Enhancing Clinical Decision-Making in Healthcare
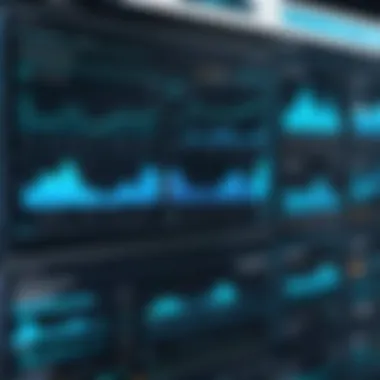
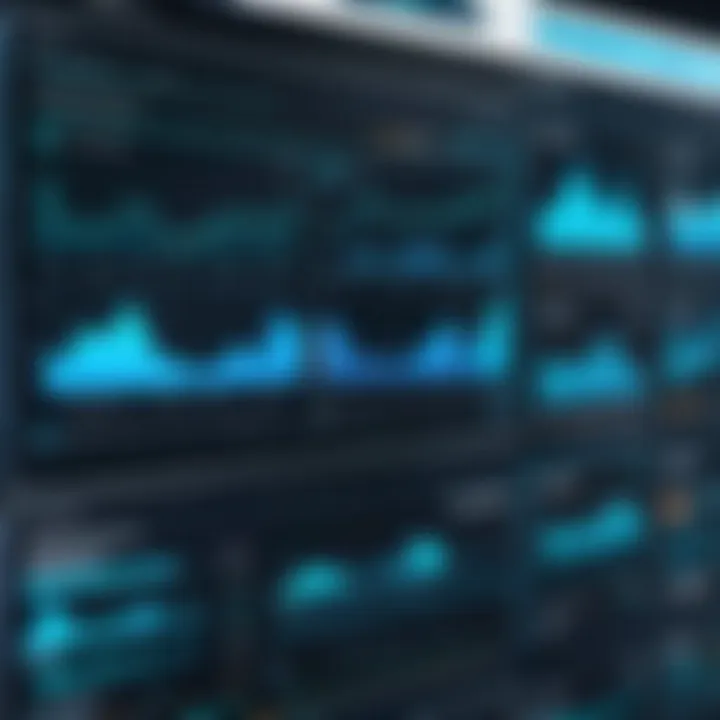
Intro
The landscape of healthcare is not just about medicines and surgeries anymore; it’s increasingly about making precise and timely decisions. As the stakes grow higher and patient needs evolve, decision support systems (DSS) have become an essential part of the clinical decision-making process. These systems integrate complex data, provider knowledge, and patient information, all to boost healthcare efficiency and accuracy.
But why should we care about decision support in healthcare? For one, the wrong call can lead to dire consequences; hence, leveraging technology to inform choices is crucial. This article will delve into how these systems work, what makes them beneficial, and why they're critical for both small clinics and large hospitals.
Key Features and Benefits
Overview of Features
At their core, decision support systems offer several features that stand out:
- Data Integration: They aggregate data from various sources, including electronic health records and lab results, creating a comprehensive view of patient data.
- Real-Time Feedback: DSS provide immediate suggestions or alerts based on the current data, making healthcare professionals’ jobs easier and more accurate.
- Clinical Guidelines: They often incorporate current medical guidelines and research, aiding clinicians in staying updated with the latest best practices.
Benefits to Users
The benefits of using decision support systems in healthcare are manifold:
- Improved Patient Outcomes: By offering tailored advice based on individual patient data, DSS enhance the quality of care given, leading to better health results.
- Efficiency Gains: Healthcare professionals can make decisions quickly, reducing waiting times for patients and optimizing workflow.
- Error Reduction: With data-driven recommendations, the risk of human error decreases significantly, ensuring safer patient care.
"Decision support isn't just a nice-to-have; it's an integral part of modern healthcare that can redefine patient experiences and outcomes."
Comparison with Alternatives
Head-to-Head Feature Analysis
When comparing decision support systems with alternative methods like traditional decision-making or even basic data management software, there are noticeable differences. For instance:
- Traditional Decision-Making: Heavily reliant on the expertise of the healthcare provider without any data support, often limited to what’s previously known or documented.
- Basic Data Management: Lacks the predictive analytics and contextual recommendations that DSS provide, making it less capable in guiding clinical choices.
Pricing Comparison
When it comes to costs, the investment in a robust decision support system can vary significantly. These costs take into account:
- Initial Setup: Licensing fees and installation costs can be steep, particularly for larger systems with extensive capabilities.
- Maintenance Fees: Ongoing costs for software updates and support can add up, especially for comprehensive systems.
- Training Costs: It's not just about buying the software; organizations must also budget for training staff to use it effectively.
In summary, decision support systems offer a comprehensive solution tailored to meet the needs of today’s healthcare professionals, paving the way for a more efficient, error-free atmosphere in clinical settings. As technology continues to advance, embracing these systems may just be what the future of healthcare demands.
Prelude to Decision Support in Healthcare
In the ever-evolving landscape of healthcare, decision-making has become increasingly complex. With the wealth of information available, healthcare professionals often find themselves leveraging decision support systems to efficiently navigate clinical challenges. The importance of decision support in healthcare cannot be overstated; it serves as a bridge between patient care and the latest medical knowledge, promoting improved outcomes and more efficient workflows.
One of the most relevant aspects of decision support systems (DSS) is their ability to transform raw data into actionable insights. Traditionally, healthcare professionals have had to rely on their expertise alone when making decisions. However, decision support tools enhance this process by synthesizing large volumes of data, thereby facilitating a more informed decision-making environment.
Defining Decision Support
Decision support can be defined as a technology-enabled application designed to help healthcare leaders and professionals make well-informed decisions. Think of it as a compass guiding them through the vast sea of clinical knowledge and patient information. By incorporating guidelines, best practices, and case data, decision support functions to reduce ambiguity and mitigate the risks associated with clinical decisions.
At the heart of these systems are a variety of components, which may include data management systems, algorithms, and prediction models. These systems are tailored to meet specific requirements, ranging from clinical decision support systems (CDSS) to operational decision support systems, offering targeted assistance across different domains of healthcare. Effectively, they hold the potential to change how decisions are made, ultimately leading to enhanced patient safety and quality of care.
History and Evolution of Decision Support Systems
The journey of decision support systems in healthcare began decades ago but has transformed dramatically over time. In the early days, the focus was largely on creating rudimentary tools aimed primarily at data management. As computers became more accessible in the late 20th century, researchers began to explore the potential of these systems to aid clinical judgement.
Fast forward to the present day, and we find ourselves in an age dominated by sophisticated algorithms and artificial intelligence. The evolution from simple data archiving systems to today’s advanced machine learning applications illustrates a significant advancement in our approach to healthcare decision-making. Here are some key milestones:
- 1970s: Initial use of computer-based information systems in hospitals.
- 1980s: Development of the first basic clinical decision support systems, focusing on drug interaction alerts.
- 1990s: Integration of clinical practice guidelines, enhancing the ability of systems to support diagnosis and treatment options.
- 2000s and beyond: The emergence of machine learning and big data analytics, enabling predictive analytics and personalized medicine.
"The history of decision support systems serves as a reminder of how technology can transform healthcare, paving the way for a more precise and effective approach to patient care."
As we look to the future, it's clear that the foundational technology and data integration will only improve, further revolutionizing healthcare decision-making. In the coming sections, we will delve deeper into the types of decision support systems, their technological frameworks, and the profound influence of artificial intelligence on this evolving domain.
Types of Decision Support Systems
In the realm of healthcare, decision support systems hold a crucial place, acting as facilitators in the decision-making process. Distinguishing among various types of decision support systems (DSS) enables healthcare providers to choose the right tools for specific tasks, enhancing overall patient care and operational efficiency. Each type plays a unique role, from improving clinical outcomes to streamlining healthcare administration, which is vital for adapting to the ever-changing demands of modern healthcare environments.
Clinical Decision Support Systems (CDSS)
Clinical Decision Support Systems are particularly designed for assisting healthcare professionals in making informed clinical decisions. These systems provide evidence-based knowledge in the form of alerts, reminders, and patient-specific information. A practical example might include a system that alerts a physician to potential drug interactions based on a patient’s current medications.
The significance of CDSS cannot be overstated; they not only enhance the quality of care but also reduce the risk of medical errors. By integrating patient data and guidelines from trusted medical sources, CDSS ensure that healthcare providers have the most relevant information at their fingertips.
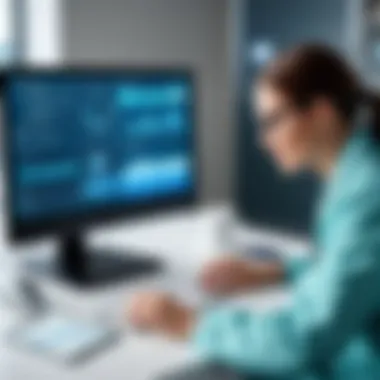
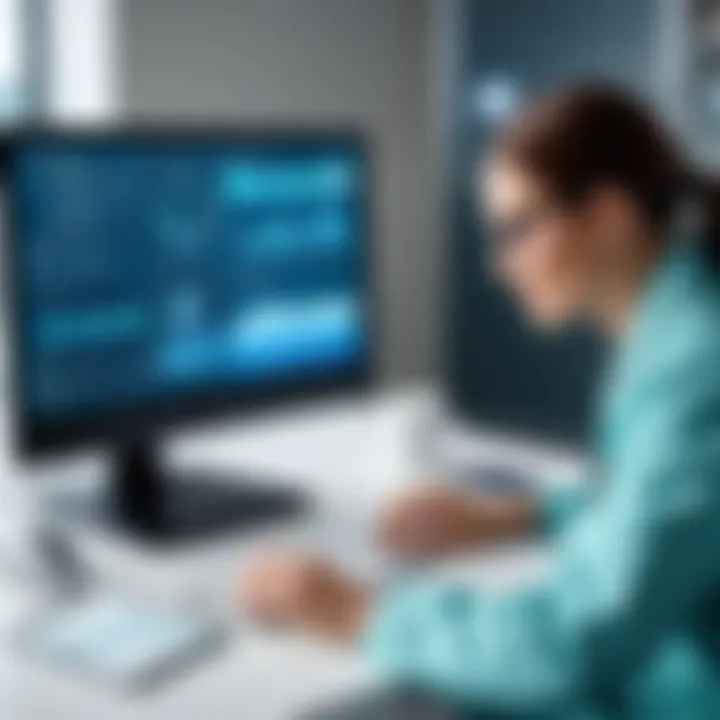
- Benefits of CDSS:
- Improves diagnostic accuracy
- Supports adherence to clinical guidelines
- Enhances patient engagement by sharing insights
However, it’s important to approach CDSS implementation with caution. Considerations such as data privacy, the potential for alert fatigue, and the need for seamless interoperability with existing medical systems must be taken into account.
Operational Decision Support Systems
Operational Decision Support Systems address various logistical challenges faced in healthcare settings. These systems optimize scheduling, resource allocation, and budget management, ensuring that hospitals can run smoothly under tight constraints. Think of a scenario where an operational DSS is used to allocate nursing staff based on real-time patient acuity data. By aligning resources dynamically with patient needs, hospitals can significantly improve both care quality and operational efficiency.
In essence, operational DSS not only reduce waste but also enhance productivity, making them invaluable tools for healthcare administrators.
- Key Elements of Operational DSS:
- Resource Management
- Patient Flow Optimization
- Financial Planning and Analysis
Adopting operational DSS is not without challenges—issues such as technology costs, staff training, and data integration persist and need effective strategies for resolution.
Management Decision Support Systems
Management Decision Support Systems focus on strategic decision-making within healthcare organizations. These systems assimilate data from various operational sectors, providing insights that guide long-term planning and policy-making. For instance, a management DSS might analyze patient satisfaction data, financial reports, and clinical outcomes to assist executives in identifying areas for improvement within their institutions.
Using data analytics tools, these systems can help executives make informed, strategic choices that align with organizational goals.
- Advantages of Management DSS:
- Enhanced strategic planning
- Data-driven decision-making
- Improved organizational accountability
Nevertheless, the successful implementation of Management DSS hinges on high data quality and accessibility, along with a culture that embraces data utilization at all levels of the organization.
In the fast-paced world of healthcare, understanding the distinctions among different types of decision support systems is essential for leveraging their potential effectively.
In summary, the landscape of decision support systems is diverse, each type catering to specific needs across the healthcare spectrum. By grasping the roles and benefits of Clinical, Operational, and Management Decision Support Systems, healthcare providers can better equip themselves for the challenges ahead.
Technological Framework for Decision Support Systems
In contemporary healthcare, the backbone of decision support systems (DSS) lies in its technological framework. This framework bridges the gap between data and actionable insights, playing a critical role in enhancing clinical efficiency and patient care. Without a robust technological foundation, these systems cannot function effectively, which makes understanding its components essential for IT and software professionals engaged in healthcare solutions.
Data Sources and Integration
To facilitate effective decision-making, DSS relies heavily on diverse data sources. These can range from Electronic Health Records (EHRs), lab test results, radiology images, to even wearable health tech data. In this digital age, the integration of these varied data sources is crucial. It’s like putting together a puzzle where every piece tells a part of the patient's story.
- Types of Data:
- Structured Data: This includes standard medical records like patient demographics and lab reports, typically organized in tables.
- Unstructured Data: This can be found in clinical notes or radiology images, which are often more challenging to process but incredibly insightful.
Integration involves not just gathering data but ensuring that systems communicate smoothly. APIs (Application Programming Interfaces) often come into play here, acting as intermediaries that allow different software to speak the same language. If data integration is executed incorrectly, it can lead to discrepancies that complicate clinical decisions.
Algorithm and Analytical Methods
Once the data is gathered and integrated, the next step is employing algorithms and analytical methods to derive meaningful insights. Think of algorithms as recipes guiding clinicians through complex data. They help in identifying patterns that might not be readily visible to the human eye.
- Predictive Analytics: This method utilizes historical data to predict future outcomes, potentially alerting healthcare providers about patients at risk of complications.
- Prescriptive Analytics: Unlike predictive analytics, which forecasts future events, prescriptive analytics suggests actions to take in various scenarios.
For example, algorithms can analyze a patient's past medical history alongside current clinical presentations to suggest personalized treatment plans. However, developing these algorithms requires a deep understanding of both healthcare processes and data science.
User Interfaces and Interaction
The effectiveness of any decision support system hinges on its user interface (UI) and how healthcare professionals interact with the technology. An intuitive, easy-to-navigate interface can encourage user adoption and enhance the decision-making process.
- Design Principles:
- Simplicity: Complicated interfaces can lead to errors, hence UIs must present information clearly.
- Real-time Feedback: Engaging users with immediate responses can assist in confirming their actions or decisions.
- Customization: Allowing users to tailor dashboards or reports according to their needs can make systems more user-friendly.
A well-designed user interface creates a seamless experience, enabling clinicians to focus on patient care rather than struggling with technology.
As we dive deeper into the technological framework of decision support systems, it's clear that the interplay between data, analysis, and interface quality directly impacts the quality of care providers can deliver.
In summation, the technological framework for decision support systems is multifaceted, drawing on integrated data sources, smart algorithms, and intuitive user interfaces. These components work in harmony, translating raw data into insights that enhance clinical decision-making and ultimately, patient outcomes.
The Role of Artificial Intelligence in Decision Support
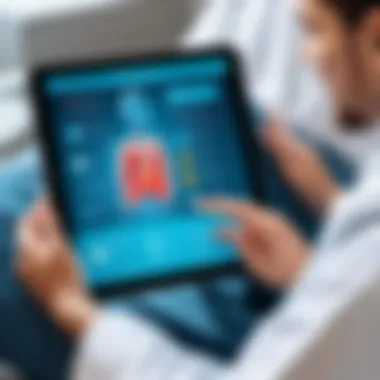
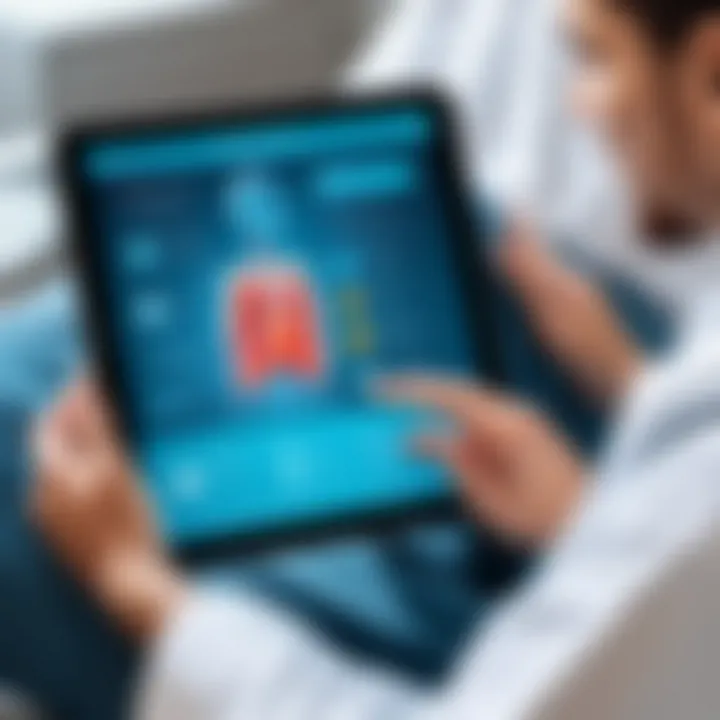
The advent of Artificial Intelligence (AI) has ushered in a new era in the realm of decision support within healthcare. Its integration into various systems not only augments the efficiency of clinical workflows but also enriches the quality of patient care. By leveraging data and algorithms, AI can aid healthcare professionals in making informed decisions that could lead to better health outcomes. Understanding how AI fits into this landscape is crucial. The transformative potential of AI lies in its ability to learn from vast datasets, recognize patterns, and assist clinicians in navigating complex medical scenarios.
Machine Learning Applications
Machine learning is a subset of AI that involves training algorithms on substantial amounts of data to improve their performance over time. In healthcare decision support, machine learning applications can dramatically enhance diagnostic accuracy and prediction capabilities. For instance, consider a machine learning model designed to predict patient readmission risks. By analyzing historical patient data, including demographics, medical history, and treatment history, the model can identify the likelihood of a patient being readmitted within a certain timeframe.
This capability enables healthcare providers to implement timely interventions, such as tailored discharge planning or follow-up appointments, thus reducing unnecessary readmissions and ultimately enhancing patient care. Another compelling application is in radiology, where machine learning can assist in detecting anomalies in medical images, such as X-rays or MRIs. The speed and precision of these models can aid radiologists by flagging areas of concern, allowing for a more accurate and efficient review process.
The continued advancements in machine learning methods not only improve these applications but also scavenge through the vast pools of healthcare data that can often feel like finding a needle in a haystack.
Natural Language Processing in Healthcare
Natural Language Processing (NLP) is another critical component of AI in decision support. NLP involves the ability of machines to understand and interpret human language as it is spoken or written, which is invaluable when dealing with the intricate nature of medical documentation. The vast amount of unstructured data generated in healthcare settings, such as clinical notes, research articles, and patient feedback, can be overwhelming. NLP provides a means to distill this information into actionable insights.
For example, healthcare systems can utilize NLP tools to extract relevant details from clinical notes to assist in patient assessments or even flag potential safety issues. By monitoring patient feedback across different sources, such as social media or surveys, organizations can gain insights into public sentiment and areas for improvement.
Moreover, when combined with decision support systems, NLP aids in creating chatbots that can efficiently handle patient inquiries. These bots can process patient symptoms, offer preliminary advice, and guide patients to appropriate care channels, ensuring they receive timely assistance. In this sense, NLP acts as a bridge by connecting vast information reservoirs with clinical applications, thus facilitating better decision-making.
Ultimately, the incorporation of AI technologies like machine learning and NLP not only optimizes processes but also empowers clinicians to focus more on patient care rather than administrative tasks.
By continually evolving and integrating into healthcare systems, AI truly reshapes how decisions are made and ultimately leads to enhanced patient experiences and outcomes.
Benefits of Decision Support Systems
Decision support systems (DSS) silently but steadily transform the healthcare landscape. They serve as backbone tools in enabling clinicians to make informed choices that directly affect patient care. Understanding the key benefits of these systems is vital for both healthcare providers and IT professionals involved in system design and integration. Several specific elements underline their significance and implications across the board.
Improved Patient Outcomes
One of the most compelling advantages of decision support systems is their role in improving patient outcomes. When medical professionals harness the power of DSS, they can base their decisions on comprehensive data analysis, rather than instinct or outdated practices. For instance, systems like Epic and Cerner provide alerts for potential drug interactions or flag critical lab results, steering clinicians towards appropriate actions without missing crucial details.
Moreover, studies consistently indicate lower error rates in clinical settings equipped with robust decision support tools. A noteworthy research piece by the Journal of the American Medical Informatics Association highlighted that hospitals using DSS demonstrated a significant decrease in medication errors, which ultimately leads to healthier patients and reduced readmission rates.
"The right decision at the right time can save lives. DSS is the compass that guides healthcare professionals in the stormy sea of medical choices."
Enhanced Workflow Efficiency
Efficiency is another area where DSS shine. With features designed to streamline processes, decision support systems help reduce the time it takes for healthcare providers to access necessary information. Instead of sifting through paperwork or looking up data individually, systems can present summarized patient histories, treatment guidelines, and even predictive analytics based on past data—all at the click of a button.
In practices where time is of the essence, this efficiency can have far-reaching consequences. A surgery center utilizing a DSS for scheduling and resource management reported a reduction in patient wait times by 30%. This not only improves patient satisfaction but also optimizes the use of clinical staff and resources, thus increasing throughput without sacrificing quality.
Support for Evidence-Based Practices
Lastly, DSS supports the shift towards evidence-based medicine—a pivotal trend in modern healthcare. By integrating clinical guidelines and protocols directly into workflows, these systems ensure that healthcare decisions align with the latest research findings. For example, tools like UpToDate provide instant access to treatment recommendations that are regularly updated to reflect new evidence.
The incorporation of DSS in promoting evidence-based practices promises consistency and fosters a culture of continuous improvement within healthcare institutions. Clinical teams are more empowered to follow guidelines, knowing they have a reliable source backing their decisions. Consequently, this leads to standardized practices across departments, enhancing overall quality of care.
In sum, the benefits of decision support systems in healthcare extend beyond mere convenience. They represent a strategic framework that aids in clinical decision-making, enhances operational efficiency, and ensures adherence to best practices—ultimately paving the way for a more effective healthcare system.
Challenges in Implementing Decision Support Systems
Implementing decision support systems (DSS) in healthcare is no walk in the park. While these systems hold the promise of enhancing clinical decision-making and improving patient outcomes, several hurdles can crop up along the way. Addressing these challenges is crucial not just for the successful deployment of DSS but for ensuring that they are effectively integrated into daily practice. Understanding these obstacles can lead to informed strategies that leverage the full potential of decision support technology.
Integration with Existing Systems
One of the the most significant challenges in adopting decision support is integration, or rather the lack thereof. Many healthcare facilities rely on legacy systems that run on outdated technology. These systems often don’t play nice with newer DSS platforms, creating compatibility issues. Without seamless integration, the data that fuels decision support systems can become trapped in silos, effectively rendering the entire initiative moot.
For example, let’s consider a hospital that employs an advanced clinical decision support system for medication management. If this system cannot pull data from the electronic health records (EHR) already in use, healthcare professionals might not receive alerts for drug interactions or allergies. This disconnect not only diminishes the system's effectiveness but also poses inherent risks to patient safety.
Key Considerations:
- Standards and Protocols: Adopting industry standards helps foster better interoperability.
- Continuous Updates: Regularly updating systems can help mitigate integration problems.
- Collaboration: Vendor collaboration is crucial to ensure all systems can communicate efficiently.
User Acceptance and Training Issues
Even the most advanced DSS can fall flat if the end-users—healthcare professionals—do not accept them. Changes around the hospital often face the brunt of resistance. Healthcare workers accustomed to their traditional methods may see these new tools as unnecessary overhead rather than a valuable ally in patient care.
In this situation, a robust training program becomes a linchpin for success. Training not only eases the transition but also fosters confidence among staff members, clarifying the benefits that the systems offer. When employees feel comfortable with the technology, they’re more likely to embrace it, leading to improved usage rates and enhanced patient outcomes.
"Change is hard, and it's no different in healthcare. Staff need to see that these tools will actually lighten their load rather than add to it."
Points to Address:
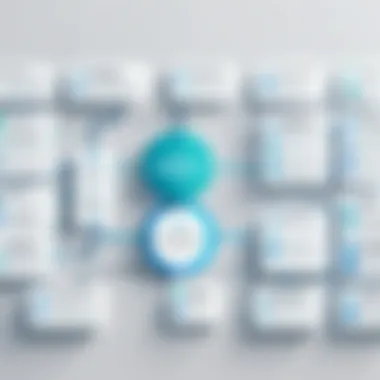
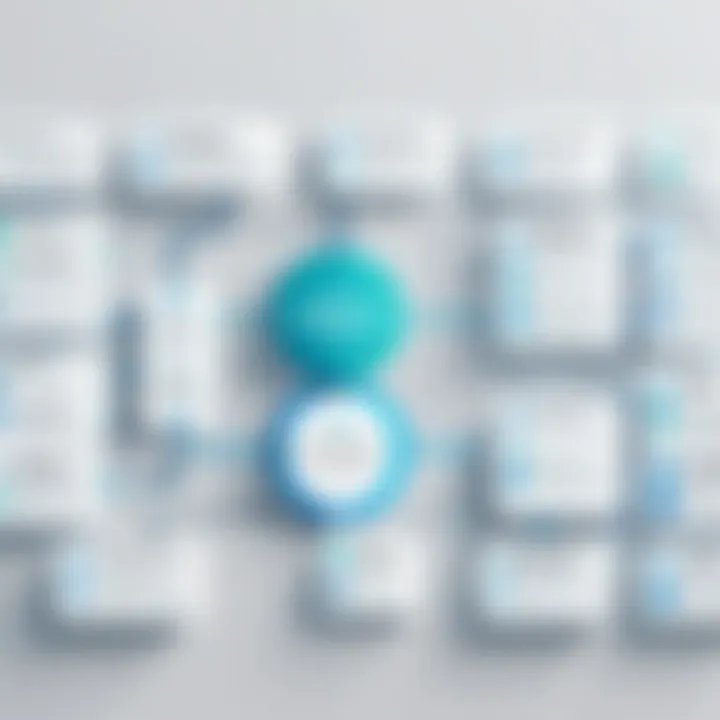
- Tailored Training Programs: One-size-fits-all training can backfire. Customized sessions take individual needs into account.
- Ongoing Support: Make sure there’s a help desk or support team readily available as questions arise.
- Feedback Mechanisms: Implementing user feedback can help providers refine the system for better usability.
Data Quality and Availability
Data is the lifeblood of decision support systems. However, if the available data is either poor in quality or sparse, the entire system could become ineffective. Inaccurate, outdated, or incomplete data can lead to erroneous conclusions, which can have real-world consequences for patient care.
Establishing solid data governance practices is essential in this regard. Consistent data entry standards, regular audits, and validation processes can help maintain high data quality. Healthcare institutions must also address gaps in data availability. If certain patient data is missing—as can happen with fragmented care—the DSS cannot provide complete insights, thus compromising its utility.
Critical Elements Include:
- Regular Audits: Conducting routine checks ensures data meets quality standards.
- Cross-Departmental Collaboration: Departments should work together to guarantee data consistency and completeness.
- Investing in Upgrades: Sometimes, improving data collection tools is necessary for obtaining better quality data.
In summary, while implementing decision support systems is fraught with challenges, a proactive approach addressing integration issues, user acceptance, and data quality can pave the way for greater success in healthcare delivery. Recognizing these hurdles reveals an opportunity to refine processes and ultimately enhance patient outcomes.
Future Directions in Decision Support for Healthcare
The landscape of decision support in healthcare is evolving at a breakneck pace. The future holds immense potential for improving patient care and operational efficiency through emerging technologies and innovative approaches. As healthcare becomes increasingly data-driven, understanding how these advancements can be harnessed is critical for professionals across the board – from IT experts to healthcare providers.
Emerging Technologies and Innovations
The integration of innovative technologies in decision support systems is set to revolutionize how healthcare is delivered. One of the most promising areas is the utilization of big data analytics. By analyzing vast amounts of health data, healthcare professionals can identify trends that inform preventive measures. For instance, mining data from various sources, such as electronic health records and genomics, provides deeper insights into population health trends. This could lead to more accurate predictions about disease outbreaks and effectively allocate resources before a crisis hits.
Another noteworthy advancement is the incorporation of wearable technology. Devices such as smartwatches and health trackers gather real-time data on patients' vitals. This data can be used not only for ongoing monitoring but also for immediate decision-making in clinical situations. Healthcare providers can receive alerts if a patient's health deteriorates, ensuring timely interventions.
Furthermore, virtual reality (VR) and augmented reality (AR) applications are making headway. They serve as innovative training tools for healthcare professionals. For example, surgeons can practice complex procedures in a simulated environment before performing them in real life, thereby minimizing risks and improving outcomes.
Shifting Regulatory Landscapes
As technologies evolve, so too do the regulations governing their use. Understanding this shifting landscape is essential for the successful implementation of decision support systems. Regulatory bodies are increasingly focused on ensuring patient data privacy and safety while also fostering innovation. For instance, the Health Insurance Portability and Accountability Act (HIPAA) mandates strict guidelines on data handling but allows for the exploration of new methods, provided they uphold patient confidentiality.
Adapting to these changes requires that healthcare organizations remain agile in their approach. Ongoing education about current compliance standards is vital. Additionally, stakeholders need to actively engage with regulatory bodies to influence policies that can foster innovation while safeguarding patients.
Investing in staff training to navigate regulatory changes can empower teams to fully utilize decision support tools.
Case Studies: Decision Support Systems in Action
Understanding the practical implications of decision support systems (DSS) in healthcare is vital for several reasons. Case studies illuminate the capabilities and limitations of these systems, showing their real-world impact. With the healthcare landscape constantly evolving, concrete examples from hospitals can help guide future implementations, highlighting both successes and areas needing improvement.
Success Stories from Hospitals
When it comes to decision support systems, success stories can serve as powerful testimonials. Just think of instantiation of the Epic's clinical decision support tools within various institutions. Epic has produced a number of case studies that attribute tangible reductions in medication errors and better adherence to clinical guidelines directly to its deployment.
For instance, consider the experience of the Mount Sinai Health System in New York. By implementing Epic's CDS, they have made great strides in improving patient safety. In one reported case, the system's alerts regarding potential drug interactions led to the identification of errors before they could reach the patient. This proactive approach has resulted in a significant drop in adverse drug events, an essential metric when assessing care quality.
Another noteworthy example is the implementation of Stanson Health's CDS at a community health clinic in California. This system focuses on preventive care and chronic disease management. By integrating Stanson's tools, the clinic saw a rise in compliance with screening guidelines. The approach allowed healthcare providers to understand better which patients needed preventive measures, improving overall health outcomes in the community.
"Case studies show that when implemented effectively, decision support systems can dramatically improve patient care and safety."
Lessons Learned from Failures
While it’s easy to highlight success stories, failures carry lessons that are equally valuable. Understanding what went wrong can help organizations avoid similar pitfalls in the future.
A notable case involved a well-known hospital that rushed the deployment of a leading decision support system. The new platform came with numerous features, yet the hospital staff received insufficient training on using these tools effectively. Consequently, instead of aiding decision-making, the system became an obstacle. Reports indicated that some healthcare workers began ignoring alerts, believing them to be irrelevant, which compromised patient safety instead of enhancing it.
Similarly, a community hospital’s implementation of a vendor-specific CDS was met with resistance due to lack of integration with existing electronic health records. This mismatch caused significant disruptions, leading to a decline in morale among staff members who felt overburdened with redundant tasks. The takeaway here is clear: thorough user acceptance testing and seamless integration are crucial. Without thoughtful consideration of how new systems will mesh with existing workflows, organizations may end up complicating processes rather than simplifying them.
In concluding this section, it’s essential to note that both successes and failures provide invaluable insight into DSS in healthcare. Real-world case studies offer a roadmap that incorporates lessons from experience, enabling healthcare entities to sift through complexities and leverage decision support systems more effectively.
End: The Impact of Decision Support on Healthcare Delivery
The role of decision support systems in healthcare cannot be overstated. These systems are not just tools; they represent a transformative force that shapes how healthcare is delivered on many levels. By improving clinical decision-making, decision support systems actively contribute to better patient care, optimizing resource use, and enhancing the efficiency of healthcare operations.
Summary of Key Insights
- Enhanced Decision-Making
Decision support systems empower healthcare providers with timely access to critical information. This ensures that decisions made are based on the most current data, guidelines, and patient histories. It reduces the cognitive load on doctors, allowing them to focus on patient interaction rather than sifting through heaps of information. - Improved Patient Outcomes
The integration of decision support tools has shown a direct correlation with improved patient outcomes. For instance, automated reminders for preventive care have led to increased vaccinations and regular screenings, bridging the gap between recommended practices and their implementation in real scenarios. - Workflow Efficiency
By streamlining processes, decision support systems eliminate redundancies in patient care pathways. Clinicians can avoid unnecessary tests or treatments through intelligent recommendations, thereby not just enhancing the patient experience but also conserving the financial resources tied up in superfluous healthcare costs. - Support for Clinical Guidelines
These systems offer clinicians a framework for following established clinical guidelines. They help in the application of evidence-based practices, ensuring that all decisions are aligned with the best possible standards of care.
Looking Ahead: Opportunities and Risks
As decision support systems evolve, so do the opportunities and risks associated with them.
- Opportunities:
- Risks:
- With the advent of machine learning and AI capabilities, systems will only get smarter. They can predict outcomes based on historical data and assist in personalized treatments that were previously unattainable. This personalized approach is at the heart of the promise of precision medicine.
- Integration with wearable technology and remote monitoring solutions opens a new frontier. Real-time data can be analyzed, providing opportunities for proactive interventions, thereby reducing complications in chronic illnesses.
- However, heavy reliance on these systems can lead to concerns about data privacy and security. As systems grow in complexity, they can become targets for cyberattacks, potentially compromising sensitive patient information.
- Also, a lack of transparency in how decisions are derived from algorithms can create issues of trust among providers. If clinicians cannot understand the reasoning behind a recommendation, there may be hesitance to adopt these systems.
In summation, decision support systems represent a valuable asset in the realm of healthcare. However, as we advance into the future, a balanced approach will be necessary—one that embraces the benefits while judiciously managing the risks inherent in these powerful tools. The conversation about decision support will only deepen as these technologies continue to evolve.