Exploring the Landscape of Fraud Detection Systems
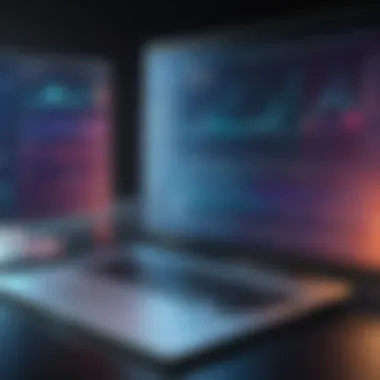
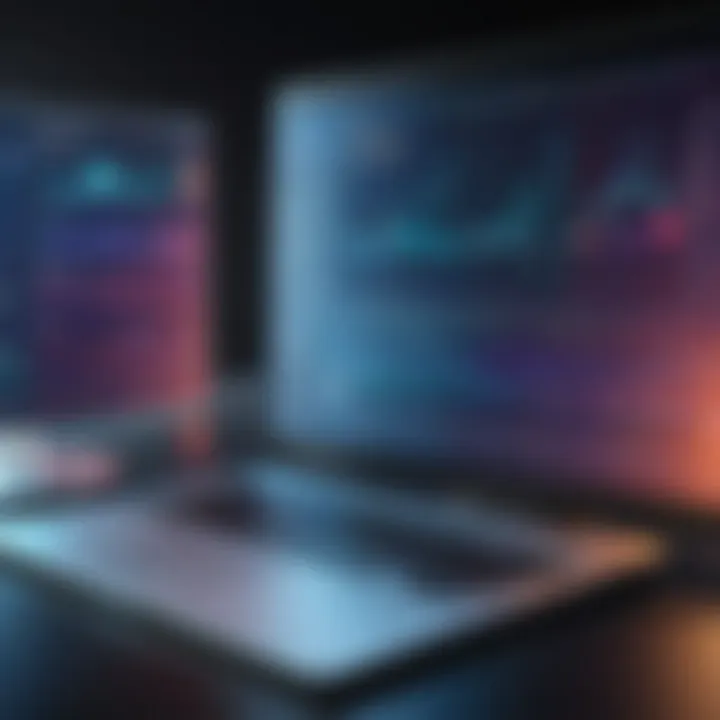
Intro
Fraud detection systems have become an essential component in the fight against fraudulent activities that pose risks to businesses and individuals alike. As fraud schemes evolve, so do the methods used to combat them. These systems are designed not only to identify potential threats but also to provide early warnings, thereby minimizing damages. Understanding these systems requires a detailed look at their functionality, how they integrate technology, such as artificial intelligence, and the challenges companies face in implementation.
Key Features and Benefits
Overview of Features
Fraud detection systems offer several key features that work together to enhance their effectiveness:
- Real-Time Monitoring: Continuous analysis of transactions helps in the immediate identification of suspicious activities.
- Data Analytics: Advanced algorithms sift through large datasets to detect patterns that may indicate fraud.
- User Behavior Analytics: By establishing a baseline of normal user behavior, these systems can quickly flag anomalies that warrant further investigation.
- Integration with Other Systems: Their capability to integrate seamlessly with existing business processes and software increases their utility.
- Alerts and Reporting: Timely alerts can help organizations respond proactively to threats. Comprehensive reporting aids in regulatory compliance.
Benefits to Users
The advantages of implementing a fraud detection system are profound:
- Reduction in Financial Loss: Early detection of fraudulent activities can save organizations from significant financial losses.
- Enhanced Security: Establishing an advanced fraud detection system projects a commitment to security, improving stakeholder confidence.
- Operational Efficiency: Automating fraud detection reduces the workload of compliance and security teams, allowing them to focus on more strategic tasks.
- Data-Driven Insights: Organizations benefit from insights and analytics which can inform policy changes and operational strategies.
"Implementing a robust fraud detection solution not only mitigates risks but also empowers businesses to operate confidently in a technology-driven landscape."
Comparison with Alternatives
Head-to-Head Feature Analysis
When evaluating different solutions, it is crucial to compare their features:
- Traditional Methods: While often based on manual checks, they are limited in their ability to process data quickly.
- Machine Learning Options: Systems that utilize machine learning offer adaptive learning capabilities, improving over time as they encounter new data.
- Hybrid Solutions: Combining traditional methods with machine learning provides a balanced approach, maximizing the strengths of both.
Pricing Comparison
Pricing varies widely based on system capabilities:
- Traditional Systems: Generally have lower initial costs, but can lead to higher costs over time due to labor and potential losses.
- AI-Based Solutions: Often involve higher upfront investment but can provide long-term savings through efficiency and accuracy.
- Subscription Models: Some companies offer tiered pricing based on service levels, which can be beneficial for small and large businesses alike.
As technology continues to advance, understanding the intricacies of fraud detection systems will be crucial for organizations striving to protect themselves. The effectiveness of these systems relies on their adaptability and their continued evolution in response to emerging threats.
Foreword to Fraud Detection Systems
Fraud detection systems play a vital role in modern business environments, as they combat the pervasive issue of fraudulent activities. These systems are designed to identify suspicious behavior and mitigate risks associated with financial losses. In a world where cyber threats and deceitful tactics continually evolve, organizations must adopt robust fraud detection frameworks to safeguard their assets. The importance of this topic lies in its direct impact on the financial health and reputation of businesses.
A well-functioning fraud detection system can enhance the trust of consumers, which is essential for competition in various sectors. It helps businesses not only in managing risks but also in complying with regulations mandated by governing bodies. With technology advancing, the integration of such systems into existing infrastructure is not just a temporary solution, but a necessary strategy for future resilience.
This article will demystify the overarching structure of fraud detection systems. Several components will be examined, such as the types of fraud, data analysis techniques, and the technologies involved. Understanding these elements provides critical insights into how businesses can effectively implement and improve their fraud detection measures.
Defining Fraud Detection Systems
Fraud detection systems refer to a set of algorithms and methodologies designed to identify fraudulent behavior. These systems utilize various data sources and analytical techniques to recognize patterns, anomalies, and inconsistencies indicative of fraud. The operational mechanics of these systems are multifaceted, involving data input, processing, and output stages.
The primary goal of these systems is to minimize the occurrence of fraudulent activities by alerting users to suspicious patterns. They can be classified into several types based on their approach, such as rule-based systems or machine learning algorithms. Each type presents unique advantages and challenges.
Importance of Fraud Detection
Fraud detection is crucial for maintaining organizational integrity and financial stability. Businesses face significant risks, including monetary loss, legal repercussions, and reputational damage, when fraud occurs. Implementing effective fraud detection systems not only protects against these threats but also enhances overall operational efficiency.
Here are some key reasons why fraud detection is essential:
- Protects Assets: Identifying fraudulent activities helps preserve financial resources, which is fundamental for any business's sustainability.
- Enhances Reputation: By showing a commitment to security, companies can build customer trust, an invaluable asset in today’s competitive market.
- Regulatory Compliance: Many industries are subject to regulations demanding effective fraud prevention measures. Compliance is necessary to avoid penalties and ensure smooth operations.
- Real-Time Monitoring: Advanced fraud detection systems provide real-time alerts, enabling businesses to react promptly to threats before they escalate.
"A proactive approach to fraud detection is far more effective than a reactive one."
In summary, understanding the fundamentals of fraud detection systems is crucial to safeguarding business operations. As fraud tactics continue to become more sophisticated, the necessity for innovative fraud detection measures only grows.
Types of Fraud
Understanding the various types of fraud is a cornerstone in developing effective fraud detection systems. Different types of fraud not only require unique detection techniques but also present distinct challenges that organizations must navigate. Recognizing these nuances is essential for IT professionals, software developers, and businesses of all sizes as they aim to protect their assets and maintain trust with customers. By educating oneself on the numerous types of fraud, one can bolster the defense mechanisms in place and adapt strategies accordingly. Each fraud type brings specific patterns and indicators that systems must identify to mitigate risks before they escalate.
Financial Fraud
Financial fraud encompasses a broad range of malicious activities aimed at illegally obtaining money or property. This category includes schemes like credit card fraud, investment scams, and Ponzi schemes. These actions can have devastating effects on individuals and corporations alike, leading to significant financial losses and reputational damage.
To detect financial fraud, systems typically employ both real-time monitoring and predictive analytics. For instance, when unusual spending patterns arise, the system can trigger alerts to investigators for further analysis. Additionally, organizations can establish machine learning algorithms that constantly adapt to evolving fraud tactics, ensuring more robust defenses.
Identity Theft
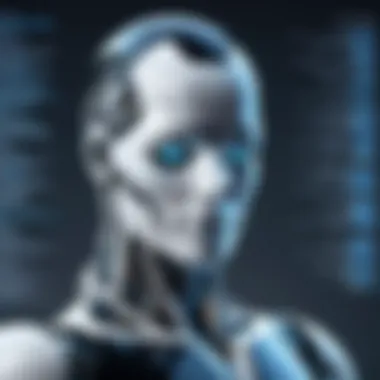
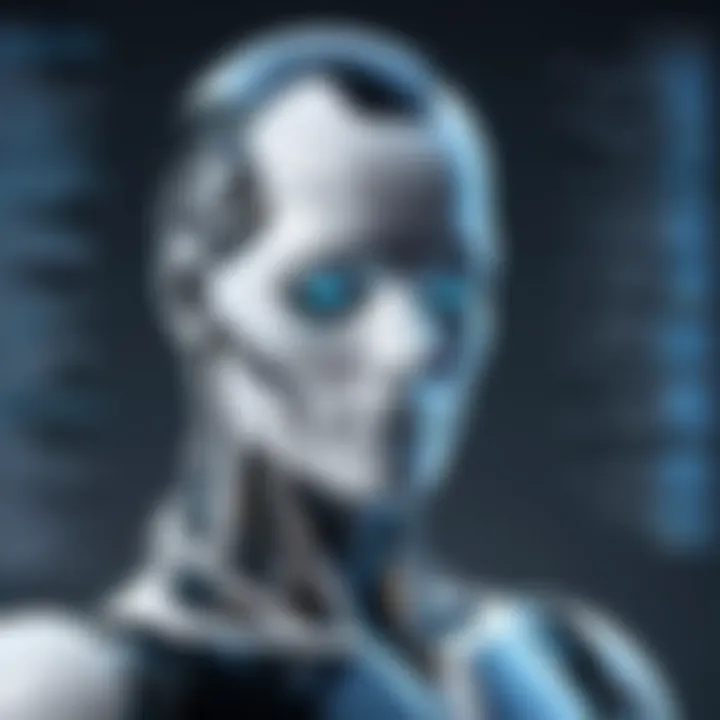
Identity theft refers to the unauthorized use of someone else's personal information, often for financial gain. This fraud type has been increasingly facilitated by advancements in technology, particularly through online platforms. Thieves may leverage stolen personal data to open credit accounts, conduct high-value purchases, or commit various forms of fraud.
Organizations can combat identity theft through proactive measures, such as multi-factor authentication and encryption of sensitive information. Additionally, education plays a vital role; users need to be aware of phishing attacks and other tactics that threaten their personal data. By implementing a combination of technology and user awareness, firms can significantly decrease the risk of identity theft.
Insurance Fraud
Insurance fraud occurs when individuals or organizations deceive an insurance provider to receive benefits that they are not entitled to. Common examples include exaggerating claims, staging accidents, or even submitting false claims altogether. Insurance fraud not only escalates premiums for consumers but also strains the industry's resources.
Fraud detection systems in insurance often utilize claims data analysis along with historical patterns to flag suspicious claims. For instance, certain claim characteristics, like the frequency of claims from a specific individual, can signal potential fraud. Recognizing these patterns is crucial for insurers to maintain their integrity and offer fair pricing to honest policyholders.
"Understanding various types of fraud is crucial for developing effective detection systems that adapt to dynamic threats."
Each type of fraud presents unique challenges; therefore, a comprehensive approach to fraud detection must incorporate specific strategies for identifying, investigating, and resolving these issues. By enhancing their capabilities in this area, organizations not only safeguard their resources but also their reputation—ensuring a sustainable and trustworthy environment for their customers.
Core Components of Fraud Detection Systems
Understanding the core components of fraud detection systems is essential for effectively mitigating fraudulent activities. These components play a significant role in the detection and prevention of fraud, ensuring systems are robust and reliable. The integration of technology into these components enhances the efficiency of analyzing vast amounts of data while minimizing the risk of oversight.
Data Collection
Data collection forms the foundation of any fraud detection system. It involves gathering relevant information from multiple sources such as transaction histories, customer profiles, and behavioral patterns. The quality and quantity of data collected directly affect the accuracy of fraud detection.
Some important aspects of data collection include:
- Diverse Sources: Integrating data from various sources, such as databases, APIs, and even social media platforms, can provide a more holistic view of potential fraudulent activities. This cross-referencing helps in enhancing the detection process.
- Real-Time Data: Collecting data in real-time allows systems to identify anomalies as they occur. Quick identification can limit potential damage from fraudulent activities.
- Historical Data: Analyzing past data patterns helps in recognizing trends and understanding typical behavior, thus helping to flag any deviations promptly.
Ensuring accurate and comprehensive data collection is critical as it lays the groundwork for analysis and decision-making in fraud detection systems.
Data Analysis Techniques
Once data is collected, the next step is analyzing it for suspicious patterns that could indicate fraud. Various techniques are employed in this stage, including:
- Statistical Analysis: Basic statistical methods help identify abnormal patterns. For example, if transaction amounts deviate significantly from an individual's historical spending habits, it raises flags.
- Machine Learning Models: Advanced algorithms are used to learn from past data and improve detection over time. Supervised and unsupervised learning methods can refine the accuracy of identifying fraudulent transactions.
- Natural Language Processing: This technique can analyze customer interactions, reviews, and complaints for potential warning signs of fraud or abusive behavior.
Implementing robust data analysis enhances the system's ability to detect fraud quickly, reducing false negatives and improving overall success rates.
Reporting and Escalation
Effective reporting and escalation processes are vital following the identification of potential fraud. This component ensures that detected anomalies are communicated and investigated properly. Key elements include:
- Automated Alerts: Systems should generate automated alerts based on predefined criteria. This enables quicker responses to suspicious activities.
- Incident Management: A structured approach to handle reported suspicious activities is necessary. Team members should know how to escalate findings for further investigation effectively.
- Documentation: Maintaining detailed records of detected incidents, including responses and resolutions, helps improve future detection capabilities and serve as references for legal processes if needed.
The reporting and escalation phase is a critical intersection where technology meets human oversight. Efficient processes improve response capabilities and help organizations adjust their fraud detection strategies.
Technologies Used in Fraud Detection
In the ever-evolving landscape of fraud management, the underlying technologies play a pivotal role. When discussing fraud detection systems, technology is not merely a component; it is the foundation upon which effective detection operates. This section explores the different technologies employed to combat fraud and their specific benefits in the modern context.
Machine Learning Algorithms
Machine learning algorithms have transformed the way fraud is detected and managed. These algorithms can analyze historical data to identify patterns that indicate fraudulent behavior. By employing various techniques, organizations can enhance their fraud detection efficiency significantly.
Key benefits of using machine learning algorithms in fraud detection include:
- Adaptive Learning: These algorithms learn continuously from new data, improving their accuracy over time.
- Pattern Recognition: They can recognize subtle patterns and anomalies that manual systems may overlook.
- Automation: Automation of data processing speeds up the identification of fraudulent transactions, which is crucial in real-time environments.
In practice, institutions like banks utilize supervised learning methods to train models on labeled datasets, which consist of flagged fraudulent cases. This enables the model to predict potential fraud in future transactions effectively.
Neural Networks
Neural networks, a class of machine learning algorithms inspired by the human brain, have gained popularity in fraud detection. They are particularly effective due to their ability to capture complex relationships in data.
Benefits of neural networks in this context include:
- Accuracy: They improve prediction rates for fraud detection when handling vast amounts of data.
- Deep Learning Capabilities: Neural networks can handle multi-layered data inputs, resulting in a more nuanced understanding of transactional anomalies.
- Scalability: As organizations grow, neural networks can scale effortlessly, adapting to larger datasets without loss of performance.
By analyzing transactions in real time, neural networks can flag unusual activities instantaneously, thereby allowing businesses to react swiftly to potential threats.
Rule-Based Systems
Rule-based systems form another essential layer in fraud detection technologies. These systems operate on sets of predefined rules created based on expert knowledge. They are often used in tandem with machine learning methods to provide a systematic approach to fraud management.
Some characteristics of rule-based systems include:
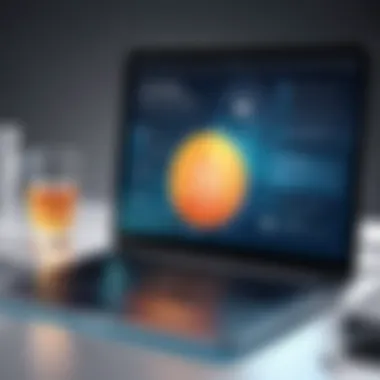
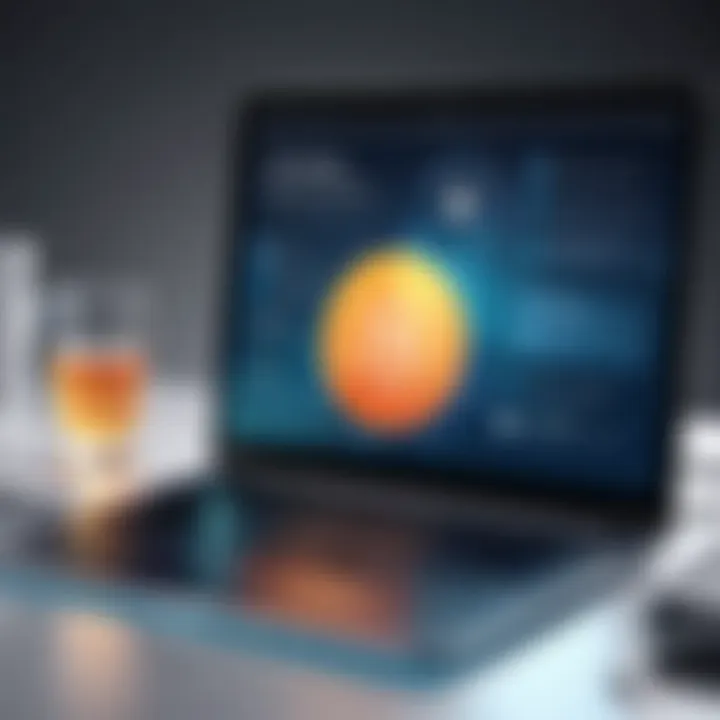
- Simplicity: Easy to implement and understand, making them suitable for organizations with limited technological expertise.
- Quick Deployment: These systems can be deployed relatively quickly, allowing for immediate fraud protection.
- Customization: Organizations can tailor rules to address specific risks pertinent to their operations.
However, it is important to highlight the limitations. Rule-based systems may struggle with novel fraud tactics since they rely on existing rules. Thus, it is advisable for businesses to consider a hybrid approach, integrating rule-based systems with more dynamic technologies like machine learning for optimal effectiveness.
"The right technology stack is crucial for building resilient fraud detection systems that can evolve as threats change."
Machine Learning in Fraud Detection
Machine learning is a pivotal element in the realm of fraud detection systems. Its ability to analyze vast amounts of data efficiently makes it indispensable in identifying fraudulent activities in real-time. The adoption of machine learning can enhance fraud detection processes by enabling systems to learn from data patterns, thus improving their predictive accuracy. Companies that harness machine learning can respond to threats more quickly, minimizing potential losses and maintaining trust with their clients.
The benefits of integrating machine learning into fraud detection are numerous. For starters, it allows for adaptive learning. As new fraud tactics emerge, machine learning algorithms can update themselves and improve their detection strategies accordingly. This ensures that systems remain relevant and effective amid constantly changing threats. Additionally, it reduces the burden on human analysts by automating routine monitoring tasks, allowing them to focus on more complex investigations.
It’s also crucial to consider the challenges that come with implementing machine learning. Ensuring data quality is essential. Inaccurate or irrelevant data can lead to misleading outcomes and decreased effectiveness. Furthermore, businesses must address ethical considerations, especially concerning data privacy. Transparency in how data is used and processed is vital to build trust with users.
Supervised Learning
Supervised learning is a fundamental method within machine learning utilized in fraud detection. In this approach, algorithms learn from labeled datasets, which helps them identify patterns linked to fraudulent behavior. Through this process, historical data that indicates fraud or legitimate transactions become the basis for training the system.
Key advantages of supervised learning include:
- High predictive accuracy: With sufficient labeled examples, algorithms can achieve impressive accuracy in identifying fraudulent activity.
- Clear feedback mechanism: Analysts can fine-tune the models based on their performance, leading to continuous improvement.
However, the effectiveness of supervised learning is contingent on the quality and quantity of labeled data available. Real-world applications often face the challenge of data scarcity, particularly for rare events like fraud. Ensuring a diverse and representative dataset becomes vital to train robust models that can generalize well to unseen instances.
Unsupervised Learning
Unsupervised learning offers a different perspective in the fraud detection landscape. Unlike supervised learning, this method does not rely on labeled data. Instead, it discovers patterns and anomalies within the data through clustering or association techniques. This can be particularly useful for identifying new or emerging fraud schemes that have not been previously documented.
The benefits of unsupervised learning include:
- Ability to detect unknown fraud types: Since the system is not limited by historical data, it can identify new fraudulent patterns that have not been seen before.
- Reduced reliance on labeled data: Organizations can leverage vast amounts of unlabeled data to train models, which is often more readily available.
However, challenges remain with unsupervised learning. Determining what constitutes an anomaly can be subjective, leading to high rates of false positives. This can overwhelm analysts and cause them to miss critical fraud signals. More research is necessary to refine these algorithms and minimize false identifications.
Challenges in Implementing Fraud Detection Systems
Fraud detection systems play a vital role in maintaining the integrity of various industries. However, implementing these systems is not without its challenges. Understanding these challenges is crucial for businesses and IT professionals, as they can significantly impact the effectiveness of fraud detection solutions. Each challenge presents unique considerations that necessitate careful planning and execution. Key challenges include false positives, data privacy issues, and the need to adapt to constantly evolving fraud tactics.
False Positives
False positives occur when legitimate transactions are incorrectly flagged as fraudulent. This can create significant issues for both consumers and businesses. The implications include customer dissatisfaction, resource wastage, and the potential for monetary loss.
Managing false positives is a balancing act. On one hand, fraud detection systems need to be sensitive enough to catch fraudulent activities. On the other hand, they should minimize the rate at which they flag legitimate transactions. One strategy is to continually refine the algorithms used in detection systems, allowing for better accuracy over time.
Organizations can also invest in customer education. Helping customers understand the reason for alerts can mitigate frustration. Additionally, using layered security measures can help differentiate between genuine and fraudulent activity.
Data Privacy Issues
Data privacy is a major concern when implementing fraud detection systems. These systems often require vast amounts of sensitive personal data to function effectively. Balancing the need for data collection against privacy regulations, such as GDPR or HIPAA, is essential. Organizations must ensure compliance with these regulations to avoid severe penalties.
Moreover, there is a risk of data breaches. Protecting data is not just an ethical obligation but also a legal requirement. Implementing robust security measures, including encryption and access controls, is a critical step. Organizations should foster a culture of data security awareness and regular audits of systems to ensure they are up to date with the latest practices.
Constantly Evolving Fraud Tactics
Fraud tactics are not static; they evolve continuously. As fraudsters find new methods, fraud detection systems must adapt accordingly. This poses a significant challenge, as organizations need to stay ahead of these tactics to effectively mitigate risks.
Regular updates to machine learning algorithms and rules-based systems are necessary to reflect the latest trends in fraud. Collaboration with industry counterparts can also provide insights into emerging threats. Joining forums or groups focused on fraud prevention can enhance knowledge sharing and resource pooling.
"In the battle against fraud, adaptability is as vital as the initial detection strategy."
Enhancing the awareness of employees through training programs can also help in detecting fraud attempts that may not have been anticipated by the systems in place. It’s crucial for organizations to foster an environment that supports continuous learning and adapting to new fraud landscapes.
Industry Applications of Fraud Detection Systems
Fraud detection systems play a vital role across various industries. Their application is essential for mitigating risks associated with fraudulent activities, which can have severe financial and reputational consequences. As organizations increasingly depend on digital transactions and data exchange, the need for robust fraud detection solutions becomes more evident. Each industry faces unique challenge and operational contexts, which influences how these systems are implemented. Understanding the specific applications of fraud detection in banking, e-commerce, and telecommunications provides a clearer view of their significance.
Banking and Financial Services
In the banking sector, fraud detection systems are crucial for protecting assets and maintaining customer trust. Financial institutions deal with numerous transactions daily, making them attractive targets for fraudsters. These systems analyze patterns in transaction data to identify unusual behaviors that may indicate fraud. For example, a sudden high-value transfer to a foreign account might trigger an alert.
The use of machine learning algorithms enhances these systems by continually improving their accuracy. The constant evolution of fraud tactics necessitates that fraud detection systems adapt quickly to new methods. In addition, regulatory requirements demand transparent and efficient fraud detection processes. This includes tracking suspicious activities and reporting them in compliance with government regulations. Hence, effective fraud detection is not just an operational necessity; it is a regulatory imperative in banking.
E-Commerce
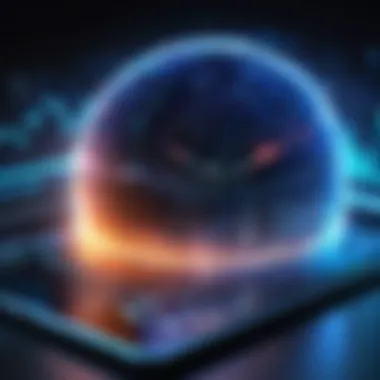
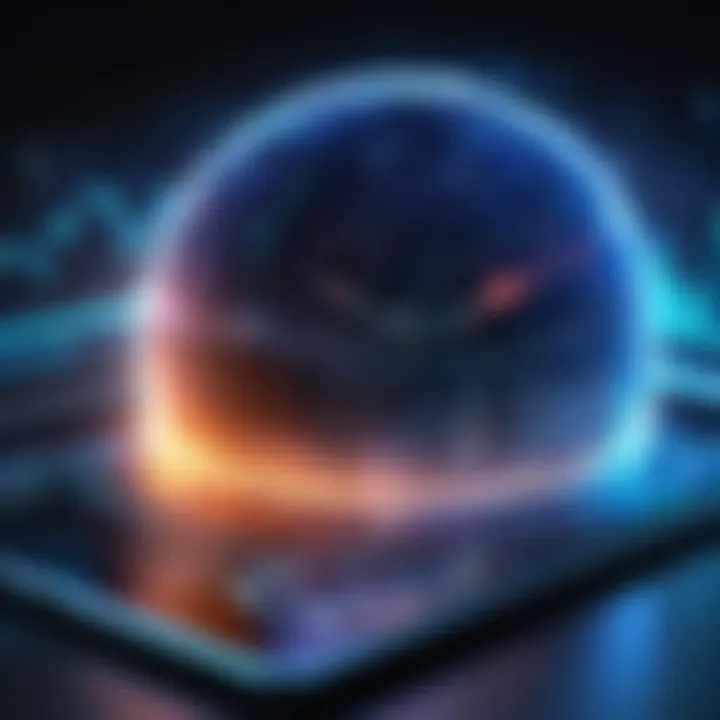
The rise of e-commerce also underscores the need for effective fraud detection systems. Online retail places both businesses and consumers at risk due to the nature of internet transactions. Issues such as credit card fraud, account takeovers, and fraudulent chargebacks are prevalent in this space. The anonymity of online purchases can make it difficult for retailers to verify identities, thus increasing the potential for fraudulent activities.
Fraud detection solutions for e-commerce often employ real-time monitoring and behavior analysis. For instance, if an account suddenly shows a high volume of purchases after being inactive for weeks, it may signal a security threat. This proactive approach helps merchants reduce their losses and protect their customers. The ability to assess transactions quickly is vital. Delayed detection can not only lead to financial losses but also damage customer relationships and undermine brand reputation.
Telecommunications
In the telecommunications industry, fraud can take many shapes, from subscription fraud to SIM card cloning. These risks can lead to substantial financial losses and negatively impact service quality. Fraud detection systems in this sector are essential for monitoring usage patterns and detecting anomalies. For example, if a new customer activates multiple lines in a short period, it may raise suspicion.
The implementation of automated fraud detection is particularly beneficial in telecommunications. This sector often requires handling massive amounts of data, and manual detection methods are simply not scalable. Here, advanced analytics and predictive modeling come into play, allowing companies to foresee potential fraudulent activities before they occur. Furthermore, continuous learning from new data ensures that these systems remain effective against emerging fraud tactics.
"The application of fraud detection systems must align with the unique requirements of each industry to be genuinely effective."
These industry-specific fraud detection solutions are not only about preventing loss but are integral in building a better trust framework between customers and service providers. As the landscape of fraud continues to evolve, so will the technologies and methodologies employed to combat these threats. Understanding these applications is vital for IT professionals and businesses aiming to implement robust fraud detection strategies.
Measuring Effectiveness of Fraud Detection Systems
Evaluating the effectiveness of fraud detection systems is critical for any organization relying on such technology. As fraud tactics become more sophisticated and prevalent, the ability to measure performance is essential to adapt and refine detection processes. This section delves into the methodologies employed to gauge effectiveness, the benefits of such evaluations, and key considerations that organizations must acknowledge when measuring performance.
Key Performance Indicators
Key Performance Indicators (KPIs) serve as the backbone for assessing the effectiveness of fraud detection systems. They provide quantitative data that organizations can use to manage and optimize their fraud detection strategies. Some important KPIs include:
- Detection Rate: This metric shows the percentage of fraudulent activities identified by the system compared to total fraudulent activities that occurred. A higher detection rate indicates a more effective system.
- False Positive Rate: This KPI focuses on the number of legitimate transactions mistakenly flagged as fraudulent. A low false positive rate signals that the system is working well, reducing unnecessary disruptions for genuine customers.
- Response Time: The time taken to react to potential fraud cases is critical. A quicker response can significantly mitigate financial losses.
- Cost Per Detection: Analyzing costs associated with identifying fraud can help organizations understand whether their investment in fraud detection systems is reasonable and effective.
"Without proper KPIs, it's difficult to improve fraud detection systems effectively."
Performance Benchmarks
Setting performance benchmarks is an integral part of measuring the effectiveness of fraud detection systems. Benchmarks provide a standard or reference point against which performance can be compared. This aspect can prove useful in several ways:
- Evaluating Progress: Organizations can track improvements in fraud detection systems over time by regularly comparing current performance metrics against these established benchmarks.
- Benchmarking Against Peers: Companies can compare their KPIs with industry standards or competitors. This comparison helps identify areas for improvement and set realistic targets.
- Informed Decision Making: Benchmarks provide valuable context for decision-makers. By understanding what is considered acceptable performance in the industry, organizations can make informed adjustments to their systems.
To implement effective performance benchmarking:
- Establish clear and relevant KPIs,
- Regularly review and adjust benchmarks based on evolving fraud tactics and industry changes,
- Collaborate with other organizations or industry groups to develop comprehensive benchmarks.
By effectively measuring and benchmarking the performance of fraud detection systems, organizations can manage risk more efficiently, allocate resources appropriately, and continuously improve their protective measures against fraud.
Future Trends in Fraud Detection
The landscape of fraud detection is rapidly changing. Technologies like artificial intelligence (AI) and advanced automation increasingly shape the future of this vital area. Embracing these developments is key for businesses aiming to protect themselves from emerging threats. The upcoming trends promise enhanced coverage and detection performance, allowing for adaptive and dynamic responses to the ever-evolving methods utilized by fraudsters.
Integration of AI and Automation
The integration of AI and automation in fraud detection systems marks a significant leap forward. With AI, patterns buried in vast datasets can surface to reveal suspicious activities that human investigators might overlook. Unlike traditional systems, AI enhances the ability to adapt and learn from new data. This adaptability means fraud detection systems can evolve in response to changes in fraud tactics.
Automation complements this growth by streamlining the processing of detection results. Automatic routine checks free up resources for more strategic initiatives, allowing organizations to deploy human expertise where it is most needed. Automation also reduces response times, making it possible for businesses to act quickly on potential threats, thus minimizing risk. Organizations adopting AI and automation often report noticeably improved efficiency.
"Adopting AI and automation in fraud detection can transform how organizations prevent and respond to fraud. Efficiency and adaptability are no longer optional."
Real-Time Monitoring Solutions
Real-time monitoring represents another forward-thinking approach in fraud detection. Instead of relying on periodic assessments, real-time solutions continuously analyze transactions and data streams. This continuous analysis helps identify anomalies instantaneously, allowing teams to investigate and address issues as they arise.
By implementing real-time monitoring solutions, organizations gain several advantages. First, they increase their ability to detect fraudulent transactions before they can grow into significant losses. Second, they offer a more engaging user experience. Users can interact with systems that respond instantly to their activities, enhancing satisfaction and trust.
Some key benefits include:
- Immediate Detection: Catching fraudulent activities as they happen reduces the impact.
- Enhanced Customer Trust: Customers feel more secure with immediate responses.
- Data-Driven Insights: Continuous data feeds allow businesses to draw better insights over time.
With the proper technology and commitment to improvement, organizations can make substantial strides in counteracting fraudulent threats. The future of fraud detection looks promising, combining the power of AI, automation, and continuous analysis.
Closure
The conclusion serves as a crucial capstone to our exploration of fraud detection systems. It summarizes the complexities and multifaceted nature of fraud detection methodologies, technologies, and practices discussed throughout the article. In an age where digital transactions are increasingly prevalent, the security frameworks employed by various industries must evolve concurrently. Understanding the key insights allows organizations to adapt and strengthen their defenses against fraudulent activities.
Recap of Key Insights
In this section, we can distill the main points covered regarding fraud detection systems:
- Diverse Types of Fraud: Our discussion highlighted various fraud types, including financial fraud, identity theft, and insurance fraud, each requiring specific detection strategies.
- Advanced Technologies: The role of technologies like machine learning and neural networks in detecting anomalies and predicting fraudulent behavior cannot be understated.
- Challenges: We underscored the significance of addressing false positives and data privacy issues, which are common hurdles faced by organizations.
- Industry Applications: Different sectors, such as banking and e-commerce, demonstrate the versatility and necessity of robust fraud detection systems.
The synthesis of these insights equips readers with the knowledge to navigate the evolving landscape of fraud detection.
The Role of Continuous Improvement
Continuous improvement is essential in the realm of fraud detection. As fraud tactics grow more sophisticated, systems must adapt and evolve. Organizations need to regularly evaluate and refine their detection methods, algorithms, and technologies. They should incorporate feedback mechanisms and stay informed on emerging trends and threats.
Employing continuous learning practices not only enhances the effectiveness of fraud detection systems but also fosters a proactive security culture within organizations. This adaptability can provide a significant competitive advantage in safeguarding assets and ensuring customer trust.
To sum up, the importance of ongoing enhancement in fraud detection systems cannot be overlooked. It underlines the commitment to security and delivering value to stakeholders in an ever-changing environment.