Understanding Predictive Analytics in AI for Better Decisions
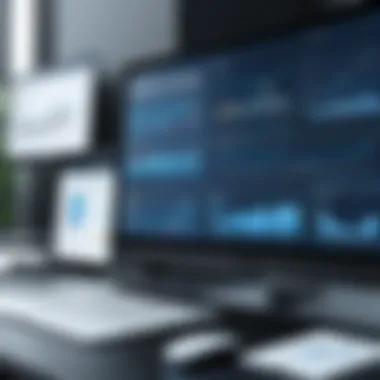
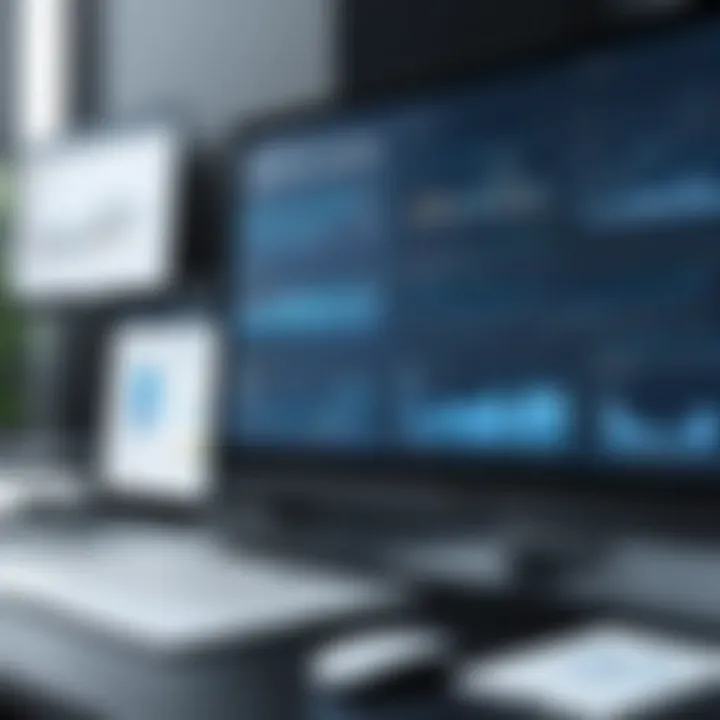
Intro
Predictive analytics is a crucial aspect of artificial intelligence that enables organizations to harness data for better decision-making. This field draws from historical data to forecast potential outcomes, allowing professionals to strategize effectively. The emphasis on integrating predictive analytics within AI has grown, leading businesses to explore its applications across various industries.
The significance of grasping predictive analytics cannot be overstated. With the rise of big data, the demand for tools that can interpret vast quantities of information has increased. By utilizing predictive models, companies can uncover needs they might not have realized they had, which directly influences their operational strategies. The industry landscape is quickly evolving, and staying informed can make the difference between gaining a competitive edge and falling behind.
The core components of predictive analytics involve algorithms and machine learning techniques that analyze data trends. These methodologies allow for recognizing patterns, making predictions, and ultimately guiding decisions based on empirical evidence. As such, delving into how predictive analytics operates within the AI framework is essential for both IT professionals and business leaders seeking to enhance their strategic capabilities in an increasingly data-driven world.
Prologue to Predictive Analytics
Predictive analytics is increasingly crucial in today’s fast-paced data-driven world. Organizations across various industries are turning to this powerful tool to inform decision-making and drive strategic initiatives. By leveraging statistical algorithms and machine learning techniques, predictive analytics helps businesses anticipate future outcomes based on historical data. The ability to make predictions not only enhances efficiency but also improves competitiveness in the marketplace. Understanding this concept is essential for IT and software professionals, as well as businesses wanting to harness the advantages it offers.
Defining Predictive Analytics
Predictive analytics, at its core, involves using data, statistical algorithms, and machine learning to identify the likelihood of future outcomes. It combines historical data with sophisticated analytical techniques to provide insights that inform decision-making. This process often includes data input from various sources, such as customer data, sales trends, and operational metrics.
However, defining predictive analytics is not limited to its technical aspects. It also encompasses its practical applications, such as forecasting sales, assessing risks, and optimizing marketing strategies. Furthermore, the significance of predictive analytics lies in its ability to convert complex data into actionable intelligence. Such intelligence can guide companies in making informed decisions, thus enhancing their overall performance.
Historical Context and Evolution
The evolution of predictive analytics can be traced back several decades, with its roots in statistics and data mining. Initially, businesses relied on basic statistical techniques to forecast outcomes. However, advancements in technology have revolutionized this field.
In the early days, predictive models were primarily driven by linear regression analysis. The introduction of more sophisticated methods, such as decision trees and neural networks, has since expanded the potential applications of predictive analytics.
The rise of big data in the 21st century further transformed the landscape. Organizations began to collect vast amounts of data from diverse sources, necessitating the development of more advanced analytics techniques. As computing power has improved, so have the algorithms, resulting in more accurate predictive models. Today, predictive analytics plays a pivotal role in customer relationship management, cybersecurity, healthcare, and beyond.
Understanding the historical context of predictive analytics enables professionals to appreciate its evolution and effectively apply its principles in various domains.
The Role of Artificial Intelligence in Predictive Analytics
Artificial Intelligence (AI) plays a pivotal role in the realm of predictive analytics. This section aims to present the intricacies of how AI enhances predictive models to create actionable insights. The importance can be largely attributed to the sophistication and efficiency that AI introduces. These technologies help organizations analyze vast amounts of data, identifying patterns that can significantly inform strategic decisions.
AI's ability to learn from data, known as machine learning, is particularly relevant. It adapts algorithms based on new inputs, improving the accuracy of predictions. In sectors like finance and healthcare, where decisions must be informed by intricate data, AI's contributions are invaluable. By integrating predictive capabilities with AI, organizations can elevate their forecasting accuracy and drive informed decisions.
Understanding AI Fundamentals
To grasp AI's role in predictive analytics, one must first understand the fundamentals of AI itself. At its core, AI refers to computer systems designed to perform tasks that typically require human intelligence. Key definitions and concepts include:
- Machine Learning: A subset of AI where algorithms learn patterns from data.
- Natural Language Processing: Enabling machines to understand and interpret human language.
- Deep Learning: A layer of machine learning that uses neural networks to model complex patterns.
These foundational elements enable AI to analyze data effectively. In predictive analytics, AI algorithms sift through historical data, employing statistical techniques to generate forecasts.
Synergy Between AI and Predictive Analytics
The intersection of AI and predictive analytics creates a powerful synergy, enhancing decision-making across various industries. This combination offers several critical benefits:
- Increased Efficiency: AI streamlines data processing, resulting in faster and more accurate predictions.
- Real-Time Analysis: Businesses can access up-to-date insights and respond to changes promptly, an advantage in fast-paced markets.
- Personalization: AI systems can tailor predictions based on individual user behavior, increasing relevance and effectiveness.
However, this synergy is not without its challenges. For instance, the complexity of AI models can lead to difficulties in interpretability. Stakeholders need to balance the desire for accuracy with the need to understand how predictions are made.
"The melding of AI with predictive analytics is transforming decision-making, but it requires careful navigation of its complexities."
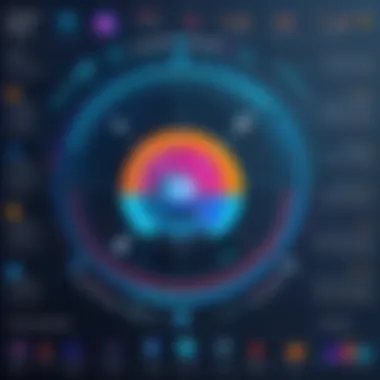
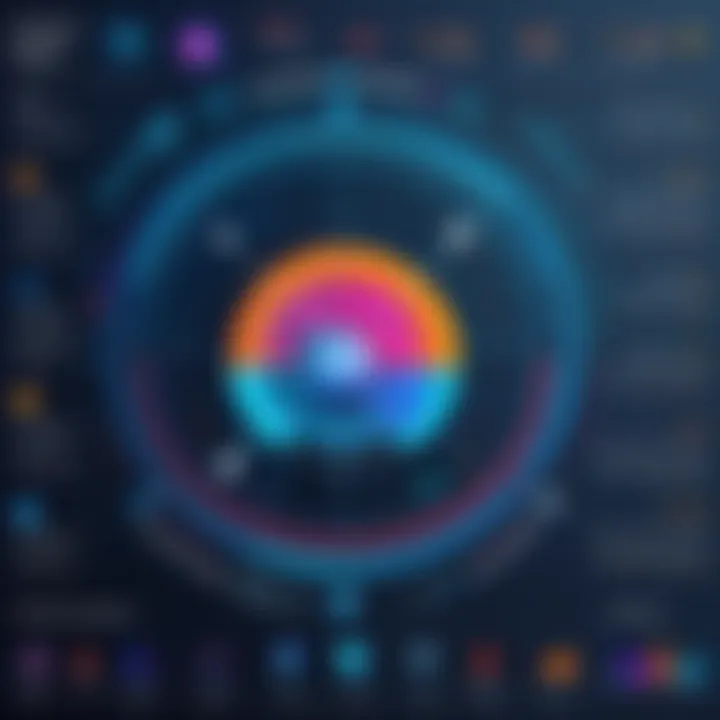
In summary, the role of AI in predictive analytics is crucial. It amplifies analytical capabilities, but organizations must maintain awareness of the associated risks and challenges.
Key Components of Predictive Analytics
The efficacy of predictive analytics relies heavily on its core components. These components shape how data is utilized and govern the quality of insights derived. Among these, data collection and management, alongside the algorithms and modeling techniques, play crucial roles in achieving precise predictions. The ultimate goal of predictive analytics is enhancing decision-making, thereby delivering significant benefits to businesses and practitioners alike.
Data Collection and Management
Data collection is the foundational step in predictive analytics. It involves gathering relevant and timely data from various sources. The quality of this data dictates the robustness of any predictive model. In this sense, businesses must prioritize accuracy, completeness, and relevance.
Once data is collected, management becomes crucial. Proper management strategies ensure that data remains organized, accessible, and usable. Data may come from disparate systems, so integration tools are often necessary. Adopting a data governance framework can enhance accountability, ensuring that the data's use aligns with organizational ethics and standards.
Effective data management also involves cleaning and preprocessing. Removing duplicates, filling in missing values, and standardizing formats plays a vital role in making data ready for analysis. These steps help reduce noise and enhance the model's performance.
Algorithms and Modeling Techniques
Algorithms and modeling techniques are the engine behind predictive analytics. They analyze historical data to identify patterns and predict future outcomes. Various algorithms offer unique capabilities, allowing analysts to select the ideal fit for their specific needs.
Regression Analysis
Regression analysis is a statistical method widely utilized in predictive analytics. Its primary function is to establish a relationship between dependent and independent variables. This method allows practitioners to forecast outcomes based on input parameters. A key characteristic of regression analysis is its simplicity and interpretability, making it a popular choice among data analysts.
Advantages of regression analysis include:
- Straightforward implementation.
- Clear visualizations of relationships.
- Effective for continuous outcome prediction.
However, disadvantages include its assumption of linearity, which can limit applications when data relationships are fundamentally nonlinear.
Decision Trees
Decision trees serve as another valuable tool in predictive analytics. They create a model in the form of a tree structure, mapping out decisions and their likely consequences. This model effectively handles both categorical and continuous variables, making it versatile.
A significant advantage of decision trees is their ease of interpretation. Stakeholders can grasp the decision-making process quickly. However, they are prone to overfitting, where a model captures noise instead of the underlying pattern, leading to poor predictions on new data.
Neural Networks
Neural networks stand out for their capability to model complex relationships in data. They simulate human brain function, learning from vast amounts of information. Neural networks excel in tasks such as image recognition and natural language processing, adding to their appeal in various industries.
The primary strength of neural networks lies in their ability to handle vast datasets with multiple variables. This makes them particularly beneficial in intricate, non-linear prediction scenarios. Yet, they can be challenging to interpret compared to other models. This lack of transparency can be a significant drawback, especially when stakeholders require clear explanations of decisions based on these models.
"In predictive analytics, knowing how to collect, manage, and analyze data is crucial to deriving meaningful insights."
This knowledge not only aids in harnessing the potential of predictive analytics but also aligns with ethical practices in data handling.
Applications Across Industries
The application of predictive analytics in various sectors has significantly reshaped decision-making processes. Throughout different industries, organizations harness the insights derived from data patterns to make informed decisions. This not only leads to improved performance but also enhances customer satisfaction and operational efficiency. Understanding these applications allows IT professionals, software engineers, and business leaders to leverage predictive analytics effectively.
Healthcare: Predicting Patient Outcomes
In healthcare, predictive analytics plays a crucial role in forecasting patient outcomes. By analyzing historical data, medical practitioners can identify trends and predict which patients may be at risk of various conditions. For instance, hospitals use algorithms to analyze patient records, lab results, and treatment responses to determine the likelihood of readmission. This insight enables healthcare providers to take preventative actions, optimizing patient care.
Benefits:
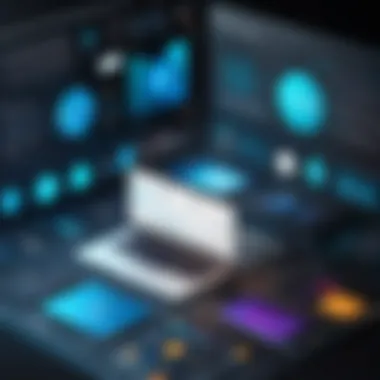
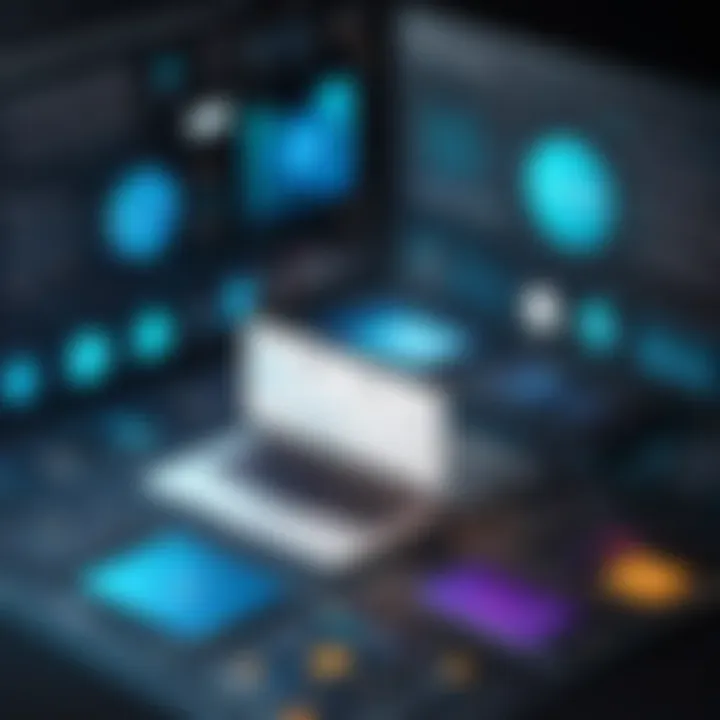
- Reduces preventable complications
- Improves patient management strategies
- Enhances allocation of medical resources
Moreover, predictive models improve the timeliness of patient interventions. Tools like IBM Watson Health utilize extensive datasets to provide recommendations for personalized treatments, making care more efficient.
Finance: Risk Assessment and Fraud Detection
In the finance sector, predictive analytics is integral for managing risks and detecting fraud. Financial institutions deploy various algorithms that analyze transactions in real-time, identifying anomalous behaviors. These systems help in flagging unusual activity, enabling swift responses to potential threats.
Benefits:
- Enhances the security of transactions
- Improves risk management frameworks
- Reduces financial losses due to fraud
For example, PayPal utilizes predictive analytics to monitor transaction patterns and assess the probability that a transaction may be fraudulent. This proactive approach has significantly reduced fraud cases across platforms.
Retail: Enhancing Customer Experience
Retailers employ predictive analytics to refine customer experiences. By analyzing purchasing behavior, retailers can forecast demand for products, optimizing inventory management. Predictive models can predict customer preferences and tailor marketing campaigns accordingly.
Benefits:
- Increases customer satisfaction
- Improves inventory turnover rates
- Maximizes sales opportunities
Companies like Amazon use predictive analytics to recommend products based on user browsing history and purchase patterns. This enhances customer engagement and drives sales. Retailers that adapt their strategies accordingly can experience a substantial competitive edge.
Manufacturing: Predictive Maintenance
In manufacturing, predictive analytics significantly influences maintenance strategies. By monitoring machine performance data, companies can predict equipment failures before they occur. This approach minimizes downtime and prolongs asset life.
Benefits:
- Reduces maintenance costs
- Enhances operational efficiency
- Improves safety standards
For instance, General Electric implements predictive analytics in its manufacturing plants, allowing for timely maintenance and reduced operational disruptions. By anticipating equipment failures, manufacturers can effectively schedule repairs, leading to optimized production processes.
Challenges and Limitations
Understanding the challenges and limitations of predictive analytics in artificial intelligence is crucial for IT and software professionals, as well as businesses of all sizes. While predictive analytics offers tremendous advantages in enhancing decision-making, it is not without its hurdles. Overcoming these challenges is necessary to leverage the full potential of AI-driven predictive analytics. Key areas of concern include data quality, ethical implications, and the transparency of algorithms used in predictive models.
Data Quality and Availability
The foundation of predictive analytics rests on data quality and availability. Inaccurate or incomplete data can lead to misguided insights, which in turn negatively impact decision-making processes. Organizations must prioritize data governance practices that ensure data integrity and accessibility.
- Data collection: Having a robust data collection strategy is essential. It involves integrating various data sources like customer interactions, sensor data, and social media inputs.
- Data cleaning: Regularly cleansing the data to remove inaccuracies can improve the reliability of the models.
- Data management: Efficient management systems should be in place to handle data storage and retrieval seamlessly.
Limited data access can restrict an organization’s ability to make informed predictions. Organizations need to work closely with data providers and invest in building infrastructure that captures and manages high-quality data effectively.
Quality data is the backbone of all predictive analytics efforts. Without it, insights lose their credibility.
Ethical Considerations
Ethics in predictive analytics cannot be overlooked. As AI algorithms learn from data, they may inadvertently perpetuate biases present in that data. This can lead to outcomes that are unfair or discriminatory.
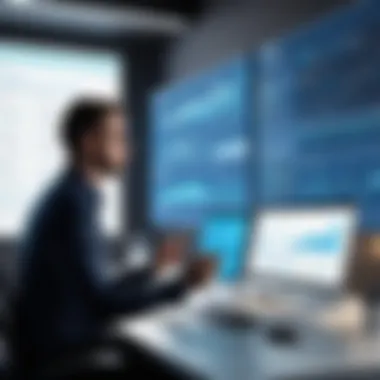
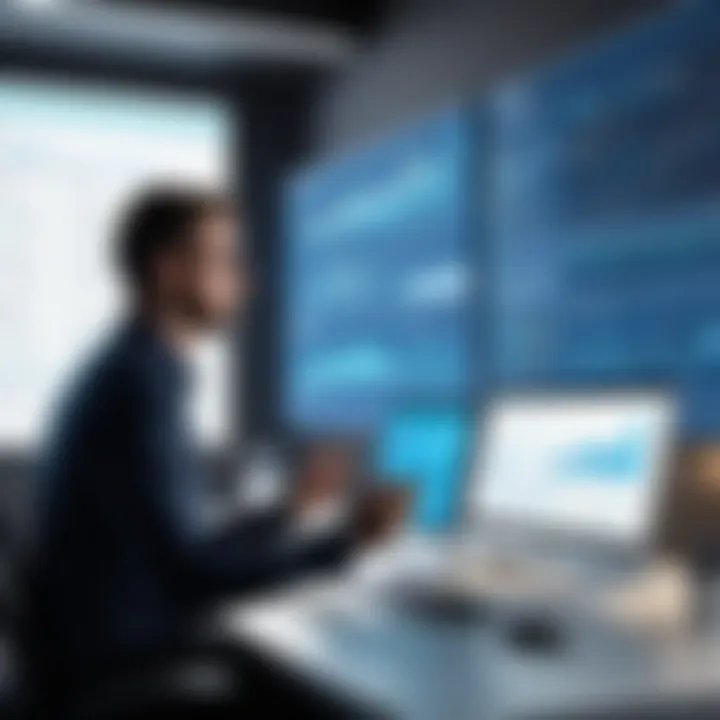
Organizations must address ethical implications by considering:
- Bias detection: Regular audits of models for bias are necessary. Organizations should implement procedures to detect and mitigate any form of biased data that can negatively affect decision-making.
- Transparency: Clear guidelines should be established around data usage and AI decision-making processes, ensuring stakeholders understand how decisions are derived.
- Accountability: Establishing accountability frameworks ensures that there is a mechanism to address any unethical consequences that arise from predictive analytics applications.
Interpretability and Transparency of Models
The interpretability of predictive models is vital for fostering trust among stakeholders. Complex algorithms, like neural networks, often function as black boxes. This means their decision-making processes are not easily understood, leading to skepticism about the outcomes produced.
- Model selection: Choosing simpler models when possible can enhance interpretability without sacrificing performance.
- Documentation: Providing comprehensive documentation of model functioning is essential. This includes details on the algorithms used, data inputs, and reasoning behind model choices.
- Visualization: Implementing visualization tools can help articulate predictions more clearly and allow users to grasp model outputs better.
Future Trends in Predictive Analytics
As predictive analytics continues to evolve, staying abreast of emerging trends is crucial for businesses and IT professionals. This section examines noteworthy developments that are shaping the future of predictive analytics. These trends not only enhance decision-making but also provide a competitive edge in various industries. Understanding these elements can help organizations strategize more effectively, ensuring they leverage data-driven insights optimally.
Advancements in Machine Learning Algorithms
Machine learning algorithms are central to predictive analytics. The advancements in these algorithms enhance their efficiency and accuracy in predicting outcomes. Innovations like ensemble learning and deep learning are gaining popularity. Ensemble learning, for instance, combines multiple models to improve predictions. This can lead to better reliability in forecasts as it mitigates the risk of overfitting, where the model performs well on training data but poorly on unseen data.
Deep learning allows models to learn from large datasets with complex structures. Techniques such as convolutional neural networks are particularly useful in image recognition and natural language processing tasks. Experimenting with these advanced techniques can yield significant insights that traditional modeling may overlook.
Moreover, the introduction of automated machine learning—automating the process of model selection and tuning—speeds up analysis and reduces the expertise barrier for organizations lacking data scientists. This democratization of machine learning fosters widespread application, thus enhancing decision-making capabilities across sectors.
Integration with Big Data Technologies
The interplay between predictive analytics and big data technologies is vital for realizing the full potential of data analysis. As data sources proliferate, organizations need robust frameworks to manage and analyze this information. Tools such as Apache Hadoop and Spark facilitate the handling of massive datasets efficiently. These technologies enable real-time data processing and analytics, which are essential for timely decision-making.
Integrating predictive analytics with big data capabilities allows organizations to uncover deeper insights. For instance, companies can analyze customer data streams in real time to tailor marketing strategies and optimize inventory management. Moreover, using cloud-based solutions enhances accessibility and scalability, making predictive analytics feasible for both small businesses and large enterprises.
The Impact of Quantum Computing
Quantum computing represents a paradigm shift, promising exponential increases in computational power. This advancement could revolutionize predictive analytics by enabling models to process vast amounts of data much faster than classical computers. Quantum algorithms may reveal patterns and insights that conventional methods cannot, thereby facilitating deeper predictive insights.
For example, quantum computing holds potential for complex simulations, such as predicting market behavior under various scenarios or modeling patient responses in healthcare. However, this technology is still in its infancy and poses challenges regarding implementation and standardization.
Investing in understanding and implementing these trends can significantly enhance predictive capabilities and drive a competitive advantage.
By keeping an eye on these advancements, businesses can ensure they are not only prepared for future challenges but also positioned to take advantage of upcoming opportunities in predictive analytics.
End
In the realm of modern business, the importance of predictive analytics powered by artificial intelligence cannot be overstated. This article has explored the various dimensions of this fusion, demonstrating how organizations can leverage these technologies to strengthen their decision-making processes. The key elements highlighted include the integration of advanced algorithms, comprehensive data management, and the significant role that AI plays in enhancing the accuracy of predictions.
Summarizing Key Insights
The exploration of predictive analytics through AI reveals several core insights:
- Enhanced Decision Making: Predictive analytics provides a data-driven foundation that can foresee trends and outcomes, giving leaders the necessary foresight to make informed decisions.
- Industry Applications: Various sectors, including healthcare, finance, retail, and manufacturing, benefit uniquely from the insights produced. This adaptive application underscores the versatility of predictive analytics.
- Challenges and Limitations: Addressing data quality, ethical considerations, and model interpretability is crucial. Stakeholders must navigate these challenges to fully utilize the potential of predictive analytics.
- Future of Predictive Analytics: As technology advances, particularly in machine learning and quantum computing, the capabilities of predictive analytics will continue to evolve, creating new opportunities for businesses willing to embrace these changes.
In summary, predictive analytics serves as a vital tool in the decision-making arsenal, offering strategic pathways while also requiring careful consideration of its implications.
Call to Action for Stakeholders
For stakeholders in technology and business, the time to act is now. Organizations are encouraged to:
- Invest in training and development in predictive analytics to keep pace with technological advancements.
- Prioritize the establishment of robust data management systems that ensure data quality and integrity.
- Foster collaboration across departments to facilitate the integration of predictive analytics into core decision-making practices.
- Engage with ethical frameworks that guide the use of AI in predictive analytics, ensuring transparency and accountability in model usage.
Adopting predictive analytics is not merely an option; it has become a strategic imperative. By taking proactive steps, enterprises can not only enhance their decision-making capabilities but also secure a competitive edge in an increasingly data-driven world.
"Embracing predictive analytics is essential for any organization wanting to adapt and thrive in today's competitive landscape."