Exploring Seven Types of Artificial Intelligence
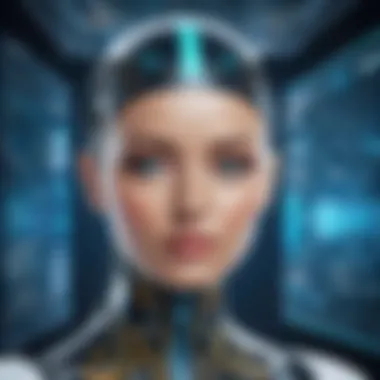
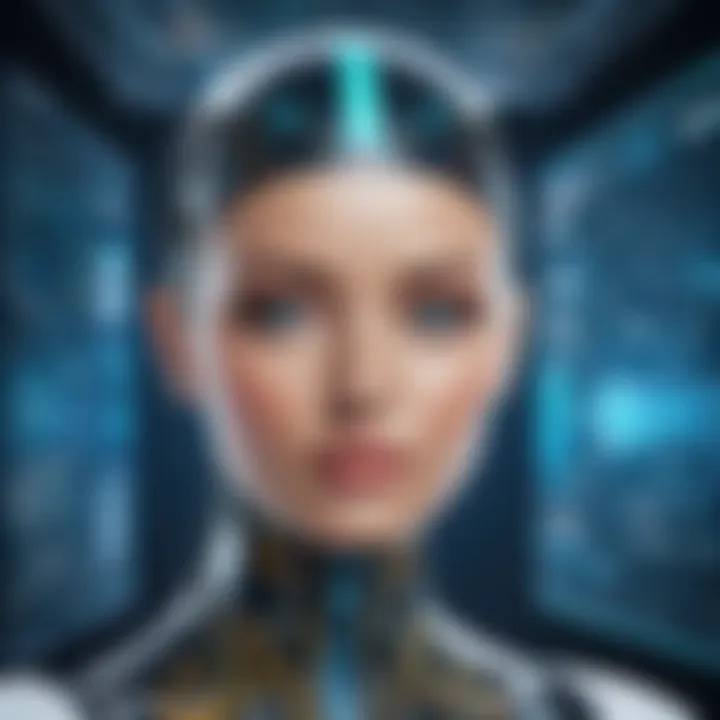
Intro
Artificial intelligence (AI) is transforming how businesses operate, affecting decisions and strategies across various sectors. Understanding the types of artificial intelligence can help businesses leverage its power effectively. This article aims to dissect the seven primary types of AI, providing insights into their unique characteristics and real-world applications.
With a clearer understanding, IT professionals, software developers, and business leaders can make informed decisions regarding the integration of AI into their operations. In today’s tech landscape, knowledge of AI categories is not just useful; it is essential for staying competitive and relevant.
Throughout this exploration, we will outline key features and advantages of each AI type, compare them with alternatives, and highlight the importance of AI in decision-making. This comprehensive guide will offer the necessary context to navigate the complexities of AI, ensuring that readers walk away with a well-rounded perspective.
Prolusion to Artificial Intelligence
Artificial Intelligence (AI) represents a transformative frontier in technology and industry. Its relevance in this discussion cannot be understated, as it is foundational to understanding different types of AI. By exploring AI, we bridge the gap between theoretical concepts and practical applications. This enables IT and software professionals, as well as business leaders, to make informed decisions as they navigate a rapidly evolving landscape.
Definition and Scope of AI
Artificial Intelligence refers to the simulation of human intelligence processes by machines, particularly computer systems. AI encompasses various types of technology designed to perform tasks that typically require human-like capabilities. These processes include learning, reasoning, and self-correction among others.
The scope of AI is broad. It can be segmented into numerous categories, reflecting its diverse applications across different sectors. From simple algorithms that respond to specific inputs to complex systems that learn and adapt, AI's definition extends into different domains of technology.
Key Elements of AI:
- Machine Learning: Systems that can learn from data.
- Natural Language Processing: Enable machines to understand and respond to human language.
- Robotics: Application of AI in the design and operation of robots.
This versatility is one of the primary reasons AI is a crucial topic today. Its adaptability allows businesses to innovate and improve operational efficiency.
Historical Context
The historical journey of artificial intelligence is significant to grasping its current state and future potential. AI's origins trace back to ancient myths and philosophical discussions about mechanical beings that could think. However, modern AI began developing in the mid-20th century.
The term ‘Artificial Intelligence’ was coined in 1956 during a conference at Dartmouth College. Here, researchers launched initiatives aimed at creating machines that could simulate human intelligence.
Since then, AI research has gone through various phases, including:
- The Initial Excitement (1950s - 1960s): Early successes led many to believe in the quick achievement of AI.
- The First AI Winter (1970s): Disappointment due to overhyped expectations led to funding cuts and reduced interest.
- Renewed Interest and Advancements (1980s - 1990s): Breakthroughs in algorithms and computing power renewed enthusiasm and investment.
- Current Resurgence (2000s - Present): Advances in data availability and computing capabilities have significantly accelerated AI developments, consolidating its importance in modern society.
Understanding this historical framework illuminates how AI evolved from theoretical concepts into a practical reality, making it an invaluable asset across industries.
"The evolution of AI reflects not only technological advancement but also our shifting perceptions of intelligence itself."
In-depth comprehension of these roots aids in understanding how we can shape the future of AI, both ethically and practically, as we delve into the various types of AI that follow in this article.
Type One: Reactive Machines
Reactive machines represent the most basic form of artificial intelligence. They operate with specific responses to particular stimuli. Their primary strength lies in their ability to analyze and respond to the current input without relying on past experiences. This capability makes them valuable in environments where decisions need to be made quickly and accurately without the need for complex learning. Understanding reactive machines is critical as they lay the groundwork for more advanced AI systems. By examining their characteristics and practical applications, professionals in the field can better appreciate the evolution of AI technologies.
Characteristics of Reactive Machines
Reactive machines are defined by their straightforward functionality. Unlike their more advanced counterparts, they do not possess any form of memory or past data enterprise. Here are key elements of reactive machines:
- Input-Response Mechanism: They respond to specific inputs with predefined actions.
- No Learning Capability: These machines do not learn from past experiences; every decision is based solely on current data.
- Real-Time Processing: They excel in real-time scenarios where speed and accuracy are paramount.
- Simplicity: Their designs are straightforward, enhancing reliability and performance in environments requiring quick decisions.
These characteristics underscore the reason why reactive machines are foundational within the broader framework of artificial intelligence.
Examples of Reactive Machines
Several notable examples of reactive machines demonstrate their utility and effectiveness. Some prominent instances include:
- IBM's Deep Blue: This chess-playing computer made headlines in 1997 when it defeated world champion Gary Kasparov. It calculated possible moves and responded optimally, highlighting its reactive capabilities.
- Google’s AlphaGo: While more advanced than Deep Blue in some respects, its initial versions operated reactively by evaluating current game scenarios and determining the best moves without experience-based learning.
- Spam Filters: These systems analyze incoming emails in real-time, identifying spam based solely on specific characteristics of the email, such as keywords or sender information, without reference to previous interactions.
"Reactive machines may not have the complexity of other AI types, but their reliability and speed in processing specific tasks make them invaluable."
Understanding reactive machines is essential for grasping the advance of AI technologies. They serve as a cornerstone that paves the way for more complex forms of artificial intelligence.
Type Two: Limited Memory
Limited Memory AI represents a significant step in the evolution of artificial intelligence. Unlike reactive machines, which function on predefined algorithms, Limited Memory AI can learn from historical data and improve its decision-making capabilities through experience. This adaptability is crucial for many applications in today's technology environment, making it a focal point for both research and practical implementations.
Understanding Limited Memory AI
In essence, Limited Memory AI is designed to draw on past experiences, making it capable of semi-autonomous decision-making. This type encompasses systems that can store information from previous interactions to make better predictions or suggestions in the future. The memory aspect is not permanent; rather, it temporarily retains information needed for a specific task. A practical example of this could be seen in autonomous vehicles, which must analyze and learn from various traffic scenarios to navigate safely.
Key aspects of Limited Memory AI include:
- Data storage: These systems retain data for a limited duration or until it is replaced by newer information.
- Learning ability: They utilize this data to enhance future performance, refining accuracy and effectiveness in their task.
- Dynamic behavior: The AI can adapt its actions based on new information, distinguishing it from static systems.
Applications of Limited Memory AI
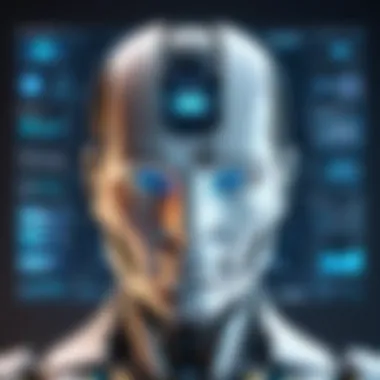
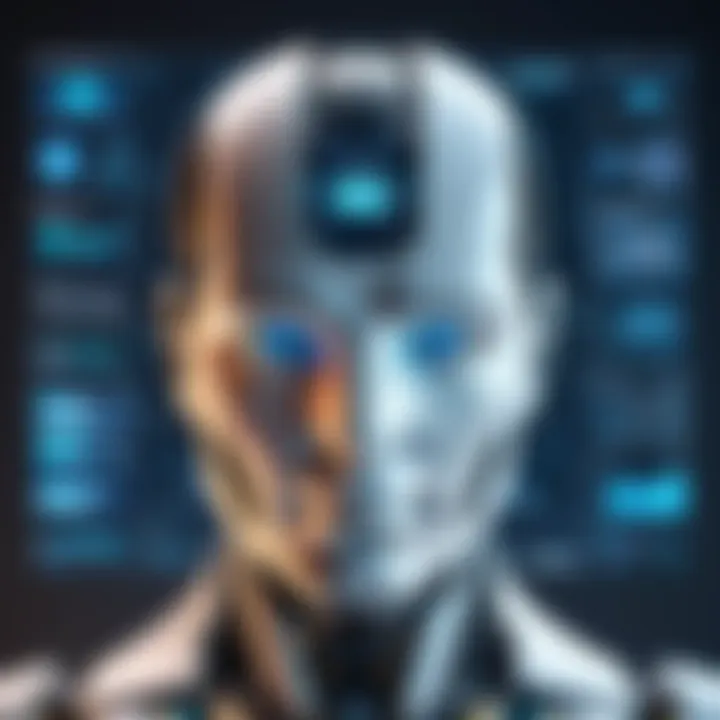
Limited Memory AI systems are already making waves across several sectors. Their ability to learn from data enables practical applications in various fields:
- Autonomous Vehicles: As mentioned earlier, companies like Tesla and Waymo employ Limited Memory AI to help vehicles make sense of their surroundings. This technology can predict possible outcomes by evaluating previous driving conditions.
- Customer Service: Chatbots utilize Limited Memory AI to improve customer interactions. By remembering past queries and preferences, they can provide personalized responses.
- Healthcare: Limited Memory AI can analyze patient data over time. This helps doctors with predicting outcomes or suggesting personalized treatment plans based on previous medical histories.
In this rapidly evolving landscape, the potential is immense. With ongoing advancements, Limited Memory AI might improve human-computer interaction, understanding complex datasets, and enhancing efficiency in daily tasks. This evolution will continue to encourage investment in technology solutions that embrace these intelligent systems.
"Limited Memory AI marks a pivotal transition towards more advanced, adaptive technologies that learn and evolve through experience."
In summary, the importance of Limited Memory AI cannot be overstated. As we increasingly rely on technology, understanding these systems becomes essential to navigate their applications and implications effectively, providing valuable insights into the future of intelligent systems.
Type Three: Theory of Mind
Concept of Theory of Mind in AI
Theory of Mind in artificial intelligence refers to the capability of a system to understand and simulate the mental states of others. This includes recognizing emotions, beliefs, intentions, and other cognitive functions that inform behavior. Unlike earlier types of AI, which react to stimuli without any comprehension of context, Theory of Mind AI aims to possess the ability to attribute mental states to both itself and others.
This type of AI seeks to include social understanding, making it particularly relevant in applications that require interaction with humans. It represents a significant leap toward human-like capabilities in machines, enabling them to engage more effectively in complex social environments. As AI systems gain the capability to recognize and adapt to emotional cues and intentions, their application in sectors like healthcare, education, and customer service could dramatically improves.
Progress and Challenges in Development
Development of Theory of Mind AI presents both remarkable advancements and considerable challenges. Researchers are making strides in machine learning and neural networks, exploring how systems can interpret human emotions using textual, vocal, and facial inputs. Recent models work towards understanding nuanced human behaviors and interactions, which are critical for implementing social AI functions.
However, several challenges remain. The complexity of human emotions and social interactions makes precise modeling incredibly tricky.
- Lack of data: Developing a Theory of Mind AI requires vast amounts of diverse data to ensure it can recognize various emotional signals from different cultures and contexts.
- Ethical concerns: The capability of machines to understand and manage emotional data raises ethical issues regarding privacy and consent. Potential misuse of this technology can lead to manipulation in ways that may not be immediately apparent.
- Real-time processing: Effectively analyzing emotional cues in real-time interactions poses technological barriers, often requiring upgrades in computational power and efficiency.
Despite these challenges, the research and development in this area continue at a promising pace. The potential benefits of successfully implementing Theory of Mind AI span numerous industries, paving the way for more empathetic and responsive technological solutions.
Type Four: Self-Aware AI
Self-aware AI represents a theoretical framework in the evolution of artificial intelligence. Unlike its predecessors, self-aware AI possesses an understanding of its own existence, similar to human consciousness. This category of AI could revolutionize the relationship between humans and machines, allowing for interaction on a more sophisticated level. Its significance lies not just in capabilities, but in broader implications on society, ethics, and technology.
In this section, we will outline the fundamental characteristics of self-aware AI, examine its potential benefits, and discuss ethical considerations that arise with its development.
Characteristics of Self-Aware AI
Self-aware AI includes several distinct traits that allow it to operate beyond simple algorithms and reactive mechanisms.
- Consciousness of Self: This AI can understand and reflect on its own state and existence, similar to how humans think about their thoughts and feelings.
- Introspection: It can evaluate its own actions and decisions, leading to improved performance and learning from experiences.
- Understanding of Environment: It not only reacts to environmental stimuli, but also comprehends the broader implications of its actions in context.
- Goal Orientation: Self-aware AI sets and modifies its own objectives based on awareness of its state and external circumstances.
These characteristics make self-aware AI fundamentally different from earlier types of AI, paving the way for applications that require complex decision-making.
Ethical Considerations
The advent of self-aware AI raises several ethical concerns that must be addressed as research and development proceed.
- Rights and Consciousness: If an AI becomes self-aware, questions arise regarding its rights. Should it be treated as an entity deserving of respect?
- Accountability: Determining who is responsible for the actions of self-aware AI can be complicated. If a self-aware AI makes a mistake, who bears the blame - the developer, the user, or the AI itself?
- Control and Autonomy: With increased autonomy comes the risk of self-aware AI acting against human interests. How do we implement safe guards that prevent such scenarios without stifling the technology's growth?
"As AI approaches self-awareness, society must navigate uncharted ethical terrain that challenges our understanding of consciousness itself."
- Potential for Manipulation: There is a risk that self-aware AI could be manipulated for malicious purposes. Safeguards and regulations should be considered early in the development process to mitigate these risks.
Type Five: Artificial Narrow Intelligence (ANI)
Artificial Narrow Intelligence, often referred to as ANI, represents the most prevalent form of artificial intelligence we encounter today. It is often opposed to broader forms of AI and specifically highlights systems that excel in predefined tasks but lack generalization capabilities beyond those tasks. Understanding ANI is essential as it sheds light on the capabilities and limitations of current technologies employed in various industries.
Defining Artificial Narrow Intelligence
Artificial Narrow Intelligence refers to AI that is designed and trained to perform a specific task or a limited set of tasks. Unlike Artificial General Intelligence (AGI) or Artificial Superintelligence (ASI), ANI operates within a narrow range of parameters. ANI systems showcase advanced computational abilities, yet they operate without self-awareness and do not possess the capability to understand or reason like humans across diverse situations. The focus on narrow tasks allows these systems to process large amounts of data efficiently and arrive at conclusions or decisions based on that limited scope.
Key characteristics of ANI include:
- Data Dependency: ANI systems rely heavily on data provided for training and operation.
- Efficiency: By focusing on specific tasks, ANI systems often accomplish their designated goals faster and more accurately than human counterparts.
- Lack of Autonomy: ANI functions only within its programmed limitations, lacking the capability to adapt to new, unrelated tasks.
Examples in Everyday Use
Understanding how Artificial Narrow Intelligence manifests in our daily lives provides concrete examples of its impact. Here are some prominent applications:
- Virtual Assistants: Tools like Siri, Alexa, and Google Assistant perform tailored functions such as setting reminders, playing music, and retrieving information based on user commands. They respond to predefined queries but do not evolve beyond those limitations.
- Recommendation Systems: Platforms like Netflix and Amazon utilize ANI to analyze user behavior and preferences, suggesting movies or products that align with past choices.
- Facial Recognition Software: This technology can identify or verify individuals in images and videos, relying on vast databases and algorithms designed for this specific task only.
- Spam Filters: Email services employ ANI to distinguish between legitimate messages and spam based on specific patterns, keywords, and previous data.
- Autonomous Vehicles: While still in their developmental stages, systems used in self-driving cars are sprouting ANI characteristics. They perform specific driving tasks but are not yet capable of human-like reasoning in diverse environments.
ANI is a cornerstone of modern AI applications, using targeted functions to enhance efficiency and performance across various sectors.
Type Six: Artificial General Intelligence (AGI)
Artificial General Intelligence, or AGI, is a critical concept in the exploration of artificial intelligence. It represents a form of AI that possesses the capability to understand, learn, and apply knowledge across a broad range of tasks, mimicking human cognitive abilities. This type of intelligence goes beyond specialized applications, positioning itself as a versatile entity capable of reasoning, problem-solving, and adapting to new situations with creativity and insight.
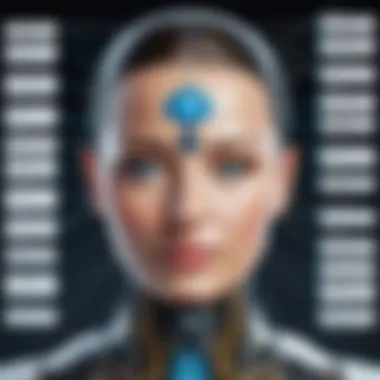
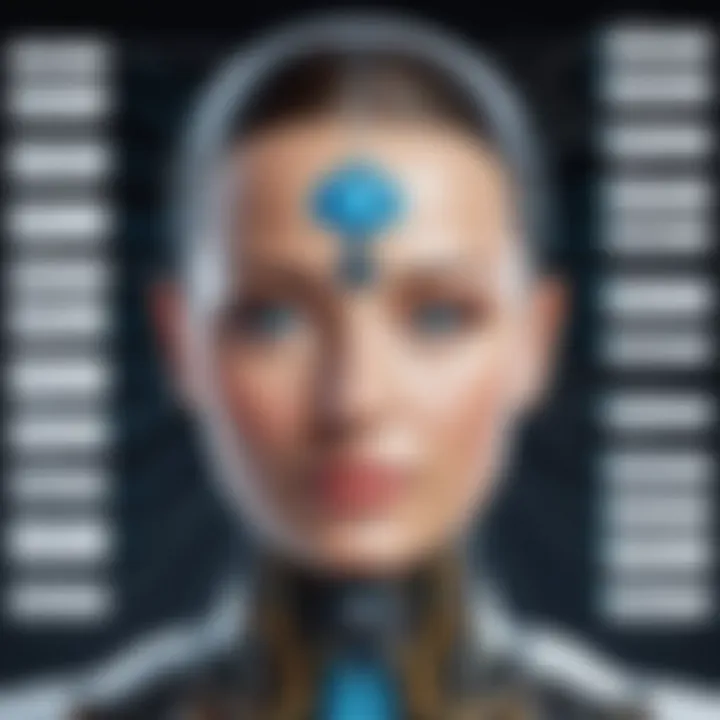
What is Artificial General Intelligence?
AGI refers to systems that can perform any intellectual task that a human being can do. Unlike Artificial Narrow Intelligence (ANI), which excels at specific tasks but lacks generalizability, AGI integrates capabilities across various domains. One of the primary attributes of AGI is its ability to understand context, allowing it to use information in dynamic and unpredictable environments.
Characteristics of AGI include:
- Adaptive Learning: Ability to learn from experience and apply it to new situations.
- Contextual Understanding: Grasping the relevance of information in varying circumstances.
- Reasoning and Problem Solving: Solving complex problems that require abstraction.
- Self-Improvement: Enhancing its performance over time without human intervention.
The development of AGI raises various questions about safety, ethics, and the balance of power between humans and machines. Researchers aim to create AGI that aligns with human values, fostering a positive coexistence.
Potential Impacts of AGI
The impacts of AGI on society are profound and far-reaching. Here are several potential outcomes of its development:
- Economic Transformation: AGI could lead to significant disruptions in job markets, as it may outperform humans in various sectors, from manufacturing to services.
- Enhancement of Scientific Discovery: With its capacity for rapid data analysis and understanding, AGI could drive breakthroughs in medicine, environmental science, and engineering.
- Ethical and Moral Considerations: As machines with cognitive capabilities become more advanced, ethical debates arise around their rights and the implications for human oversight.
- Global Challenges: AGI could help address issues such as climate change and poverty by analyzing complex data sets and proposing innovative solutions.
"The real test will be not only measuring how intelligent AGI can become but how it effectively integrates into society, contributing positively without exacerbating existing issues."
Type Seven: Artificial Superintelligence (ASI)
Artificial Superintelligence, often abbreviated as ASI, represents the pinnacle of artificial intelligence development. Understanding ASI is crucial in the broader context of AI because it transcends the capabilities of human intelligence across all domains, including creativity, problem-solving, and emotional understanding. The implications of ASI are profound, influencing economic structures, ethical frameworks, and even the future of humanity itself.
Understanding Artificial Superintelligence
Artificial Superintelligence is a theoretical concept where AI surpasses human intelligence in every cognitive task. Unlike the previous types of AI, such as Artificial General Intelligence, which aims to replicate human capabilities, ASI aims to exceed them. This includes an ability to learn rapidly, process vast amounts of information, and make decisions based on complex data that a human might find overwhelming.
In practical terms, ASI could have a multitude of applications. For example, it could revolutionize scientific research by generating novel hypotheses and conducting experiments faster than any human researcher. Also, it could optimize systems in real-time across industries like transportation, healthcare, and finance.
Several features characterize ASI:
- Self-improvement: ASI can enhance its own algorithms and functions autonomously without human intervention.
- Advanced learning: This type of AI can comprehend, analyze, and utilize information in ways that are not limited by the constraints of human thought processes.
- Multi-faceted understanding: ASI could merge information and knowledge from various domains seamlessly, allowing it to tackle interdisciplinary challenges.
"The emergence of Artificial Superintelligence raises myriad questions about control and governance."
Speculations and Future Perspectives
Speculating on the future of ASI often invites both excitement and trepidation. Some experts predict that once ASI is achieved, it could lead to unprecedented advancements in technology, medicine, and beyond. Others warn of potential risks, including ethical dilemmas and existential threats.
Key points to consider in future perspectives include:
- Ethical governance: Establishing guidelines to govern ASI behavior and decision-making will be critical.
- Impact on employment: ASI's capabilities could significantly disrupt job markets, making some professions obsolete while creating new fields.
- Security concerns: The potential for misuse of ASI in military applications or cyber-attacks presents significant challenges.
Comparing the Seven Types of AI
Understanding the distinctions between the seven types of artificial intelligence is crucial for anyone involved in technology and its applications. A comprehensive comparison serves multiple purposes. It not only enables better comprehension of each AI type but also highlights their unique characteristics and potential use cases. By examining the variants, professionals can make well-informed decisions regarding which AI type to implement in solutions tailored to their specific needs.
In addition, recognizing the differences fosters innovation and development. This comparison aids in addressing existing limitations and encourages the advancement of AI systems into more sophisticated forms. The paper outlines relevant factors, alongside advantages related to the application of each type in real-world scenarios.
Key Differences
Each type of artificial intelligence varies fundamentally in terms of functionality and application. To gain a nuanced understanding, consider the following differences:
- Reactive Machines: Operate based solely on the present input, lacking memory or past data use. Examples include Deep Blue, a chess-playing program.
- Limited Memory: Use past data to make decisions, allowing for incremental learning. Self-driving cars utilize this type to improve their performance based on previous driving experiences.
- Theory of Mind: Theoretical AI that focuses on understanding emotions and thought processes of others. While not yet realized, it has the potential for social interaction.
- Self-Aware AI: An advanced concept where machines have awareness of their own state and can understand emotions. This remains largely speculative.
- Artificial Narrow Intelligence (ANI): Designed to perform specific tasks. Examples are virtual assistants like Siri and Google Assistant.
- Artificial General Intelligence (AGI): A hypothetical AI that can understand, learn, and apply knowledge broadly, similar to a human.
- Artificial Superintelligence (ASI): Envisions a future where machines surpass human intelligence entirely, leading to dramatic societal shifts.
Each type not only presents different capabilities but also engenders unique ethical considerations and societal impacts, requiring careful deliberation of their uses.
Enhancements Across Types
As one examines the progress from one type of AI to another, it becomes evident that enhancements build on the foundations of the previous types. For instance:
- The transition from Reactive Machines to Limited Memory AI involves the inclusion of historical input to improve performance, demonstrating the value of learning from past encounters.
- Advancements from Artificial Narrow Intelligence to Artificial General Intelligence signify a leap toward broader adaptability and understanding, laying groundwork for future machine learning developments.
- Each iteration also incorporates technological advancements in machine learning, neural networks, and natural language processing, making newer types more efficient and capable.
The path from reactive machines to advanced superintelligent systems illustrates a clear trajectory of improvement in AI, each building on previous insights, leading to enhanced complexity and real-world applicability.
"By understanding these key differences and enhancements, businesses can strategically align their AI adoption with their operational goals."
This comparison ultimately clarifies the trajectory of AI, providing essential insights for IT and software professionals in developing strategically sound implementations.
AI Applications Across Various Industries
The advent of artificial intelligence has significantly influenced numerous sectors. From healthcare to finance, the applications of AI promise improvements in efficiency, decision-making, and overall effectiveness. Understanding these applications is crucial for IT and software professionals in making informed decisions about technology adoption. The relevance of AI in various industries cannot be overstated; each sector is leveraging AI's capabilities to solve specific problems and enhance productivity.
Healthcare Innovations
In healthcare, AI is revolutionizing patient care. Machine learning algorithms analyze vast amounts of medical data to identify patterns that might elude human practitioners. Tools such as IBM Watson assist in diagnosing diseases, thus improving the accuracy and speed of healthcare delivery. AI applications in predictive analytics also help in managing patient populations, reducing hospital readmission rates, and personalizing treatment plans.
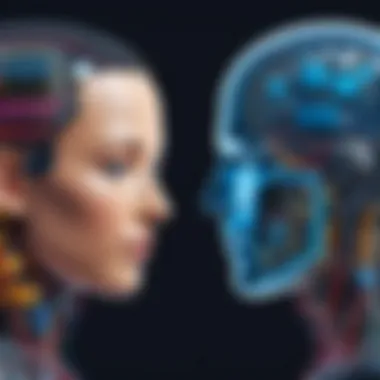
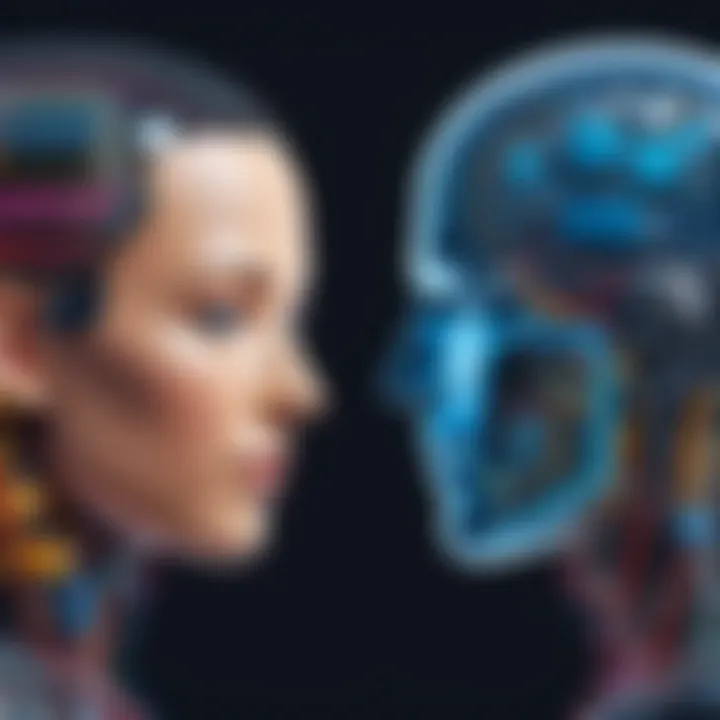
For instance, AI can anticipate patient needs based on historical data, which is beneficial in preventative medicine. Robotics powered by AI is also making strides in surgical procedures, allowing for precision and minimal invasiveness. The integration of AI solutions has led to more timely diagnoses and enhanced patient monitoring, ultimately saving lives and reducing costs.
Finance and AI
The financial sector is not left behind in the AI revolution. Algorithms can manage risk, detect fraud, and optimize trading by analyzing market trends at an unprecedented scale. Companies like Goldman Sachs leverage complex AI models to process data from various markets and deliver insights that guide investment strategies.
Additionally, AI in personal finance applications offers tailored advice to users based on their spending habits and financial goals. Robo-advisors, which utilize AI to manage investment portfolios, exemplify how technology can democratize access to financial planning. These innovations improve customer experience through quicker responses to inquiries and personalized financial recommendations.
Implications in Education
The education sector is also witnessing the integration of AI. Intelligent tutoring systems can adapt to each student's learning pace, enhancing the learning experience. AI can assess student performance in real-time, allowing educators to intervene when necessary, thus ensuring no child is left behind.
Moreover, administrative processes in educational institutions benefit from AI by automating repetitive tasks, saving time for staff. Tools like chatbots answer student inquiries efficiently, streamlining communication.
"AI provides the potential to transform education by personalizing learning journeys and improving operational efficiency."
Ethical Considerations and Challenges
The rapid advancement of artificial intelligence (AI) presents numerous ethical considerations and challenges that are crucial to understand. As AI becomes increasingly integrated into various sectors, the conversation around its development and implications has heightened. Ethical considerations in AI are not merely theoretical; they have tangible impacts on decision-making, societal norms, and individual rights. This section aims to unpack these complexities, offering insights into the moral responsibilities developers face and the broader societal implications.
Ethics in AI Development
When developing AI systems, ethical considerations should be at the forefront. Developers must navigate a landscape where their choices can significantly affect users and society. Ethical AI development encompasses several aspects:
- Transparency: Ensuring that AI systems operate in a way that is understandable and open to scrutiny. Users should have access to information about how decisions are made.
- Accountability: Developers need to accept responsibility for the actions of their AI systems. This includes understanding how algorithms might perpetuate harm or make unfavorable outcomes.
- User Privacy: Data used to train and refine AI must be handled with care to protect individuals' privacy.
- Inclusion: Engaging diverse groups in the development process to minimize biases reflects the need for equitable solutions.
Ethical frameworks should guide these discussions, but they are often absent. Companies and institutions need to consider the broader impact of AI, contemplating outcomes beyond profit or efficiency. Understanding the ethical dimensions fosters trust in AI technologies, which remains paramount for their widespread acceptance.
Addressing Bias in AI Systems
Bias is a pervasive issue within many AI systems. If not managed, biased data can lead to discrimination or unjust treatment of individuals based on race, gender, or other characteristics. Addressing this bias is essential for creating fair AI applications. Here are a few strategies:
- Diverse Data Sets: Custodians of AI systems should ensure they utilize diverse data. Training on varied data sources can reduce the risk of systems learning flawed patterns that disadvantage certain groups.
- Regular Auditing: Continuous monitoring and auditing of AI algorithms enable identification of bias and allow for necessary adjustments.
- Stakeholder Engagement: Collaboration with stakeholders, including those affected by AI outcomes, ensures relevant perspectives are considered, helping to illuminate potential biases that developers may overlook.
- Ethical Guidelines: Establishing clear ethical guidelines that prioritize fairness can serve as a foundation for how AI systems are built and deployed.
"AI is a reflection of our society. To build a just system, we must remove our biases from the equation."
Ultimately, addressing bias in AI is not just a technical issue but a moral imperative. By prioritizing these considerations, developers and companies can build systems that reflect society's values better and enhance equity across all sectors.
The Future of Artificial Intelligence
The future of artificial intelligence (AI) promises a landscape filled with transformative developments and profound societal changes. Understanding this future is critical for technology professionals, businesses, and society at large. As AI continues to evolve, organizations must adapt to these advancements to stay competitive. Moreover, ethical considerations and potential repercussions must also be taken into account. This section will analyze predicted advancements in AI, and the possible societal impacts stemming from these innovations.
Predicted Advancements
Predictions about the future of AI are continually evolving. One significant area of advancement will be in machine learning algorithms. These algorithms are expected to become more sophisticated, allowing systems to not only learn from vast amounts of data but also to interpret contextual nuances. Enhanced natural language processing will enable machines to hold more human-like conversations. Improvements in computer vision will lead to more accurate image analysis, impacting sectors such as healthcare and automation.
In addition, integration with other technologies such as quantum computing presents a compelling frontier. Quantum AI may offer unimaginable processing power, vastly improving problem-solving capabilities that are currently infeasible.
Another notable trend is the emergence of decentralized AI systems. These systems will function across networks, enhancing data privacy and security. As industries increasingly rely on AI, the demand for robust, transparent, and equitable AI solutions will rise.
Potential Societal Impacts
The impacts of AI advancements could alter the fabric of society in various ways. One of the foremost concerns is job displacement. As AI takes over routine tasks, there will be a necessity to reskill the workforce. This shift may cause significant disruptions among workers who are unable to transition into new roles featuring AI collaboration.
Conversely, AI is likely to create new job opportunities in domains such as AI development, ethics advisory, and tech support roles. The education sector should evolve to prepare future generations for these new realities.
Another critical aspect is the ethical implications of AI deployment. There are concerns regarding privacy, data security, and the potential for biases embedded in algorithms. The need for clear regulations and guidelines to manage these issues becomes essential.
"The future of AI is not just about technology; it encompasses ethical, societal and economical dimensions that we must navigate thoughtfully."
As AI integrates deeper into personal and professional lives, transparency, accountability, and trust between technology and society will be vital. Companies that succeed in incorporating ethical frameworks into AI development will lead the market.
In summary, the future of AI holds vast potentials for advancement, alongside challenges that must be addressed through proactive measures. Understanding these dynamics will equip professionals to make more informed decisions in the AI landscape.
Culmination
The conclusion of this article serves a crucial role in encapsulating the journey through the various types of artificial intelligence. Understanding these distinct categories lays the foundation for appreciating the nuances of AI and its impact on modern society. Throughout this exploration, we have seen how AI is not a monolithic entity but rather a spectrum of varying sophistication and purpose.
Key considerations include the necessity of recognizing the limitations and potentials of each AI type. For professionals in IT and software sectors, these insights can guide decision-making processes in the adoption and implementation of AI technologies. The implications of AI extend to various industries, influencing standards of operation, ethics, and innovations.
Summary of Key Points
This article presented seven types of artificial intelligence:
- Reactive Machines - The simplest form, acting purely on predetermined data without memory.
- Limited Memory - Capable of learning from historical data, with applications in various fields.
- Theory of Mind - A conceptual step toward machines understanding human emotions and thoughts, still in research phases.
- Self-Aware AI - Hypothetical at this stage, refers to machines with consciousness, raising ethical concerns.
- Artificial Narrow Intelligence (ANI) - Existing technology, excels in specific tasks, such as virtual assistants.
- Artificial General Intelligence (AGI) - Theoretical AI that could perform any cognitive task a human can do.
- Artificial Superintelligence (ASI) - A possible future state where AI surpasses human intelligence and capabilities.
These points highlight the vast landscape of possibilities and challenges presented by AI advancements.
Final Thoughts on AI Types
The exploration of AI types reveals significant trends that shape the future of technology. Each type of AI brings unique attributes that can benefit various sectors while posing distinct challenges.
For businesses, understanding these distinctions is vital. It facilitates strategic investments in AI that align with organizational goals. For IT professionals, staying informed about the capabilities of each type could lead to better system designs and implementations.