Understanding SPSS: Comprehensive Overview of its Applications
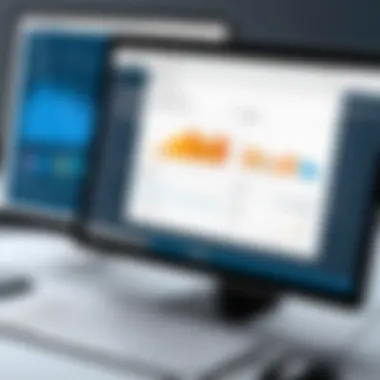
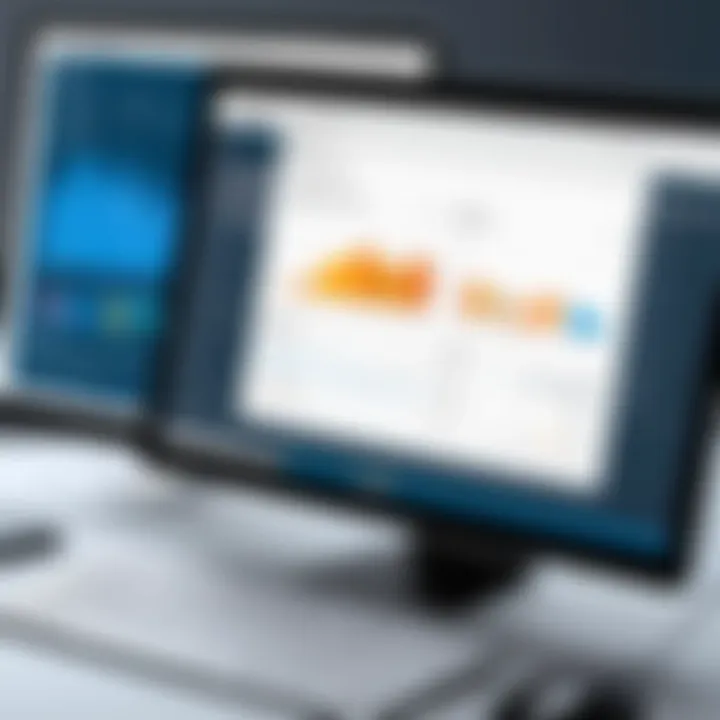
Intro
In the realm of data analysis, SPSS holds a significant spot as both a tool and a topic of intrigue. For anyone treading the waters of statistics, whether in social sciences, healthcare, or the broad domain of business analytics, understanding SPSS can be a game changer. Statistics might seem intimidating at first, but with the right tool, it transforms into a structured set of insights. SPSS, short for Statistical Package for the Social Sciences, is not just a software; it's a platform that allows users to distill complex data into actionable information.
The importance of SPSS transcends its technical features; it lays the groundwork for evidence-based decision-making. In today’s world, where data drives strategy, a grasp on SPSS can empower individuals and organizations alike. But what exactly makes SPSS the go-to option for analysts? What are the core functionalities that set it apart? This overview aims to unfold each aspect intricately, serving IT professionals, software developers, and business entities with substantial insights into SPSS.
With a solid understanding of the software's functionalities, methodologies, and real-world applications, you'll be better equipped to harness data effectively and contribute value to any analytical endeavor.
Preamble to SPSS
In today's data-driven landscape, the need for effective statistical analysis is paramount. This is where SPSS, or the Statistical Package for the Social Sciences, enters the fray as a powerhouse tool. Understanding SPSS offers not just a glimpse into statistical analysis, but also underscores its significance across various fields such as academia, healthcare, and business. This software is akin to a Swiss Army knife for data analysts, ready to tackle diverse tasks ranging from data management to complex statistical modeling.
SPSS enables professionals to convert raw data into actionable insights. The software provides numerous functionalities that help in conducting rigorous analyses, which informs decision-making processes. As one delves into the world of SPSS, grasping its foundational elements becomes vital. From managing datasets to interpreting results, each aspect plays an essential role in the workflow of statistical analysis.
Moreover, there’s a myriad of scenarios where SPSS shines. Whether it's in academic research where hypotheses need testing, or in businesses aiming to understand consumer behavior, SPSS provides the tools necessary to make sense of the overwhelming amount of data available today. However, what stands out is not just its capabilities, but also the considerations one must keep in mind while using it—like data integrity and ethical analysis practices.
Understanding SPSS is more than knowing how to use software; it represents a commitment to applying statistical principles thoroughly and thoughtfully.
History of SPSS
SPSS traces its roots back to the late 1960s, a time when statistical analysis was primarily confined to academic circles. The software was developed by Norman H. Nie, C. Hadlai Hull, and William G. M. Stein as a solution for researchers who needed an efficient method for performing complex statistical work without getting bogged down in the nitty-gritty of programming.
Initially, SPSS ran on mainframe computers, which limited access to those with substantial resources. However, as computing technology advanced, SPSS transitioned to personal computers in the 1990s. This migration opened the door for more users, effectively democratizing access to powerful statistical tools.
Over the years, SPSS has undergone numerous updates and has expanded its functionalities. The acquisition by IBM in 2009 marked another significant transformation, elevating SPSS's capabilities with greater integration into data science methodologies. Today, SPSS stands not just as a tool, but as an industry standard for statisticians and data analysts around the globe.
Basic Concepts of SPSS
At its core, SPSS is designed for ease of use, adorned with an intuitive interface that allows users to execute sophisticated statistical analyses with just a few clicks. Some basic concepts one should familiarize themselves with include:
- Data View and Variable View: These two modes allow users to edit data and define variable characteristics, respectively. Understanding these views is fundamental as they form the backbone of how data is managed within SPSS.
- Syntax and Output: SPSS can be used both via its graphical interface and through syntax commands. Learning to leverage syntax can enhance efficiency, especially for repetitive tasks. The output window is where results materialize, presenting findings in a structured format.
- Types of Data: Recognizing the distinctions between nominal, ordinal, interval, and ratio data types is crucial. The type of data influences which statistical tests can be performed.
In summary, embracing the fundamental concepts of SPSS not only aids in navigating the software efficiently, but also enables users to derive meaningful insights from their datasets.
Key Features of SPSS
Understanding SPSS’s key features is vital for professionals and businesses that rely on data for decision-making. This software, known for its robust analytical capabilities, offers an array of functionalities that make it indispensable for anyone involved in statistical analysis. In this section, we will delve deeper into three core features: Data Management Capabilities, Statistical Analysis Tools, and Graphical Representation of Data, providing a thorough look at how these elements work together to enhance the analytical experience.
Data Management Capabilities
SPSS excels in its data management features, serving as a powerful hub for data preparation. This facet of SPSS is crucial because, without properly organized data, the results of any analysis can lead to incorrect conclusions. SPSS facilitates users in handling large datasets efficiently, allowing the import of various formats like Excel spreadsheets, SQL databases, and CSV files.
Furthermore, the software supports data cleaning operations, enables recoding of variables, and provides functionalities for merging and aggregating datasets. User-friendly features like drag-and-drop functionality simplify the data manipulation process, making it accessible even to those who may not be very inclined towards statistical programs.
Proper data management is like laying solid groundwork for a house—without it, everything else can topple over.
The intuitive interface provides tools for handling missing data, identifying outliers, and reshaping data structures, so you can focus on analysis rather than data wrangling.
Statistical Analysis Tools
Statistical analysis is the very heartbeat of SPSS. The software offers a comprehensive suite of statistical tests ranging from basic descriptive statistics to more complex inferential analyses. Whether you're looking to compute averages, conduct t-tests, or carry out ANOVA, SPSS has you covered with built-in functions that make these processes straightforward.
The importance of these tools cannot be overstated, especially in fields that depend on data to validate hypotheses or generate insights. Researchers can easily select the necessary tests through user-friendly dialog boxes, and the software even suggests suitable tests based on the selected data.
Additionally, SPSS incorporates advanced statistical procedures like multivariate analysis, which is pivotal for examining relationships among several variables at once. This brings a layer of sophistication to analyses that traditional spreadsheets simply can't match.
Graphical Representation of Data
Visualizing data is just as crucial as the analysis itself, and SPSS shines in this regard. The software offers a variety of ways to represent data graphically, whether you prefer bar charts, histograms, box plots, or scatterplots. Effective visualization aids in conveying results clearly and succinctly to stakeholders, providing insights at a glance.
Creating these visual aids is often customizable, allowing users to tweak elements such as colors, labels, and scales to meet their specific needs. The clarity and professionalism of SPSS graphics make them suitable for presentations, reports, or even publications.
Furthermore, graphical outputs can easily be exported for use in other applications, expanding their utility and enhancing the overall reporting process.
Overall, SPSS's key features—data management, statistical tools, and graphical representation—work synergistically. They not only streamline the analytical process but also elevate the quality of research outputs, making it an invaluable tool for data-driven decision-making across various fields.
Applications of SPSS
Understanding the various applications of SPSS is essential for anyone looking to harness its full potential. This powerful tool is not just a statistical software but a versatile platform that can cater to diverse fields. There’s a good chance you’ll be seeing SPSS pop up in academic research, business environments, and even the healthcare sector. Each of these areas leverages SPSS in unique ways, creating a tapestry of applications that showcase its relevance and utility.
Use in Academic Research
In the realm of academia, SPSS is often the backbone of research analysis. Scholars, students, and researchers utilize it to meticulously explore data sets. Whether conducting surveys or experiments, the robust statistical methods available allow researchers to analyze large volumes of information efficiently.
One of the standout features here is its ability to handle complex statistical tests. This means researchers don’t just scratch the surface; they can delve deep into behavioral patterns, correlations, and even predictive modeling. For example, a psychology researcher might use SPSS to determine if there is a significant relationship between social media usage and anxiety levels among students. This not only enhances the academic rigor of their work but also strengthens their findings.
Some key benefits of using SPSS in academic research include:
- Data Management: Easily manage, clean, and prepare data for analysis.
- Statistical Techniques: Access to a wide array of statistical methods, from basic descriptives to advanced modeling and inferential statistics.
- User-Friendly Interface: Facilitates learning for newcomers who might not have a strong statistical background.
Business Applications
When we look at the business landscape, SPSS holds its own as a crucial tool for decision-making. Companies today generate vast amounts of data, and SPSS allows them to make sense of it all. From market research to customer segmentation, the software aids businesses in crafting strategies based on hard data.
For instance, imagine a retail organization looking to understand consumer purchasing behavior during sale seasons. They can employ SPSS to analyze past sales data, identify trends, and forecast future sales. This insight not only guides inventory decisions but also tailors marketing strategies to target specific demographics more effectively.
Benefits of implementing SPSS in business include:
- Predictive Analytics: Anticipate future trends to stay ahead of the competition.
- Data Visualization: Present findings in compelling graphical formats, making them easier for stakeholders to digest.
- Customer Insights: Gain a deeper understanding of customer preferences and behaviors.
Healthcare Analytics
In the healthcare field, SPSS is an indispensable tool, especially in analyzing patient data, clinical trials, and public health studies. Handling sensitive data is a requisite in healthcare analytics, and SPSS provides the necessary features to ensure data integrity and security while conducting analyses.
Consider a research team examining the effectiveness of a new medication. Using SPSS, they can analyze trial data to determine efficacy rates and side effects. This kind of analysis is vital, as it can influence treatment guidelines and health policies.
Some noteworthy applications in healthcare using SPSS include:
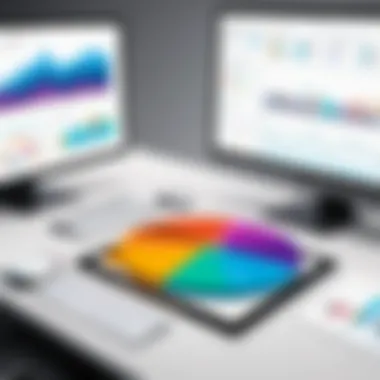
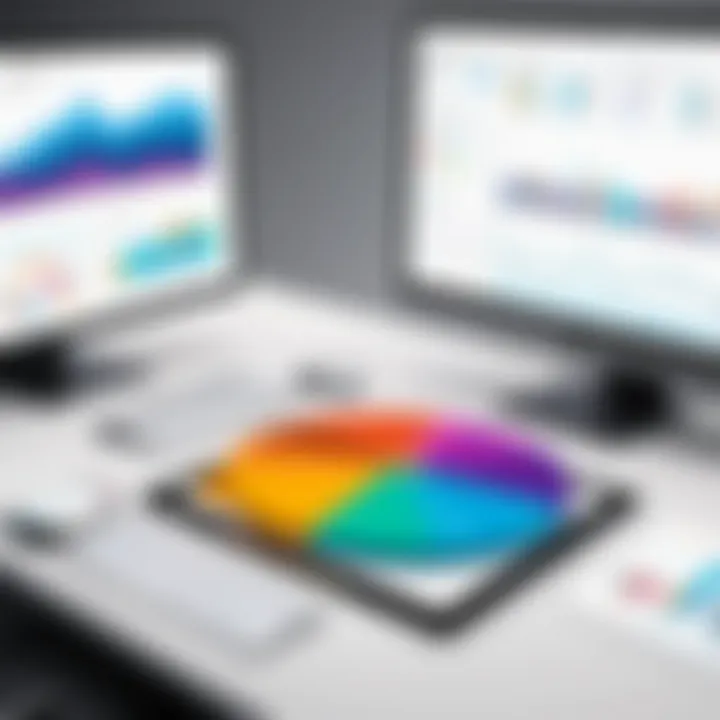
- Patient Outcome Analysis: Evaluate and improve treatment strategies based on systematic data analysis.
- Epidemiological Studies: Identify patterns and risks of diseases within populations.
- Resource Allocation: Optimize the use of healthcare resources through detailed data insights.
SPSS isn’t just a tool for number crunching—it’s a lens through which we can see critical patterns in data that can lead to impactful decisions across various fields.
By understanding how SPSS is applied across these sectors, professionals can leverage its capabilities to make informed decisions, improve operational efficiency, and ultimately, enhance outcomes.
This multifaceted utility highlights why SPSS remains a top choice for data analysis among IT professionals, business analysts, and healthcare practitioners alike.
SPSS License and Versions
The discussion surrounding SPSS licenses and versions holds considerable weight in the overall understanding of the software's functionality and accessibility. Every organization, whether it is a small startup or a large corporation, needs to make informed decisions about which version to adopt based on requirements, budget constraints, and usage expectations. The licensing models dictate not only the cost but also the features and support available, making it essential to grasp this topic thoroughly.
Available Versions
SPSS comes in several versions, each tailored for specific user needs. The primary ones are:
- SPSS Statistics: This is the most comprehensive version, ideal for users engaged in advanced statistical analyses. It includes various tools for regression, ANOVA, and advanced predictive analytics.
- SPSS Modeler: Focused on data mining, this version emphasizes predictive modeling, making it suitable for those in analytics and sectors requiring deeper insights from data.
- SPSS Amos: This is for structural equation modeling. It’s widely used in social sciences for path analysis and other complex model assessments.
- SPSS Survey Reporter: Aimed at market researchers, it simplifies the analysis of survey data and provides tools for report generation.
These versions cater to different analytical requirements, ensuring that organizations can find a suitable fit for their specific tasks. The fact that SPSS provides such specialized options underlines its adaptability and comprehensive nature.
Licensing Options
SPSS offers various licensing options to accommodate different usage scenarios:
- Individual License: Perfect for solo users, typically includes standard functionalities without extensive support. Cost-effective but limited in collaborative features.
- Volume License: Designed for organizations needing multiple copies. It often comes with a discount for bulk purchases but may have restrictions on distribution across departments.
- Site License: This option is ideal for larger institutions, providing access to all staff and students at a single location. The upfront cost can be high, but it fosters a collaborative environment.
- Cloud License: A newer model that allows users to access SPSS via the cloud. This one brings flexibility, no need for hefty installations, and potentially lower maintenance costs.
Choosing the right licensing option can significantly affect how SPSS can be utilized. Organizations must weigh their current and future needs and consider whether they have the budget to invest in extensive features or if a simpler version would suffice.
Installation and Setup of SPSS
Setting up SPSS properly is as crucial as knowing how to manipulate the data once it's there. Without a no-nonsense installation process and a solid understanding of system requirements, users might find themselves with a shiny software but no way to make it actually work. This section lays down some vital groundwork that needs to be well understood before jumping into statistical analysis and data management.
System Requirements
Before diving headfirst into the installation of SPSS, it's imperative to assess whether your system meets the necessary specifications. Like a ship without a sturdy hull, trying to run SPSS on unsupported hardware will lead to storms in your analytical journey.
Here's a snapshot of common system requirements:
- Operating System: SPSS typically runs on Windows and macOS. Ensure you have the correct version, like Windows 10 or macOS Mojave and above.
- Processor: A fast processor, ideally with multiple cores, will make a notable difference. At least a 2.1 GHz processor gets the job done.
- RAM: More is usually better here; at least 4 GB is recommended, but 8 GB or more allows smoother multitasking.
- Hard Drive Space: Ensure you have a minimum of 2 GB free, not just for installation but also for saving datasets and output files.
- Display: High-resolution screens will help you make sense of those detailed graphs and tables. A resolution of 1024 x 768 or higher is preferred.
Always double-check from the official IBM SPSS website for the most current requirements as software evolves and updates come out frequently.
Installation Process
Once the system checks out, the installation process of SPSS is fairly straightforward. However, as with any software, there can be bumps along the road. Adopting a careful step-by-step approach can save users from a world of headaches later on.
- Download the Software: Get the installation package from IBM's official site or from your institution, if it provides access to SPSS. Ensure you download the correct package for your operating system.
- Run the Installer: Once downloaded, locate the file and double-click to initiate the setup. Be patient as the installer prepares the necessary files.
- Follow Installation Prompts: The installation wizard will guide you through the setup. Generally, clicking through will suffice:
- License Information: You’ll be prompted to enter a license key. Having this ready is crucial, as the software will not run without it. If you’re using a trial version, simply select that option as well.
- Finish up: Once the installation completes, it’s wise to restart your system to ensure everything is in place and running smoothly. After the restart, launch SPSS to confirm that it works as expected.
- Accept the terms of service.
- Select 'Standard installation' unless you need to customize certain features.
As a general tip, read the installation guide provided in the installation package. Sometimes software comes with its quirks, and knowing them upfront can save you time.
Remember, a smooth installation ensures that the road ahead in your statistical analysis journey is devoid of unnecessary hurdles.
Navigating the SPSS Interface
Understanding the interface of SPSS is like unlocking the door to a treasure trove of data insights. The way the software is organized can significantly impact how efficiently users can conduct their analyses. Whether you're an IT professional, a statistician, or a business analyst, grasping the layout and functionalities of SPSS is essential.
The SPSS interface can appear overwhelming at first glance, but once you familiarize yourself with its structure, navigating through it becomes a breeze. The main components of the interface are the menu bar, toolbars, and the data editor. Each of these elements serves a specific purpose, providing access to various functionalities that streamline your data analysis process. The organized manner in which SPSS lays out its tools can enhance productivity and minimize errors.
Understanding Menus and Tools
At the top of the SPSS environment, you’ll find a menu bar that is akin to a roadmap, guiding you through different features and options. Each menu houses various submenus and tools that are crucial for performing statistical analysis. This hierarchical structure allows users to quickly locate specific functions such as data manipulation, statistical tests, and graphical representations.
Key Features of the Menu Bar:
- File: From here, you can open, save, and manage your data files.
- Edit: Offers tools for copying, pasting, or modifying existing data sets.
- View: Adjust settings for how the output and data editor are displayed.
- Data: This menu is heavily focused on data handling, including importing or exporting files.
- Analyze: Critical for performing statistical tests, including descriptive and inferential statistics.
- Graphs: Enables users to create various graphical representations of the data, which aids in visualization and interpretation.
- Utilities: Provides numerous additional tools and options that enhance the program’s capabilities.
Navigating these menus is straightforward, but some users may experience a bit of a learning curve. One suggestion is to keep a cheat sheet handy for the first few sessions as you explore different functionalities.
Working with Data Editor
The Data Editor in SPSS is where the magic happens. Imagine a digital spreadsheet that holds your sets of data, but with more power and analysis capabilities. The Data Editor provides a user-friendly grid layout, making it easier to view, edit, and manipulate your data. It consists of two views: the Data View and the Variable View.
- Data View: Displays the actual data entries in a tabular form. Each column represents a variable, and each row corresponds to one observation (or case). You can easily edit cells directly, making data entry straightforward while ensuring accuracy.
- Variable View: Here, you can define your variables, dictating properties such as variable names, types, labels, and measurement levels. Understanding how to navigate this section is crucial for maintaining data integrity.
The Data Editor is vital for ensuring that the data you analyze is clean and prepared correctly. This is where preliminary data cleansing occurs, setting the stage for accurate analysis later. Complete familiarity with the Data Editor boosts your efficiency and effectiveness as an SPSS user, making it easier to manage complex data sets without breaking a sweat.
"The interface of SPSS is your partner in data analysis; know it well, and it will serve you efficiently."
Data Analysis Techniques in SPSS
Data analysis techniques are the backbone of any statistical investigation. In the context of SPSS, understanding and utilizing these techniques is vital for transforming raw data into meaningful insights. Whether you are in academia, healthcare, or business, mastering these methods can significantly enhance your ability to make data-driven decisions. SPSS provides a plethora of statistical tools tailored to various data analysis needs, ensuring a robust framework for researchers and practitioners alike.
Descriptive Statistics
Descriptive statistics serves as an initial footing in the realm of data analysis. This set of techniques helps summarize and visualize the basic characteristics of a dataset. Think of it as painting a clear picture of what the data looks like before diving deeper into complex analysis.
- Central Tendency: Key measures like mean, median, and mode help in determining the center of the dataset. Each of these measures provides a unique perspective. For instance, the mean is sensitive to outliers, while the median offers a more resilient view.
- Dispersion: Metrics such as range, variance, and standard deviation are essential in understanding the variability of data. High variance may indicate diverse data points, while low variance suggests that the data points cluster closely around the mean.
- Data Visualization: SPSS has tools for creating charts and graphs, such as histograms and box plots, which make it easier to interpret descriptive statistics. Visuals capture attention and can communicate complex ideas quickly.
Keep in mind, descriptive statistics does not make any inferences beyond the immediate data, but it lays the groundwork. As the saying goes, “You can’t draw conclusions without having a good look at the data.”
Inferential Statistics
Inferential statistics is the bridge that connects sample data to broader generalizations about a population. In this sense, it goes beyond mere description to draw conclusions and make predictions.
- Hypothesis Testing: Techniques like t-tests, chi-square tests, and ANOVA (Analysis of Variance) allow you to assess the validity of hypotheses. Hypothesis testing helps answer questions, like whether a new drug is effective or if a marketing strategy yields better sales than a previous one.
- Confidence Intervals: This concept quantifies uncertainty about estimates. Instead of providing a single point estimate, confidence intervals showcase a range within which you can expect the true population parameter to lie.
- Sampling Techniques: SPSS supports various sampling methods. This is important; after all, errors can creep in from poorly designed samples or improper data collections.
Overall, inferential statistics not only utilizes the data at hand but also allows one to make educated guesses about the larger picture. It’s about understanding trends without needing to gather every single fact.
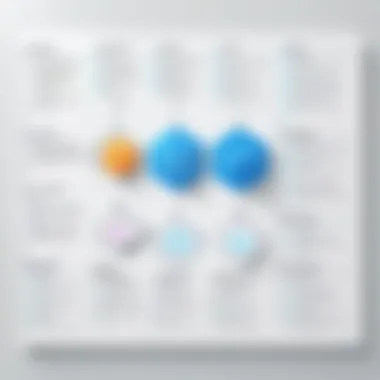
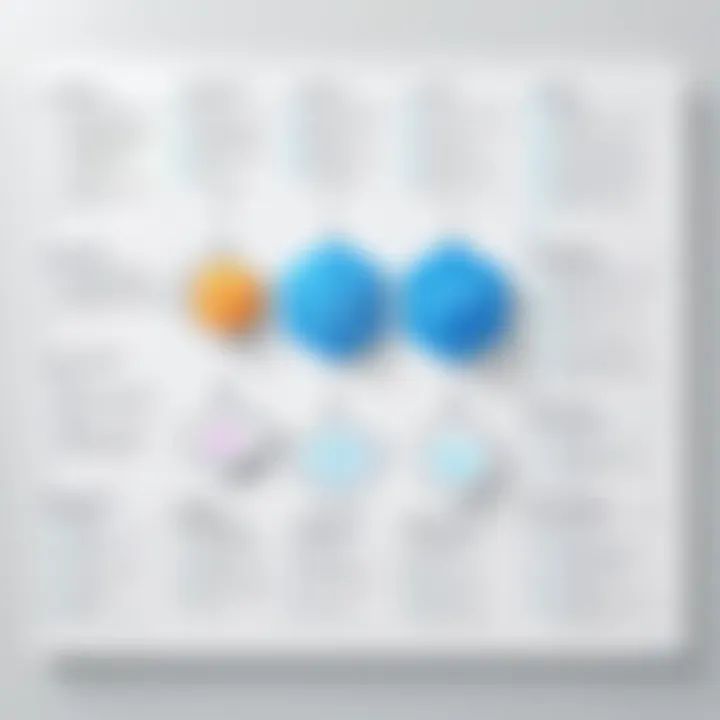
Regression Analysis
Diving deeper into data relationships, regression analysis emerges as a fundamental technique for predicting outcomes based on predictor variables. It’s like having a crystal ball that shows how different elements affect your target variable.
- Simple Linear Regression: This technique examines the relationship between two variables. For example, predicting sales based on advertising spend represents a typical use case. The simplicity of this approach aids in grasping the correlation between factors.
- Multiple Regression: Unlike simple linear regression, multiple regression goes further by analyzing multiple independent variables simultaneously. For instance, predicting home prices can include factors like location, square footage, and age of the property.
- Model Diagnostics: After a regression model is created, it’s essential to assess its credibility. Residual analysis helps in understanding how well the data aligns with the model. A good fit indicates that the predictions resonate with real-world values.
"Regression is not just a statistical tool; it's a means to unlock the relationships hidden within your data."
Interpreting SPSS Output
Interpreting the output generated by SPSS is a crucial aspect of data analysis. The results determine the direction of decisions, research conclusions, and policy formulations. Essentially, SPSS provides a visual representation of complex data using tables and graphs, making it more accessible to users. Understanding these outputs is vital for extracting actionable insights from the statistical analyses conducted within the software.
When one delves into SPSS output, it's key to recognize that the findings have significance beyond mere numbers. They tell a story—one about the data's trends, correlations, and even any anomalies that might exist. Whether in academia, business, or healthcare, the ability to interpret these outputs can facilitate informed decisions and strategies that have real-world implications. Hence, grasping the nuances involved in understanding this output can profoundly affect outcomes.
Understanding Output Tables
Output tables are a primary component of SPSS results. They summarize the statistical findings and present them in a structured format. This organization allows users to glean essential information at a glance. Here's a breakdown of how to make sense of these tables:
- Identify the Table Types: SPSS provides various table formats, such as descriptive statistics, correlation, and regression tables. Each serves a unique purpose and provides different insights.
- Look for Key Statistics: In descriptive tables, common statistics include means, medians, modes, and standard deviations. Understanding these can reveal underlying patterns within the dataset.
- P-Values and Significance: These are vital in inferential statistics. A small p-value (typically 0.05) suggests that the results observed are statistically significant, implying they are unlikely to have occurred by chance.
- Effect Sizes: These offer a measure of the strength of a phenomenon. Knowing the effect size can inform how impactful the findings might be.
Thus, taking the time to dig into these tables offers a clearer understanding of not just the results but the context in which the data exists.
Navigating Graphical Outputs
Graphs add an additional layer of understanding to data interpretation. They can illustrate trends and relationships more effectively than tables alone. Here’s what to consider when evaluating SPSS graphical outputs:
- Types of Graphs: SPSS can generate bar charts, histograms, scatter plots, and more. Each type conveys different patterns in the data. For instance, scatter plots can highlight correlations and linear relationships between variables, whereas bar charts can demonstrate comparative data across categories.
- Legends and Labels: Pay close attention to legends and axis labels. They provide essential details that inform what the graph represents. Misinterpretation due to overlooking these elements can lead to incorrect conclusions.
- Trends and Patterns: Look for trends indicated by the graphical data. An upward trend in a line graph, for example, can suggest a positive correlation between variables.
Visual representations in SPSS are not just embellishments; they are powerful tools that can quickly communicate complex information at a glance.
Understanding SPSS outputs, both graphical and tabular, is essential for deriving meaningful insights and making informed decisions based on empirical data.
Common Challenges and Solutions
Navigating through SPSS can be akin to traversing a labyrinth; it offers powerful tools and features, yet it can intimidate even the most seasoned users. Understanding the common challenges that practitioners face, as well as their viable solutions, is essential for maximizing the software's potential. Reliability of analysis is fundamentally tied to how we manage data integrity and how we troubleshoot errors that inevitably arise during statistical analysis. This section sheds light on two critical areas: Data Integrity Issues and Troubleshooting Errors, offering insights on maintaining accuracy and ensuring a smoother analytical experience.
Data Integrity Issues
Data integrity stands at the core of any statistical analysis conducted with SPSS. Essentially, it refers to the accuracy and consistency of the data over its lifecycle. If the data is flawed, mischaracterized, or improperly formatted, it will impact the outcome of analyses and can lead to erroneous conclusions. Here are a few common integrity issues:
- Incorrect Data Entry: A simple typographical error can distort entire datasets. Reviewing data for accuracy is paramount.
- Missing Values: When participants skip questions, it can create gaps in data. Strategies for addressing missing data, such as imputation techniques, need to be recognized and applied.
- Outliers: Outliers can skew results significantly. Identifying them is essential as they can either be genuine observations or errors in data collection.
To mitigate these issues, consider implementing validation checks both pre- and post-data entry. This includes utilizing custom scripts within SPSS to flag discrepancies or outliers. Furthermore, establishing strong protocols for data management ensures integrity throughout the analysis process.
Troubleshooting Errors
No matter how adept one is with SPSS, errors will arise. Whether it’s an unexpected output or an error message flashing on the screen, knowing how to troubleshoot effectively is invaluable. Some common issues encountered include:
- Syntax Errors: When running analyses, failing to follow the correct syntax can halt progress. A careful review of the commands used can address this, as many errors simply stem from typographical mistakes, like a missing comma or typo in variable names.
- Software Crashes: System crashes can be disheartening, especially after long hours of work. Ensuring compatibility with system requirements and saving work regularly helps mitigate this risk.
- Incompatibility with Data Formats: Some users may encounter formats that SPSS struggles with, leading to import errors. Ensuring that data is in the correct format, such as .sav or .csv, is crucial. Additionally, SPSS often requires data to be organized in a specific structural manner, otherwise it may reject the data.
Addressing troubleshooting effectively often requires a calm approach. Familiarity with the SPSS error messages can aid in identifying the root cause, and many forums, such as Reddit or SPSS user communities, can offer valuable insights.
Advanced SPSS Capabilities
SPSS is not just a straightforward statistical tool; it boasts advanced capabilities that enhance its utility beyond basic functions. These features are particularly relevant for professionals who seek to maximize their data analysis processes.
Advanced SPSS capabilities allow users to tailor their data analysis approach, facilitating deeper insights and more robust statistical evaluations. This section delves into two critical elements: Custom Macro Development and Integration with Other Software. Both of these features significantly bolster SPSS's adaptability and effectiveness in various applications.
Custom Macro Development
Custom macros in SPSS are like personal assistants that take care of repetitive tasks for you. This powerful tool can save time and enhance productivity by automating routine procedures.
Macros can streamline complex analyses, allowing users to record a sequence of commands and replay them with a single click. This is particularly beneficial for users routinely handling large datasets or applying similar statistical techniques across different projects. The beauty of macro development lies in its flexibility. Users can create a macro that fits their specific needs, incorporating unique parameters and functions that align with their analytical goals.
For instance, someone dealing with multiple survey datasets could develop a macro that standardizes responses, calculates average scores, and outputs the results into a predefined format. By doing so, they can focus on interpreting data rather than getting bogged down in the calculation process.
"Automating repetitive tasks in SPSS through custom macros not only enhances efficiency but also ensures consistency in analyses."
To develop a macro, users simply need to begin recording while performing the desired task. Upon stopping the recording, the user can edit the macro code to refine it further. Knowledge of SPSS syntax becomes handy here, as it can create robust macros that can handle various scenarios.
Integration with Other Software
Today's data landscape is interconnected. Thus, integrating SPSS with other software is crucial for comprehensive analysis.
SPSS's ability to work seamlessly with different data sources, such as Microsoft Excel, R, or even SQL databases, enhances its functionality tremendously. This interoperability allows users to import and export data effortlessly, ensuring that information flows smoothly between platforms without loss of integrity.
For businesses that derive their data from various systems, this integration capability is invaluable. For instance, a company might gather customer feedback through an online survey tool and store that data in a database. By integrating SPSS with the database, analysts can directly pull the data for analysis without manual entry, which reduces the chances of errors and saves time.
Moreover, advanced users may want to integrate SPSS with programming languages such as Python or R to extend SPSS's capabilities even further. This can allow for more sophisticated analysis through advanced algorithms or machine learning models that go beyond SPSS's inherent functionalities.
In summary, the advanced capabilities of SPSS, especially in custom macro development and software integration, are crucial for modern analytical demands. By leveraging these features, users can not only improve their efficiency but also enhance the depth and quality of their statistical analyses.
Educational Resources for SPSS
In the realm of statistical analysis, where SPSS reigns as an essential tool, the significance of accessible educational resources cannot be overstated. Educational tools empower users at various experience levels, from novice analysts to seasoned researchers, helping them navigate and utilize the software effectively. Understanding how to harness the full capabilities of SPSS allows users to enhance their analytical skills, leading to more informed decisions based on robust data interpretations. Moreover, proficiency in SPSS can offer a competitive edge in today's data-driven job market, where analytics skills are increasingly sought after.
Online Tutorials and Courses
With a plethora of online tutorials and courses available, individuals seeking to enhance their SPSS knowledge are spoilt for choice. These resources cater to specific learning styles and paces, making them an appealing option for many.
- Structured Learning: Many platforms provide comprehensive courses that lead learners from the basics to advanced analytics techniques. This step-by-step approach can be invaluable for beginners who may feel overwhelmed by SPSS’s rich features.
- Interactive Elements: Online courses often incorporate quizzes and hands-on projects, providing a practical understanding of concepts. This active engagement aids retention and application of knowledge.
- Flexible Scheduling: The flexibility of online learning allows individuals to study at their convenience. Whether it’s short tutorials on YouTube or more extended courses on platforms like Coursera or Udemy, learners can fit education into their busy schedules.
Some notable platforms worth exploring include:
- Coursera – Often collaborates with universities to offer credible courses.
- LinkedIn Learning – Provides courses tailored for professionals looking to enhance their skills.
- YouTube – A great place for free tutorials across various topics, including SPSS.
Engaging with these online courses not only enhances understanding but also fosters confidence in using SPSS for real-world applications.
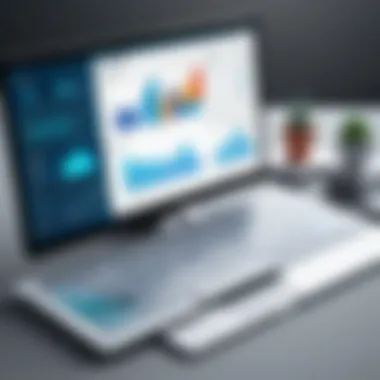
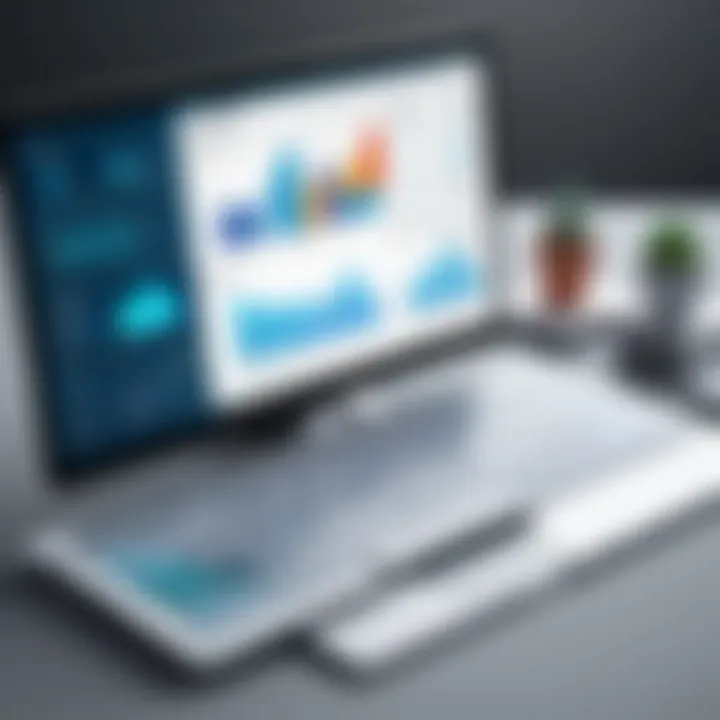
Books and Publications
For those who prefer a more traditional approach to learning, books and publications specializing in SPSS can be a treasure trove of knowledge. These texts often dive deep into theoretical foundations, practical applications, and case studies that illustrate real-world usage.
- Comprehensive Guides: Books like "SPSS for Dummies" or "Discovering Statistics Using IBM SPSS Statistics" provide structured content that spans the software's functions. These guides often have exercises and examples that reinforce learning.
- Research Publications: Journals and articles in statistical research often showcase the use of SPSS in various studies, giving readers insight into its application in the field.
- Step-by-Step Manuals: Many publications offer detailed, step-by-step instructions for complex analyses, which can be particularly useful for users faced with specific statistical problems.
"Learning from books allows for in-depth exploration of SPSS, offering a breadth of information that complements online interactive tools."
Comparing SPSS with Other Statistical Tools
In the realm of data analysis, choosing the right statistical tool is akin to selecting the best knife for the job. SPSS stands out as a prominent player in this field, yet it's crucial to compare it with other tools like R Programming and SAS to fully understand its strengths and weaknesses.
Assessing SPSS against its counterparts not only offers insights into its capabilities but also informs choices for various analytical needs. Any analyst worth their salt knows that no single tool fits all scenarios. This comparison highlights specific elements such as usability, flexibility, and the diverse applications inherent to each tool. By understanding these differences, professionals can streamline their data analysis processes, ensuring efficiency and accuracy in their results.
SPSS vs. R Programming
When contrasting SPSS with R Programming, a couple of stark differences come to light. At first glance, SPSS provides a user-friendly interface. This lets analysts grasp the basics rapidly, making it ideal for those who may not have a strong background in programming. Conversely, R is a programming language that demands a degree of coding knowledge. This can create a steep learning curve for those unfamiliar with the intricacies of coding.
However, don’t let that simplicity deceive you. R can be exceptionally powerful. Its capabilities extend beyond basic statistical analyses into advanced modeling and visualizations. Users can customize their analyses with packages, making R immensely versatile. Each package built tends to cater to specialized needs, where SPSS may not always offer direct solutions.
Another notable consideration is cost. SPSS demands a financial investment. In contrast, R is open-source, which means it’s free to use. This can be a game-changer, especially for small businesses or academic institutions with limited budgets.
While SPSS may excel in ease of use, R’s vast resource pool and flexibility position it as a robust alternative for in-depth statistical tasks.
SPSS vs. SAS
Now, let’s turn to SAS, another titan in the statistical computing arena. While SPSS caters largely to social sciences and similar fields, SAS is widely embraced in industries like healthcare and finance, where heavy lifting in analytics is commonplace. This specificity often dictates preference based on the field of work.
In terms of user experience, SAS also has a steep learning curve, albeit not as daunting as R’s. Over the years, SAS has built a reputation among professionals for its reliability and credibility in enterprise environments. SPSS, with its graphical interface, might appeal to users seeking quick access to functionalities without diving deep into syntax.
Yet, both SPSS and SAS deliver sophisticated statistical analysis capabilities. SAS shines with its data processing efficiency, especially with larger datasets. If an analyst is dealing with big data, the performance metrics here tilt the scales in SAS's favor.
"When analyzing large-scale datasets, familiarity with SAS's optimization strategies can save countless hours."
Additionally, cost again plays a role. SAS licenses can be on the pricey side, similar to SPSS, which brings us back to the question of cost-effectiveness. In essence, the choice may boil down to specific use cases, operational needs, and budget constraints.
The End
Comparing SPSS with R Programming and SAS reveals a spectrum of capabilities and suitability for various tasks. R holds distinct advantages in flexibility and cost-effectiveness, while SAS offers robust processing power for larger data volumes. SPSS finds its niche in user-friendliness and accessibility. Every professional has different needs, and understanding these subtleties is key to choosing the right tool for data analysis.
The Future of SPSS
As the landscape of data analysis continues to evolve, understanding the future of SPSS becomes crucial for any professional navigating the intricate world of statistics. This section focuses on potential trajectories that are likely to shape this software's development alongside modern analytical practices. The evolution of SPSS not only reflects advancements in technology but also addresses user needs in various fields, including academia, business, and healthcare. The crux of the future lies in its adaptability and integration within new environments and methodologies.
Emerging Trends in Data Analysis
Data analysis is in a state of rapid progression, driven by advances in artificial intelligence, machine learning, and a growing emphasis on real-time data processing. As SPSS rides along these waves of innovation, several trends are noteworthy:
- AI and Machine Learning Integration: The amalgamation of SPSS with machine learning frameworks allows users to harness predictive analytics more effectively. This integration enhances the potential for more complex models that can adapt over time, yielding insights previously thought out of reach for standard statistical software.
- Big Data Compatibility: With the ever-increasing data pool from various sources, SPSS is evolving to handle big data analytics. The ability to merge and analyze large datasets efficiently will not only enhance its utility but also ensure relevancy in an increasingly data-driven society.
- User-Centric Interfaces: As the user community expands, so does the demand for intuitive interfaces. Future iterations of SPSS are likely to feature more guided analytics capabilities that help users—regardless of their statistical background—navigate through data sets and extract actionable insights with greater ease.
"The future of data analysis is not just about number crunching; it's about storytelling through data that resonates with the audience's understanding."
By considering these trends, SPSS can position itself as an indispensable tool in the modern data analytics toolkit.
SPSS in a Cloud-Based Environment
The transition to cloud-based environments signifies a major leap forward for data analysis tools, including SPSS. Moving SPSS functionalities to the cloud offers a slew of benefits:
- Accessibility: Users can access data and analytics tools from anywhere, breaking down the strict geographical barriers that traditional software imposed. This convenience is particularly impactful for teams that operate remotely or across multiple locations.
- Scalability: Cloud solutions inherently offer scalable options. As data needs change for organizations, SPSS can seamlessly accommodate an increase in capacity without requiring significant hardware investments or complex installations.
- Collaborative Features: Operating in a cloud environment enhances collaboration among users. Multiple stakeholders can work on the same dataset, allowing for real-time updates and shared insights, leading to more informed decision-making.
The shift to the cloud is not necessarily without its challenges—data security and privacy concerns are paramount. However, SPSS's commitment to user security, paired with enhanced capabilities in cloud environments, presents a compelling case for its sustained use in the future.
In summary, the future of SPSS is promising, with exciting developments on the horizon that align with broader trends in data analysis and technological innovation. Incorporating these trends enables both SPSS and its users to stay ahead of the curve, ensuring that the software remains a relevant player in the ever-expanding field of analytics.
Ethical Considerations in Statistical Analysis
When diving into the realm of data analysis, a comprehensive approach must also consider the ethical aspects that underpin statistical practices. The importance of ethical considerations in statistical analysis cannot be overstated. As data becomes increasingly integral to decision-making processes across various sectors, the responsibility of analysts grows. Engaging in ethical statistical practices not only enhances the credibility of analyses but also ensures that the rights and privacy of individuals are respected.
Data Privacy Issues
Data privacy is one of the keystones of ethical analysis. With data breaches making headlines, understanding the implications of data privacy becomes paramount. Analysts often deal with sensitive information, whether it's personal health records in healthcare analytics or customer data in business environments. Thus, they must adhere to stringent guidelines and regulations such as GDPR or HIPAA to protect this information.
Implications of Ignoring Privacy:
- Legal Repercussions: Violating data privacy laws can lead to hefty fines and legal action against individuals or organizations.
- Loss of Trust: Once trust is broken, it's difficult, sometimes impossible, to restore. End-users may be hesitant to share data in the future.
- Data Integrity Issues: Mishandling data can fundamentally skew results, leading to incorrect conclusions that, by extension, could misinform policy-making or business strategies.
Data privacy isn't just a legal requirement; it's a moral obligation. Protecting individuals' information should always be a top priority.
Transparency in Reporting
Another vital aspect of ethical considerations revolves around transparency in reporting statistical findings. Analysts have a duty to present their results honestly, without cherry-picking data or selectively reporting metrics to fit a narrative.
Benefits of Transparency:
- Credibility: When the methodology and data sources are clear, findings gain legitimacy. Readers can trust that results have been derived from sound analyses.
- Facilitates Peer Review: Transparent reporting allows others in the field to verify and replicate studies, reinforcing scientific rigor.
- Promotes Accountability: Analysts who are transparent in their work are less likely to engage in practices like data fabrication. They also create a culture of ethical rigor within their teams.
Finale
In this concluding section, it is vital to underscore the significance of SPSS within the realms of data analysis and statistical research. As we've delved into earlier, SPSS stands as a fundamental tool, cherished by many professionals who navigate the complexities of statistical evaluation. Its extensive array of features makes it not just a software choice but a powerful ally in deciphering data trends and informing critical decisions across various sectors.
Summarizing Key Insights
Throughout the article, we dissected numerous aspects of SPSS, revealing its layered functionalities and applications. Here are some key insights:
- User-Friendly Interface: SPSS's intuitive design enables users, even those with limited statistical know-how, to maneuver through data management and analysis tasks.
- Robust Analytical Tools: From descriptive to inferential statistics, SPSS provides an extensive suite of tools that cater to diverse analytical needs. This makes the software versatile and comprehensive.
- Data Visualization: The capability of SPSS to graphically represent data allows practitioners to convey complex information in a digestible format — an essential skill in any analytical profession.
- Diverse Applications: Whether in academic research or healthcare analytics, SPSS serves as a reliable platform for drawing actionable insights from data.
This compilation illustrates how SPSS not only facilitates statistical analysis but also supports informed decision-making across various industries. Understanding these insights is crucial for leveraging SPSS’s capabilities effectively.
Final Thoughts on SPSS
As we wrap up our exploration of SPSS, it's apparent that this statistical software is more than a mere tool; it's a gateway to unlocking insightful narratives hidden within data. The benefits derived from adeptly utilizing SPSS can propel organizations toward greater efficiency and success in their operations.
One can't ignore the implications of becoming proficient in SPSS. As businesses lean more toward data-driven insights, mastering this software becomes essential. The knowledge gained from using SPSS often translates into tangible business improvements, whether through enhanced customer understanding, improved operational efficiencies, or reduced risk.
"Data is the new oil; with SPSS, you have the drill and the refinery to extract valuable insights."