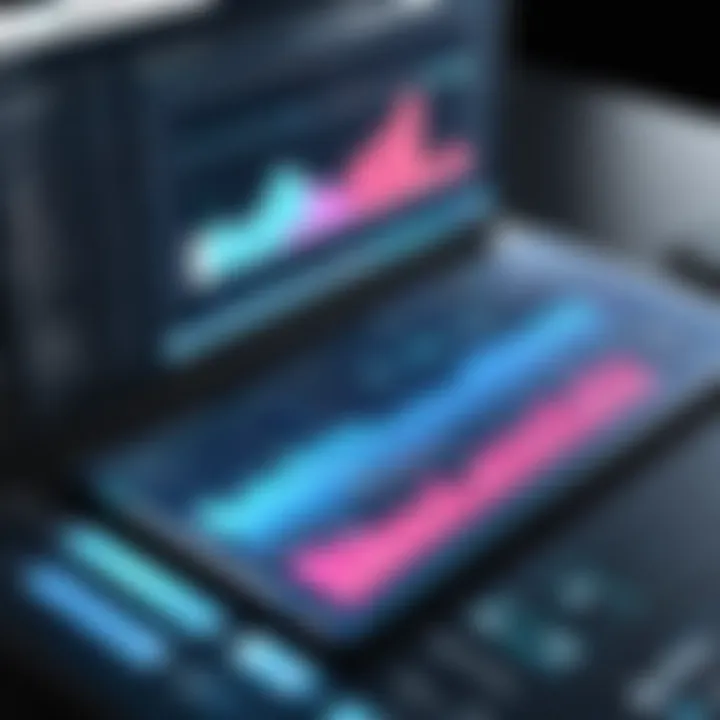
Intro
In today’s data-driven world, making sense of numbers and statistics is crucial for both businesses and researchers alike. Within this realm, IBM SPSS Statistics software stands out as a powerful ally. It’s designed to help users tackle complex data analysis tasks without getting lost in the weeds. This review aims to demystify the capabilities of SPSS, shedding light on its functionalities and why it's a preferred choice for many. From intricate statistical models to user-friendly interfaces, SPSS bridges the gap between raw data and actionable insights.
Key Features and Benefits
Overview of Features
IBM SPSS Statistics comes armed with an impressive array of features that cater to the nuanced needs of various users. Some noteworthy ones include:
- Data Cleaning and Management: Tools that allow users to transform messy data into structured, usable formats.
- Advanced Statistical Techniques: A wide range of methods such as regression, ANOVA, and factor analysis, providing robust options for in-depth analysis.
- Visual Data Presentation: Capabilities for detailed graphing and charting, making it easier to communicate findings through visualization.
- Customization and Extensions: Users can tailor the software using custom scripts and add-ons, enhancing flexibility and functionality.
SPSS also integrates smoothly with programming languages like Python and R, letting advanced users harness the power of coding alongside standard features.
Benefits to Users
The extraction of meaningful insights is paramount, and here’s where SPSS shines:
- User-Friendly Interface: Even if you're not a stats whiz, the intuitive layout makes it accessible to novices while still robust for seasoned professionals.
- Speedy Analysis: The software processes large datasets quickly, allowing for timely decision-making. Businesses can rely on SPSS to keep up with rapid data influxes.
- Industry Versatility: Whether in healthcare, academia, or finance, SPSS’s versatility accommodates various sectors, making it a reliable choice across the board.
"The best software in the world still requires users who know how to analyze effectively"—a reminder that tools are only as good as the users who wield them.
Comparison with Alternatives
While SPSS is a leading player in the statistical software market, understanding how it stacks up against its competitors helps in making informed choices.
Head-to-Head Feature Analysis
When compared to alternatives like R, SAS, or even Excel, SPSS holds its ground in several areas:
- Ease of Use: SPSS is often preferred by businesses without dedicated data science teams, as its learning curve is less steep.
- Comprehensive Support: With an extensive help system and community, users can find solutions and connect with others easily.
- Licensing and Updates: SPSS offers simple licensing for businesses, while updates are generally straightforward without causing significant disruptions.
Pricing Comparison
Pricing structures vary widely across analytical tools. SPSS, while not the cheapest on the market, provides value through its comprehensive features:
- Basic Plans: Suitable for smaller businesses or freelancers often start at a reasonable rate but come with limited functionalities.
- Enterprise Solutions: Larger organizations often invest in subscriptions that provide full features, including collaboration tools, which can significantly enhance productivity.
In closing, deciding on data analysis software is pivotal. With this review, users should have a clearer perspective on how IBM SPSS Statistics can meet their analytical needs while weighing it against competitors.
Prolusion to IBM SPSS Statistics
In today's data-driven world, the ability to analyze and interpret data effectively is more important than ever. IBM SPSS Statistics stands as a cornerstone tool for this task, offering a robust platform for statistical analysis that caters to a broad audience—from seasoned statisticians to novice data analysts. Known for its user-friendly interface and powerful analytical capabilities, IBM SPSS Statistics enables users to draw meaningful insights from their data, making it an invaluable asset for decision-making across a wide array of sectors, including healthcare, business, and academia.
Why does SPSS matter? Well, it’s not just another software; it’s a dynamic resource that allows professionals to unravel complex data sets with relative ease. Users can conduct a variety of analyses, from basic descriptions to intricate inferential statistics, all within an integrated environment. This aspect of versatility is critical for professionals who need to adapt their analyses to diverse datasets and specific research queries.
Moreover, the importance of this software cannot be overstated when we consider the ever-growing demand for data literacy in the workforce. With SPSS, users can bridge the knowledge gap between raw data and actionable insights. Its careful design caters specifically to those lacking extensive statistical training, making it accessible to beginners while still accommodating the advanced requirements of experts.
It's also essential to highlight that SPSS possesses strong data management capabilities. Users can organize, clean, and manipulate their data to fit their analysis needs all within the same tool, saving countless hours of data wrangling.
The software is utilized not just for its computational power but also for its wide-reaching influence in various fields:
- In healthcare, for patient outcomes analysis and epidemiological studies.
- In market research, for customer segmentation and predicting consumer behavior.
- In social sciences, for understanding societal trends and behaviors.
- In education, for analyzing student performance and measuring program efficacy.
As we delve deeper in this article, we will explore the various features, applications, and user experiences that underscore why SPSS continues to be a preferred tool among professionals dealing with data. In doing so, we will present a comprehensive understanding and evaluation of IBM SPSS Statistics, ensuring that prospective users can make informed choices that align with their analytical needs.
Historical Context and Development
Understanding the historical context of IBM SPSS Statistics is crucial to appreciate its growth, significance, and enduring relevance in the world of data analysis. This software did not just pop up overnight; it has roots that tap into the very foundation of statistical computing. Delving into its origins and evolving features provides insights into how it has been shaped by various user needs and technological advancements over time.
Such historical knowledge aids users today by giving them perspective on what to expect from the software, its strengths, and its shortcomings. Ultimately, knowing what has influenced its development over the years enables professionals to harness SPSS's capabilities more effectively in their own projects.
Origins of SPSS
The journey of IBM SPSS Statistics traces back to 1968 when it was created by Norman H. Nie, C. Hadlai Hull, and William G. M. Stein, the founders of the Society for the Psychological Study of Social Issues. They designed the software to simplify the arduous tasks of statistical analysis, chiefly for those in the social sciences. SPSS at its inception was an answer to a call for a more user-friendly and less error-prone way of handling data.
Initially launched for mainframe computers, SPSS captured the intrigue of many. In those days, data analysis often involved tedious manual computations, leaving much room for human error. SPSS provided a straightforward method for performing complex calculations quickly and efficiently. As a pioneering force in the field of statistics, it opened up the world of quantitative data analysis to not just statisticians but also to researchers across diverse disciplines.
Evolution Over the Years
As the years rolled on, SPSS saw a multitude of upgrades that reflected the advancing landscape of technology and user requirements. Through the 1980s and 1990s, the rise of personal computers sparked shifts in software design, making it crucial for SPSS to adapt. The introduction of the Windows-based version in 1992 represented a significant leap forward, allowing more intuitive interaction through a graphical user interface.
By then, SPSS had gathered features that catered to a broader audience, including researchers from the fields of healthcare, marketing, and education. Enhancements such as advanced statistical options, data manipulation capabilities, and automated output processes made it an indispensable tool for data analysts.
The acquisition by IBM in 2009 marked another turning point. With IBM’s robust investment, SPSS became integrated with advanced analytics, machine learning, and data mining technologies, making it even more powerful and versatile. These changes played a pivotal role in ensuring SPSS’s relevance in an age increasingly dominated by big data technology.
The resilience and adaptability of SPSS show that it has not only kept pace with technological changes over the decades but has often been at the forefront of innovation in statistical analysis software.
The narrative of SPSS is a rich tapestry woven with threads of academic ambition, technological advancements, and the demand for efficient data solutions in an ever-evolving world. Understanding this trajectory offers vital context for appreciating its capabilities and applications today.
Core Features of IBM SPSS Statistics
The backbone of IBM SPSS Statistics lies in its core features, which are designed to meet the diverse needs of data analysts and researchers alike. This section will explore these important components, detailing their benefits and providing insights into how they streamline the statistical analysis process.
Statistical Analysis Capabilities
Statistical analysis is the lifeblood of any data-centric endeavor. One of the standout aspects of IBM SPSS Statistics is its extensive array of statistical techniques. Users can perform tasks ranging from simple descriptive statistics to complex inferential analyses with remarkable ease. This versatility allows professionals to draw insights and make data-driven decisions effectively.
The software incorporates a user-friendly interface, facilitating the execution of intricate analyses without the need for advanced programming skills. For instance, a business analyst might utilize SPSS to measure customer satisfaction via survey data by performing t-tests or ANOVA effortlessly. With the built-in options, carrying out multivariate analyses and regression can be as straightforward as a few clicks.
Moreover, the software continuously evolves, including machine learning techniques and predictive analytics that help users not just interpret data but also forecast future trends. This is particularly valuable in today’s fast-paced environments, where timely insights can be the difference between success and failure.
Data Management Tools
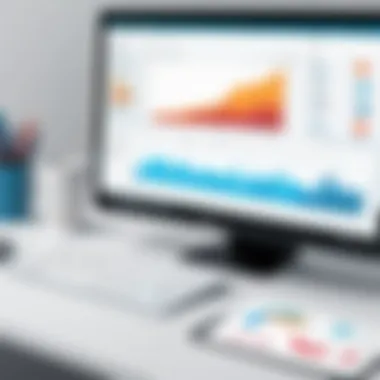
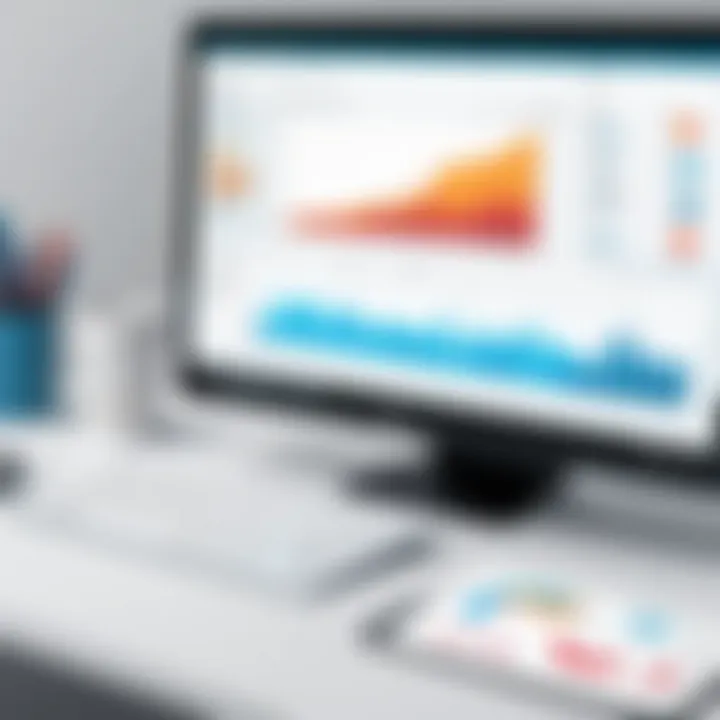
Data management tools within IBM SPSS Statistics serve as essential facilitators, ensuring that users can effectively handle their datasets before diving into analysis. Data preparation can often be a hurdle, but SPSS offers functionalities that minimize such obstacles.
With its data wrangling capabilities, users can easily clean, transform, and manipulate datasets. Whether it’s merging multiple data sources or handling missing values, SPSS provides straightforward processes to ensure that the dataset is in top shape for analysis. For example, a researcher working on a healthcare project can easily adjust datasets with patient demographics and clinical outcomes, turning raw numbers into informative insights that could lead to improved care practices.
The software also allows for automated data management tasks, which can save hours of manual labor, enabling analysts to focus more on interpreting results rather than fiddling with formats. The ability to handle large data sets makes SPSS suitable for both small-scale projects and those that require big data capabilities, thus catering to a broad audience.
Graphical User Interface
The Graphical User Interface, or GUI, of IBM SPSS Statistics exhibits a thoughtful design that caters to both novices and seasoned analysts. For users who are less technically inclined, the visual layout offers an intuitive experience that allows them to navigate through functions without feeling overwhelmed. This is achieved through clearly labeled menus, drag-and-drop options, and guiding dialogues that simplify the process.
With tools for data visualization at hand, users can create meaningful graphs and charts to represent complex data visually. Being able to showcase findings through these visuals greatly enhances the clarity of the data story being told. An analyst presenting demographic trends, for instance, can quickly generate histograms or scatterplots to highlight key statistics, effectively communicating insights to stakeholders who might not be familiar with statistical jargon.
Furthermore, the possibility to customize visual outputs provides flexibility, allowing users to tailor presentations according to their audience's preferences. This adaptability ensures that findings are conveyed in the most impactful manner, regardless of the setting—be it a board meeting or an academic conference.
"The visualization capabilities of SPSS help bridge the gap between data analysis and practical application, enabling users to tell their data's story effectively."
Statistical Techniques Offered
When diving into the realm of IBM SPSS Statistics, understanding the statistical techniques it offers is crucial. These techniques form the backbone of data analysis, allowing users to derive insights from complex datasets. With robust methods at their disposal, users can confidently anchor their findings, making informed decisions in a vast variety of fields.
Descriptive Statistics
Descriptive statistics serves as the bedrock of any data analysis endeavor. This method provides a clear picture of the data's basic characteristics without delving into any inferences or predictions. It offers users various tools to summarize data through measures like mean, median, mode, and standard deviation. Moreover, the ability to easily visualize data distributions through histograms and box plots enhances comprehension.
SPSS simplifies these calculations with user-friendly dialogue boxes, making it accessible even for those new to statistics. Users can quickly generate summaries that not only uncover trends but also raise questions worth exploring further. This initial overview is critical; it often sets the stage for deeper analytical pursuits.
Inferential Statistics
Inferential statistics takes the analysis a step further by allowing users to draw conclusions beyond the immediate dataset and make predictions about broader populations. This is where hypothesis testing comes into play. Using techniques like t-tests, ANOVA, and chi-square tests, users can evaluate their ideas against actual data, determining the significance of observed patterns.
In the context of SPSS, these tools are packed into neat modules which streamline the process—making it less daunting. Properly wielded, inferential statistics can uncover correlations, guide marketing decisions, or indicate the effectiveness of a new drug in healthcare settings. However, caution is warranted; misconceptions arising from misinterpreted data can lead to flawed conclusions.
Regression Analysis
Regression analysis is another powerful tool in the SPSS arsenal, aimed at examining relationships between variables. It not only helps in forecasts but also sheds light on which variables influence others. With linear regression, users explore straightforward relationships, while logistic regression takes a step into the world of binary outcomes.
When targeting a business audience, imagine a retailer analyzing sales against marketing spend. Using SPSS's regression tools, they can quantify the impact of each marketing tactic, helping to optimize future strategies. Yet, one must be circumspect; correlations do not imply causation. Recognizing this nuance is vital to valid analyses.
Multivariate Analysis
In an increasingly interconnected world of data, multivariate analysis has become indispensable. This technique allows researchers to study multiple variables simultaneously, unveiling complex relationships and patterns that single-variable analysis might overlook. SPSS facilitates multivariate techniques such as MANOVA and factor analysis, enabling users to dissect layers of data efficiently.
For instance, in social science research, understanding how a range of factors such as income, education, and age impact lifestyle choices can be critical. Using SPSS, researchers can visualize these relationships dynamically. However, performing multivariate analyses requires a solid understanding of the data, as misinterpretation could lead to incorrect inferences.
"In the world of data, knowing the right techniques and using them effectively is akin to wielding a sharp tool in the hands of a craftsman; precision makes all the difference."
Applications of IBM SPSS Statistics in Various Fields
IBM SPSS Statistics software finds its footing in a myriad of sectors, utilizing its powerful analytics capabilities to inform decisions and strategies. Understanding the applications in various fields can illuminate how businesses and researchers leverage the software’s tools to extract meaningful insights from data. This section will delve into specific areas—including healthcare, market research, social sciences, and education—highlighting the software’s significance and versatility.
Healthcare Analytics
In the realm of healthcare, data plays a pivotal role in enhancing outcomes, controlling costs, and improving overall service efficiency. IBM SPSS Statistics enables healthcare professionals to analyze patient data, treatment outcomes, and resource utilization effectively. It allows for the exploration of patterns and predictions regarding patient care, improving the quality of medical services.
Key benefits in healthcare analytics include:
- Improved Patient Outcomes: Analyzing treatment efficacy through statistical methods helps in refining patient care strategies.
- Cost Control: Identifying inefficiencies in resource allocation helps organizations manage budgets more effectively.
- Predictive Analytics: By predicting patient admission rates, hospitals can optimize staffing and resource distribution.
"Data is the new oil. It’s valuable, but if unrefined it cannot really be used."
With the ability to generate comprehensive reports, SPSS helps healthcare providers make data-driven decisions to improve their services and ensure patient satisfaction.
Market Research
For businesses, market research is a necessity, providing insight into consumer behavior and market trends. IBM SPSS Statistics equips marketers with tools to analyze survey data, conduct segmentation analysis, and gauge brand perception in the market.
Some noteworthy applications within market research are:
- Consumer Insights: Understanding customer preferences and behaviors aids in tailoring marketing strategies.
- Segmentation: Identifying distinct groups within a market allows for focused marketing efforts.
- Trend Analysis: Monitoring changes within consumer habits over time leads to more informed product development.
Marketers using SPSS can decode complex datasets, gaining clarity in what drives consumer decisions and how to effectively respond to market dynamics.
Social Science Research
In social sciences, the role of data analysis is fundamental to understanding human behavior and societal trends. IBM SPSS Statistics serves as a robust tool for researchers in sociology, psychology, and economics, enabling them to conduct comprehensive analyses of survey data and experimental results.
The applications in social science research include:
- Survey Analysis: SPSS helps in the analysis of broad datasets gathered from surveys, revealing insights into public opinions and social phenomena.
- Correlation Studies: Researchers can explore relationships between social variables, aiding the understanding of complex interactions.
- Longitudinal Studies: With its ability to manage and analyze data over time, SPSS supports tracking behavioral changes and social trends.
Through its powerful statistical techniques, the software assists in developing theories and testing hypotheses, providing an evidence-based understanding of societal issues.
Education and Academic Research
Across educational institutions, SPSS is utilized for academic research, allowing educators and students to analyze performance data, assess educational programs, and conduct psychological assessments. The flexibility of SPSS makes it accessible for both learners and experienced analysts within academia.
Highlighted applications in this field include:
- Performance Evaluation: Institutions analyze student performance data to assess the efficacy of teaching methods.
- Program Assessment: Evaluating the impact of academic programs on student outcomes helps in curriculum development.
- Educational Research: Facilitating research on pedagogical methods enhances the quality of education.
The user-friendly interface of SPSS allows educators to engage in rigorous data analysis, fostering a culture of evidence-based practices in educational settings.
Customization and Extensibility
Customization and extensibility are vital components of IBM SPSS Statistics, making the software not just a one-size-fits-all solution but a highly adaptable tool for various statistical needs. As the landscape of data analysis evolves, the ability to tailor software to specific requirements becomes increasingly important. Organizations often have unique datasets or particular analytical needs that off-the-shelf solutions can't adequately address. Having the ability to adjust settings, create custom functions, or integrate additional tools is what keeps SPSS relevant.
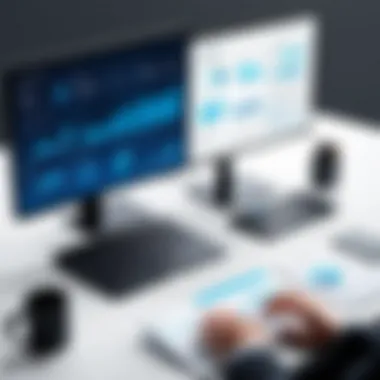
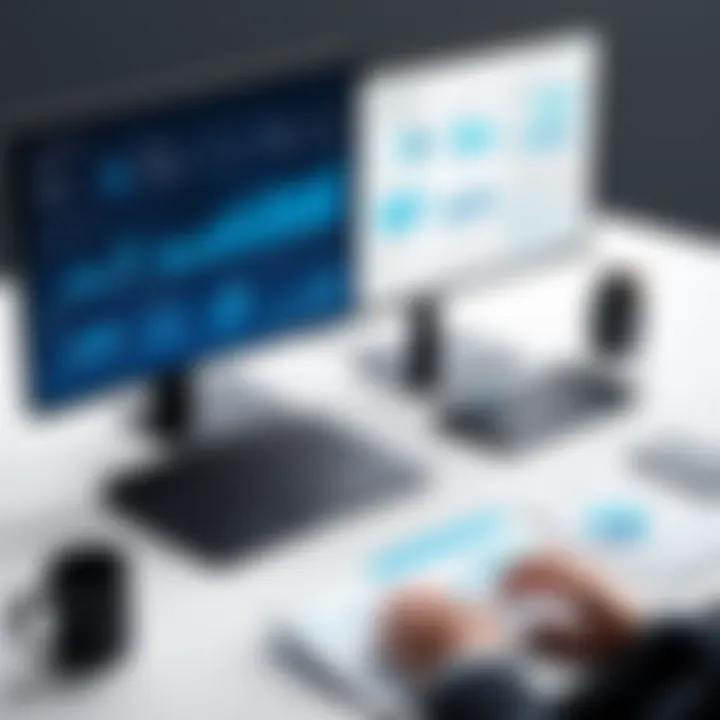
One of the significant benefits of customization is that it allows users to enhance their analytical capabilities. For instance, users can define their own statistical routines through user-defined functions. This can be especially useful in specialized fields where standard statistical methods might not suffice. Moreover, customized tools can improve workflow efficiency, enabling users to concentrate on their analysis rather than navigating through unnecessary features.
Consideration around extensibility also comes into play when individuals or businesses require integration with other software systems. SPSS is compatible with a variety of programs, making it easier to import and export data across different platforms. Such interoperability not only streamlines the data analysis workflow but also enriches the functionality of the software as a whole, allowing users to capitalize on the strengths of multiple tools.
Customization and extensibility offer a tailored user experience that can significantly impact the outcomes of data analysis projects. In a constantly shifting data environment, the flexibility of SPSS allows its users to stay agile and responsive to their unique challenges.
User-Defined Functions
User-defined functions (UDFs) are a cornerstone of customization in IBM SPSS Statistics. These functions allow users to tailor statistical computations according to their specific needs. By enabling users to define their own routines, UDFs act like a Swiss Army knife—they come in handy for countless situations. For instance, if a user frequently needs to calculate a unique form of standard deviation that isn't readily available, they can create a UDF that performs this task, saving valuable time and effort.
Additionally, having the ability to develop UDFs means that statistical analyses can become more sophisticated. Researchers in specialized fields can implement niche algorithms or methodologies that enhance their research without waiting for software updates or releases that may not address their needs.
Using UDFs not only increases efficiency but also allows for the documenting of specific analytical methods, which is especially useful in fields like social sciences where reproducibility and transparency are paramount. Here's a quick example for defining a basic UDF:
In the example, a user can define a standard deviation function, which they can call upon at any point, thus enhancing their analytic repertoire without cumbersome re-calculations.
Integration with Other Software
The ability to integrate IBM SPSS Statistics with other software is another major facet of its customization and extensibility. In today’s data-rich world, relying solely on one program can be limiting. Users often need to pull data from various sources and subsequently push results to different platforms for reporting or sharing purposes. Integration plays an essential role in creating seamless workflows.
For instance, SPSS can connect with databases like Oracle or SQL Server, allowing users to manage their data more effectively. Queries can be run directly from within SPSS, eliminating the need to switch between applications. This not only optimizes productivity but also reduces the chances of errors introduced during data transfers. Furthermore, integration with tools such as Microsoft Excel allows SPSS users to leverage familiar interfaces to conduct complex statistical analysis. Those with advanced analytic needs can also connect SPSS with programming environments like Python or R, significantly extending the range of available statistical techniques.
"Flexibility in software can lead to significant advancements in data analysis workflows, bringing efficiency and precision that manual processes simply cannot match."
Integrating SPSS with other platforms and services ultimately leads not just to a richer user experience, but also to better, more insightful data analysis outcomes. Whether it's pulling in real-time data from an online source or pushing results in a polished format to stakeholders, the software's extensibility is essential in meeting modern demands in analytical contexts.
User Experience and Learning Curve
Understanding the user experience and learning curve of IBM SPSS Statistics is essential for both novice users and seasoned data analysts. This aspect focuses on how effectively individuals can adapt to the software, addressing the accessibility and complexity of its features. A smooth user experience can significantly enhance productivity, as users can spend more time analyzing data rather than wrestling with software intricacies.
The balance between functionality and usability is vital. Users expect a platform that is not only powerful but also intuitive enough to allow for quick navigation and effective use of its tools. When considering the learning curve, it’s noteworthy that while SPSS is designed for serious data analysis, it manages to cater to various skill levels—from the inexperienced to the highly experienced. Below, we delve into the accessibility for beginners and the advanced features available for experts, providing insights on how these elements influence user experience.
Accessibility for Beginners
For newcomers to the world of statistical analysis, the accessibility of IBM SPSS Statistics plays a critical role in determining whether they will successfully integrate the software into their workflow. The program is equipped with various built-in tutorials and guides. These resources are often seen as light at the end of the tunnel, guiding users through basic functionalities and gradually introducing more advanced concepts.
A notable feature is the point-and-click interface, which allows users to perform statistical analyses without needing extensive programming knowledge. Users familiar with tools like Excel can find themselves more at ease, as the transition to SPSS is considerably smooth. There is also the menu system, which organizes functions logically, making it easier to locate specific features needed at different stages of statistical work.
Moreover, forums and community support play a huge role in the learning journey. Users can turn to platforms such as Reddit, where discussions about typical beginner hurdles often provide practical solutions. Given that learning any complex software can feel like learning a new language, having a community where users share tips, tricks, and experiences is invaluable.
Advanced Features for Experts
As users become more proficient, the true power of IBM SPSS Statistics is revealed. The advanced features tailored for experts can significantly enhance the complexity and depth of analysis. For seasoned data scientists or analysts, the ability to create custom functions and leverage syntax commands can transform SPSS from merely a data analysis tool into a versatile analytical powerhouse.
Options like scripting and automation of repetitive tasks can save tons of time. Syntax not only streamlines analyses but it also enhances reproducibility—a crucial factor in many research environments. Additionally, by using the IBM SPSS Modeler, advanced users can integrate even more complex analytical capabilities and predictive modeling fluently into their workflow.
Furthermore, experts have the opportunity to delve into custom plugin development, enabling them to extend the software’s functionalities beyond its out-of-the-box capabilities.
"SPSS is not just a tool; it becomes an extension of your analytical thoughts and processes, allowing for an exceptional depth in data exploration when mastered."
Performance Metrics
Evaluating performance metrics in IBM SPSS Statistics is critical for understanding not just how the software operates, but also how it meets the necessary demands of users. For professionals and businesses, performance metrics represent a way to measure the effectiveness and efficiency of the software. They hold the key to knowing whether SPSS can handle the volume and complexity of data in specific scenarios, ultimately influencing decision-making processes.
Performance metrics can break down into a few focal points, namely speed and efficiency and software stability and reliability. Each aspect combs through not just how the software functions in ideal conditions, but how it copes under pressure, signifying the robustness of SPSS itself.
Speed and Efficiency
When it comes to speed, one must recognize that data analysis is often a race against time. In sectors where quick decisions translate into competitive advantages, the speed at which SPSS processes data becomes indispensable. For instance, users juggling vast datasets can expect many operations—like running complex statistical tests—to be finished within a tight timeframe.
Efficiency, however, goes hand-in-hand with speed. It's not simply about how fast SPSS can churn out results, but rather how resourcefully it utilizes the available computing resources. Generally, the software optimizes memory usage and manages CPU performance efficiently, which is crucial when dealing with large data volumes. In stark contrast, some statistical software may finish an analysis faster but require an exorbitant amount of system resources, leading to sluggishness elsewhere.
Software Stability and Reliability
A cornerstone of any analytical tool, stability, and reliability are essential attributes that SPSS boasts. Users need assurance that the software will handle tasks without crashing or producing erroneous results. After all, in the world of data analysis, credibility hinges on reliability. Units of work - sometimes the product of multiple hours of intricate data manipulation - depend upon the software maintaining its integrity.
In real-world applications, instances may arise where users have pushed the limits of SPSS, performing intensive analyses without encountering glitches. This speaks volumes to the software's robustness and the confidence users often feel whilst conducting analysis.
"For any serious data analyst, there’s little room for error. SPSS delivers when it matters most, giving certainty amidst complex datasets."
In summation, performance metrics are crucial as they not only tell the performance story of SPSS but also influence user perceptions and choices. As data grows in both size and intricacy, the importance of evaluating these metrics will only amplify. Hence, prospective users must prioritize which performance metrics matter most for their specific application needs in order to fully leverage SPSS's capabilities.
Cost and Licensing Model
Understanding the cost and licensing model of IBM SPSS Statistics is crucial for organizations that are considering adopting this powerful statistical tool. The financial implications of obtaining a software license can significantly impact decision-making processes, especially for businesses with tight budgets. Evaluating the various licensing options available helps potential users align their choices with their particular needs and objectives. Alongside that, analyzing the overall value for investment gives buyers a clearer picture of what SPSS offers beyond its initial price tag.
Different Licensing Options Available
IBM offers multiple licensing options for SPSS Statistics, which cater to different types of users—from students to large corporations. The main options include:
- Subscription License: This model allows users to pay on a monthly or annual basis, providing flexibility. Companies can easily scale up or down as necessary.
- Perpetual License: Users pay a one-time fee for unlimited use of the software. This might be beneficial for organizations looking at long-term commitments. However, ongoing support and updates require additional yearly fees.
- Student License: Aimed at students, this option is offered at a significantly reduced price, enabling academic users to access the software without breaking the bank.
- Site License: Organizations can purchase a single license that covers multiple users, which is ideal for universities or businesses where many employees need access.
Each type of license has its pros and cons, making it essential for potential buyers to assess their specific scenario before deciding.
Value for Investment
When it comes to IBM SPSS Statistics, the value goes beyond the monetary cost. It encapsulates the benefits derived from using the software over time. Here are some critical aspects to consider:
- Functionality: The breadth of statistical techniques offered is substantial. Users can execute complex data analyses, which can streamline vital decision-making processes.
- Support and Training: With a purchase, companies often gain access to resources for training, which can minimize the learning curve and enhance productivity.
- Data Management: Effective data handling capabilities mean that users can organize, analyze, and visualize data all within one platform, reducing the need for multiple tools.
- Evolving with Technology: IBM regularly updates SPSS Statistics, ensuring it stays relevant amidst changing technological landscapes.
Investing in SPSS is not merely about acquiring software; it's about gaining a suite of tools that can yield data-driven insights—turning raw data into actionable information. For any organization committed to making informed decisions, exploring SPSS may well be worth the cost.
"The cost of an opportunity is an investment in the future, not just an expenditure of today."
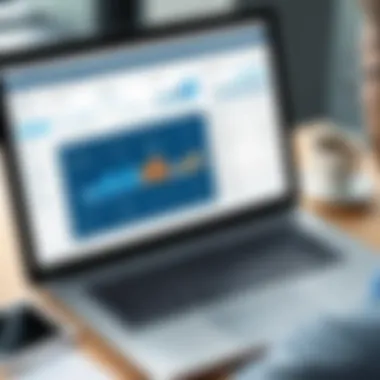
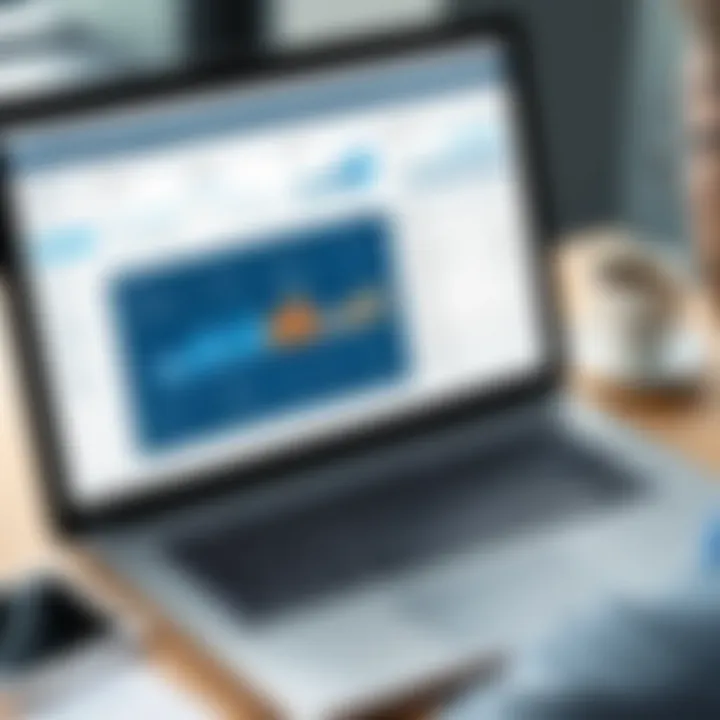
Whether your organization is a budding startup or a well-established enterprise, the ability to leverage powerful statistical solutions can significantly enhance strategic choices and ultimately drive success.
Comparison with Competing Software
In any field that involves data analysis, software tools are the lifeline for translating information into actionable insights. Comparing IBM SPSS Statistics with its competitors helps to understand where it stands in terms of features, usability, and value. Each software solution offers its own unique capabilities, thus making it crucial for users to grasp specific attributes and suitable scenarios where one may outperform the others. By analyzing competing software, users can find the best fit for their needs, whether it be for intricate academic research, business analytics, or other applications.
R Statistics
R Statistics is a heavyweight in the world of data analysis, recognized for its robustness and extensive package ecosystem. The sheer versatility R provides is a strong draw for many statisticians and data scientists. Here are a few key points regarding R:
- Free and Open Source: R is freely available, which makes it an attractive option for budget-conscious users.
- Rich Package Library: With numerous packages like for visualizations and for data manipulation, R stands out as a tool for complex analyses.
- Deeper Programming Knowledge Required: Using R effectively often necessitates a certain level of programming proficiency, which could be a barrier for non-technical users.
R shines in academia and specialized fields but may be less friendly for those who prefer a graphical interface like the one SPSS offers. As such, the decision between R and SPSS largely hinges on the user’s background and their specific requirements.
SAS Software
SAS is renowned for its enterprise-level capabilities and deep integration into industry practices. It is often utilized in large corporations for its proven statistical strength. Here’s how SAS compares with IBM SPSS Statistics:
- Data Handling at Scale: SAS excels in managing massive datasets, which is essential in industries like finance where data volume and complexity are high.
- Proprietary with High Learning Curve: Though powerful, SAS can be quite expensive, and it usually requires training to fully leverage its advanced features.
- Comprehensive Support and Community: SAS provides structured and extensive support that many organizations rely on, making it a solid choice for those needing reliability.
For firms or institutions that handle voluminous data daily and have the budget for training and licensing, SAS might be more advantageous compared to SPSS. But SPSS can often deliver the required functionality with a lower cost of entry and steeper learning curve.
Excel for Statistical Analysis
When it comes to ease of access, Microsoft Excel is a household name and frequently serves as the first introduction to data manipulation and statistics:
- Familiarity and Accessibility: Most users are accustomed to Excel’s interface, shortening the learning period significantly.
- Basic Analysis Tools Available: Excel has various built-in functions catering to basic statistical analysis, suitable for preliminary data investigations.
- Limited Advanced Features: Though great for simple analyses, Excel lacks specialized statistical tests and capabilities found in SPSS, like advanced regression techniques, which leads many users to transition to SPSS for more complex needs.
Ultimately, while Excel functions as a reliable stepping stone toward deeper statistical analysis, its limitations are apparent as more intricate statistical needs arise, thus paving the way for exploring comprehensive solutions like SPSS.
The choice of statistical software is often influenced by user expertise, analysis needs, and budget considerations. It's wise to weigh the unique strengths and weaknesses of each tool before committing.
Future Developments and Trends
The landscape of data analysis is rapidly evolving, and with it, the tools we use for such analyses. Recognizing the importance of future developments and trends in IBM SPSS Statistics software not only informs users about the potential capabilities they can expect but also prepares them for the dynamic shifts in data analysis paradigms. As we tread deeper into an era dictated by technology and data-driven decisions, exploring these advancements can underscore the relevance and potential growth of SPSS as a go-to statistical software.
Emerging Technologies in Data Analysis
When we talk about emerging technologies in data analysis, we are diving into a realm filled with possibilities that augment the capabilities of traditional software like SPSS. Key advancements include:
- Artificial Intelligence and Machine Learning: The integration of AI and machine learning algorithms within SPSS can dramatically enhance predictive analytics. For instance, instead of just performing standard regression analyses, users may leverage sophisticated algorithms that adapt and learn from data patterns, offering deeper predictions and insights.
- Natural Language Processing: The infusion of NLP can facilitate more intuitive data interactions. Imagine querying data sets simply by typing a question in plain English instead of complicated syntax. This would democratize data access, opening up opportunities for non-technical users to engage with analysis seamlessly.
- Cloud Computing: The shift towards cloud-based solutions is notable. This transition allows users to access SPSS from anywhere while benefiting from collaborative functionalities that enhance team engagement and efficiency. With cloud integration, large data sets can be hosted with greater speed and flexibility, allowing for more extensive analyses without the perennial bottleneck of local hardware.
These technologies represent a significant shift in how organizations approach data. IT professionals and software users must remain vigilant and ready to adopt tools that leverage these innovations. Their ability to adapt might just set them apart in an increasingly competitive marketplace.
Potential Enhancements to SPSS
As trends in the industry burgeon, SPSS cannot remain static. Here are a few potential enhancements that could redefine SPSS's position in the data analysis ecosystem:
- User Interface Overhaul: A fresh redesign of SPSS’s user interface could significantly enhance usability. By simplifying navigation and data visualization capabilities, the overall user experience would improve, making the powerful analytics tools more accessible.
- Improved Data Visualization Tools: Enhanced visualization options can provide users with more interactive and engaging ways to present their findings. For example, incorporating advanced charting options or real-time dashboards would allow users to communicate data trends more effectively.
- Integration with Emerging Databases: The rise of NoSQL and other non-traditional databases necessitates SPSS's adaptation. By providing seamless integrations with such systems, users could analyze a wider array of data types contributing to a more holistic analytical process.
- Increased Customization Options: As more unique analytical needs arise, the demand for user-defined functionalities will only grow. SPSS may benefit from allowing greater flexibility in crafting tailored scripts and functions, enabling users to meet precise analytical demands.
These enhancements are not just speculative—they are imperative for SPSS to maintain its relevance in a competitive environment. Market research professionals and business analysts stand to gain tremendously from a tool that evolves in tandem with industry requirements.
Overall, both the emergence of new technologies and the potential enhancements to SPSS reinforce a crucial message: the ability to adapt to change is paramount in the world of data analysis. Those who stay attuned to these shifts will be better equipped to leverage data effectively, ultimately driving informed decision-making across multiple sectors.
"In the ever-evolving world of data, adaptability isn't just an advantage; it's a necessity."
By embracing these trends and possibilities, IBM SPSS Statistics can continue to be a pioneer in statistical software solutions.
User Reviews and Feedback
When it comes to software, user reviews are like gold. They offer insights into what actual users experience, shedding light on both the strengths and drawbacks of the product in real-world usage. In the context of IBM SPSS Statistics, understanding user feedback is particularly valuable for professionals considering investing their time and resources into this software. The clout that user opinions hold can steer potential users toward or away from making long-term commitments. This section captures the crux of laymen’s experiences, showcasing positive insights and confronting challenges that SPSS users typically face.
Positive User Insights
Many users of IBM SPSS Statistics have found the software to be a powerhouse for analysis. Users frequently commend its robustness in statistical processing and how the software caters to a wide array of analytical needs. The intuitive layout of its Graphical User Interface (GUI) is often highlighted, making it more approachable for newcomers who might find statistical software daunting at first.
In particular, users often appreciate the following aspects:
- Comprehensive Tools: The availability of diverse statistical methods ranging from basic descriptive statistics to advanced regression techniques enables deep data analysis without switching between tools.
- Seamless Integration: SPSS integrates smoothly with spreadsheets and other software, enabling easy data import and manipulation, which many find a game-changer.
- Extensive Documentation: Users note that the documentation and resources available online are quite thorough. The ability to find solutions or learn how to use new features enables users to expand their analytical skills rapidly.
- Supportive Community: On platforms like Reddit and various forums, users often discuss techniques and solutions, fostering a community that helps each other navigate complex statistical issues.
“With SPSS, I feel like I have a statistical powerhouse at my fingertips, and the user interface makes it easy to drill down into the data.”
– A satisfied IBM SPSS user
Challenges and Limitations
Despite the many positives, it's essential to recognize that no software is perfect. Some users have voiced concerns regarding IBM SPSS Statistics, revealing challenges that prospective buyers should consider. It's crucial for businesses, whether small or large, to weigh these limitations seriously before adopting the tool. Commonly mentioned challenges include:
- Cost Implications: Pricing is often highlighted as a significant barrier, especially for smaller businesses or academic institutions that may struggle to allocate budget for licensing fees.
- Steep Learning Curve for Advanced Features: While the software is accessible to beginners, the more advanced functionalities can present a struggle. Some users have felt overwhelmed when trying to utilize more complex analyses without prior experience or training.
- Performance with Large Data Sets: Certain users report that SPSS can slow down or even crash when dealing with extensive datasets, making it less reliable in high-stakes scenarios where data integrity is critical.
- Limited Flexibility for Customization: More experienced analysts occasionally find SPSS weniger adjustable than open-source alternatives, limiting the software's efficacy in highly specialized analyses.
These points of contention provide a well-rounded perspective of the user base. While IBM SPSS Statistics holds many merits, being aware of its limitations is certainly a prudent approach for any potential user.
Final Thoughts on IBM SPSS Statistics
In reflecting on the significance of IBM SPSS Statistics, it's essential to grasp not just its robust capabilities, but also its standing as a go-to tool for data analysis across various industries. The intersection of usability, depth of statistical techniques, and customizability makes this software a mainstay for many professionals.
Summary of Capabilities
IBM SPSS Statistics offers a cornucopia of features that cater to a range of analytical needs. Users are particularly drawn to its:
- Statistical Analysis: There’s a rich suite of methods, from basic descriptive stats to advanced multivariate techniques. It allows users to perform detailed analyses with relative ease.
- Data Management: This software streamlines the data handling process. It offers tools to manipulate datasets and even integrates well with databases. This feature is like having a Swiss Army knife for data.
- User Interface: Many appreciate the graphical user interface, which makes the software quite approachable, especially for novices. It enables different analytical tasks to be executed visually, thus lowering the barrier to entry.
- Customization Options: Last but not least, SPSS supports extensive customization. Whether it be creating user-defined functions or integrating with other platforms, users have the flexibility to tailor their environments to fit specific project needs.
In a nutshell, these capabilities solidify SPSS as a mainstay in data analysis, providing tools that facilitate both simple and advanced tasks, allowing users to extract meaningful insights from datasets.
Ideal User Profiles
When it comes to user profiles, SPSS attracts a varied clientele, each with their unique requirements.
- Researchers and Academics: Individuals in academic settings and research institutions frequently turn to SPSS due to its comprehensive statistical tools that suit rigorous research standards. It provides the depth needed for hypothesis testing and complex analysis.
- Healthcare Analysts: Those in the healthcare field recognize SPSS as a valuable asset for analyzing patient data, performance metrics, and clinical trials, aiding in data-driven decision-making.
- Market Researchers: Professionals in market research leverage SPSS for consumer data analysis, helping them glean insights into consumer behavior and market trends.
- Small Business Owners: While SPSS may seem daunting at first, small business owners looking to understand their market or enhance their business strategies can benefit from its accessible data analysis tools.
The versatility in user profiles connected with SPSS allows it to support data analysis efforts in diverse contexts, further cementing its role as a critical tool in decision-making processes.
"IBM SPSS Statistics empowers users to unlock the potential tucked away in their data, driving informed decisions across sectors."